SCIENCE
Publications
2024
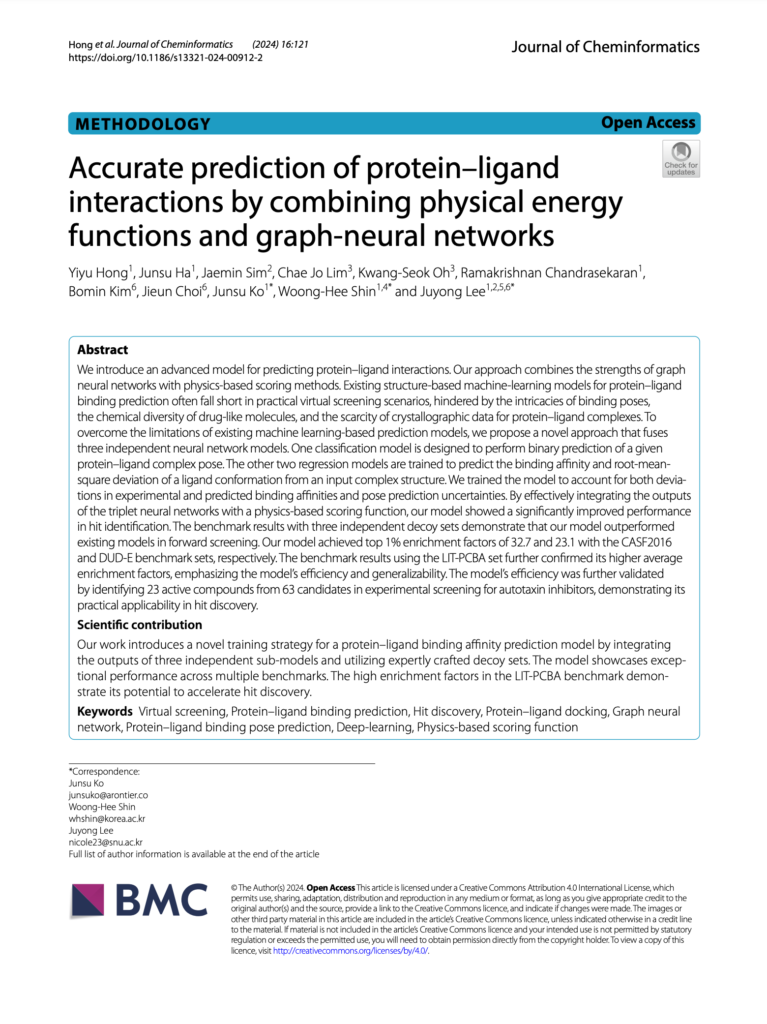
Hong Y; Ha J; Sim J; Lim C J; Oh K; Chandrasekaran R; Kim B; Choi J; Ko J; Shin W; Lee J
Accurate prediction of protein–ligand interactions by combining physical energy functions and graph-neural networks Journal Article
In: J Cheminform, vol. 16, no. 1, 2024, ISSN: 1758-2946.
@article{Hong2024,
title = {Accurate prediction of protein–ligand interactions by combining physical energy functions and graph-neural networks},
author = {Yiyu Hong and Junsu Ha and Jaemin Sim and Chae Jo Lim and Kwang-Seok Oh and Ramakrishnan Chandrasekaran and Bomin Kim and Jieun Choi and Junsu Ko and Woong-Hee Shin and Juyong Lee},
doi = {10.1186/s13321-024-00912-2},
issn = {1758-2946},
year = {2024},
date = {2024-11-04},
urldate = {2024-11-04},
journal = {J Cheminform},
volume = {16},
number = {1},
publisher = {Springer Science and Business Media LLC},
abstract = {We introduce an advanced model for predicting protein–ligand interactions. Our approach combines the strengths of graph neural networks with physics-based scoring methods. Existing structure-based machine-learning models for protein–ligand binding prediction often fall short in practical virtual screening scenarios, hindered by the intricacies of binding poses, the chemical diversity of drug-like molecules, and the scarcity of crystallographic data for protein–ligand complexes. To overcome the limitations of existing machine learning-based prediction models, we propose a novel approach that fuses three independent neural network models. One classification model is designed to perform binary prediction of a given protein–ligand complex pose. The other two regression models are trained to predict the binding affinity and root-mean-square deviation of a ligand conformation from an input complex structure. We trained the model to account for both deviations in experimental and predicted binding affinities and pose prediction uncertainties. By effectively integrating the outputs of the triplet neural networks with a physics-based scoring function, our model showed a significantly improved performance in hit identification. The benchmark results with three independent decoy sets demonstrate that our model outperformed existing models in forward screening. Our model achieved top 1% enrichment factors of 32.7 and 23.1 with the CASF2016 and DUD-E benchmark sets, respectively. The benchmark results using the LIT-PCBA set further confirmed its higher average enrichment factors, emphasizing the model’s efficiency and generalizability. The model’s efficiency was further validated by identifying 23 active compounds from 63 candidates in experimental screening for autotaxin inhibitors, demonstrating its practical applicability in hit discovery.
Scientific contribution Our work introduces a novel training strategy for a protein–ligand binding affinity prediction model by integrating the outputs of three independent sub-models and utilizing expertly crafted decoy sets. The model showcases exceptional performance across multiple benchmarks. The high enrichment factors in the LIT-PCBA benchmark demonstrate its potential to accelerate hit discovery.},
keywords = {},
pubstate = {published},
tppubtype = {article}
}
Scientific contribution Our work introduces a novel training strategy for a protein–ligand binding affinity prediction model by integrating the outputs of three independent sub-models and utilizing expertly crafted decoy sets. The model showcases exceptional performance across multiple benchmarks. The high enrichment factors in the LIT-PCBA benchmark demonstrate its potential to accelerate hit discovery.
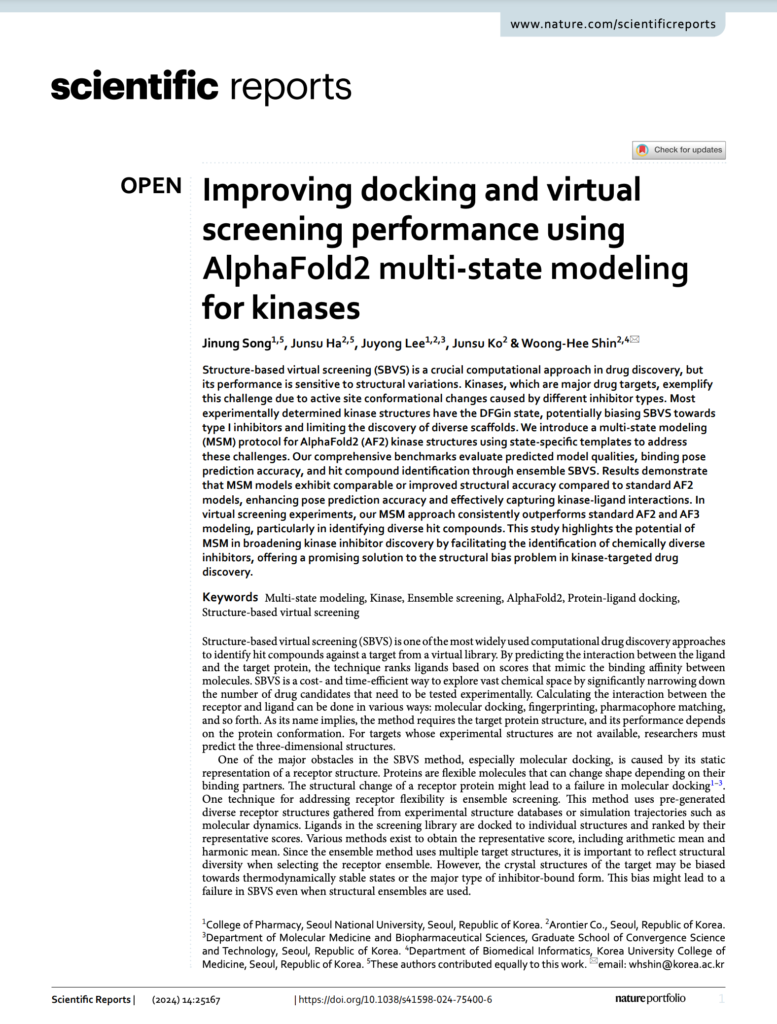
Song J; Ha J; Lee J; Ko J; Shin W
Improving docking and virtual screening performance using AlphaFold2 multi-state modeling for kinases Journal Article
In: Sci Rep, vol. 14, no. 1, 2024, ISSN: 2045-2322.
@article{Song2024b,
title = {Improving docking and virtual screening performance using AlphaFold2 multi-state modeling for kinases},
author = {Jinung Song and Junsu Ha and Juyong Lee and Junsu Ko and Woong-Hee Shin},
doi = {10.1038/s41598-024-75400-6},
issn = {2045-2322},
year = {2024},
date = {2024-10-24},
urldate = {2024-10-24},
journal = {Sci Rep},
volume = {14},
number = {1},
publisher = {Springer Science and Business Media LLC},
abstract = {Structure-based virtual screening (SBVS) is a crucial computational approach in drug discovery, but its performance is sensitive to structural variations. Kinases, which are major drug targets, exemplify this challenge due to active site conformational changes caused by different inhibitor types. Most experimentally determined kinase structures have the DFGin state, potentially biasing SBVS towards type I inhibitors and limiting the discovery of diverse scaffolds. We introduce a multi-state modeling (MSM) protocol for AlphaFold2 (AF2) kinase structures using state-specific templates to address these challenges. Our comprehensive benchmarks evaluate predicted model qualities, binding pose prediction accuracy, and hit compound identification through ensemble SBVS. Results demonstrate that MSM models exhibit comparable or improved structural accuracy compared to standard AF2 models, enhancing pose prediction accuracy and effectively capturing kinase-ligand interactions. In virtual screening experiments, our MSM approach consistently outperforms standard AF2 and AF3 modeling, particularly in identifying diverse hit compounds. This study highlights the potential of MSM in broadening kinase inhibitor discovery by facilitating the identification of chemically diverse inhibitors, offering a promising solution to the structural bias problem in kinase-targeted drug discovery.},
keywords = {},
pubstate = {published},
tppubtype = {article}
}
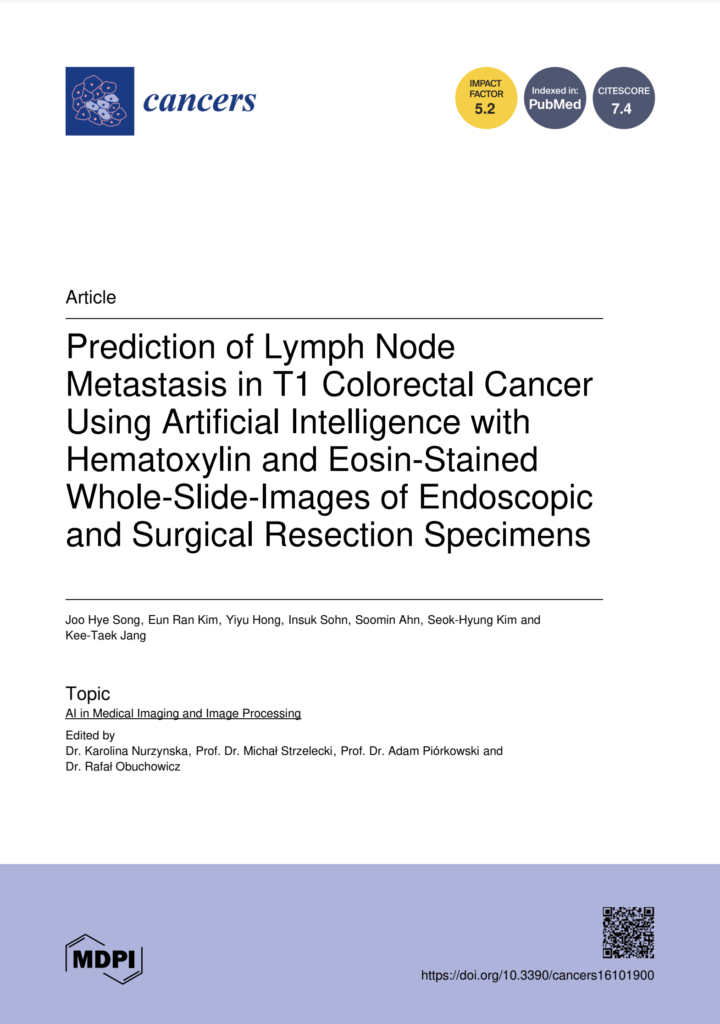
Song J H; Kim E R; Hong Y; Sohn I; Ahn S; Kim S; Jang K
In: Cancers, vol. 16, no. 10, 2024, ISSN: 2072-6694.
@article{Song2024,
title = {Prediction of Lymph Node Metastasis in T1 Colorectal Cancer Using Artificial Intelligence with Hematoxylin and Eosin-Stained Whole-Slide-Images of Endoscopic and Surgical Resection Specimens},
author = {Joo Hye Song and Eun Ran Kim and Yiyu Hong and Insuk Sohn and Soomin Ahn and Seok-Hyung Kim and Kee-Taek Jang},
doi = {10.3390/cancers16101900},
issn = {2072-6694},
year = {2024},
date = {2024-05-16},
urldate = {2024-05-16},
journal = {Cancers},
volume = {16},
number = {10},
publisher = {MDPI AG},
abstract = {According to the current guidelines, additional surgery is performed for endoscopically resected specimens of early colorectal cancer (CRC) with a high risk of lymph node metastasis (LNM). However, the rate of LNM is 2.1–25.0% in cases treated endoscopically followed by surgery, indicating a high rate of unnecessary surgeries. Therefore, this study aimed to develop an artificial intelligence (AI) model using H&E-stained whole slide images (WSIs) without handcrafted features employing surgically and endoscopically resected specimens to predict LNM in T1 CRC. To validate with an independent cohort, we developed a model with four versions comprising various combinations of training and test sets using H&E-stained WSIs from endoscopically (400 patients) and surgically resected specimens (881 patients): Version 1, Train and Test: surgical specimens; Version 2, Train and Test: endoscopic and surgically resected specimens; Version 3, Train: endoscopic and surgical specimens and Test: surgical specimens; Version 4, Train: endoscopic and surgical specimens and Test: endoscopic specimens. The area under the curve (AUC) of the receiver operating characteristic curve was used to determine the accuracy of the AI model for predicting LNM with a 5-fold cross-validation in the training set. Our AI model with H&E-stained WSIs and without annotations showed good performance power with the validation of an independent cohort in a single center. The AUC of our model was 0.758–0.830 in the training set and 0.781–0.824 in the test set, higher than that of previous AI studies with only WSI. Moreover, the AI model with Version 4, which showed the highest sensitivity (92.9%), reduced unnecessary additional surgery by 14.2% more than using the current guidelines (68.3% vs. 82.5%). This revealed the feasibility of using an AI model with only H&E-stained WSIs to predict LNM in T1 CRC.},
keywords = {},
pubstate = {published},
tppubtype = {article}
}
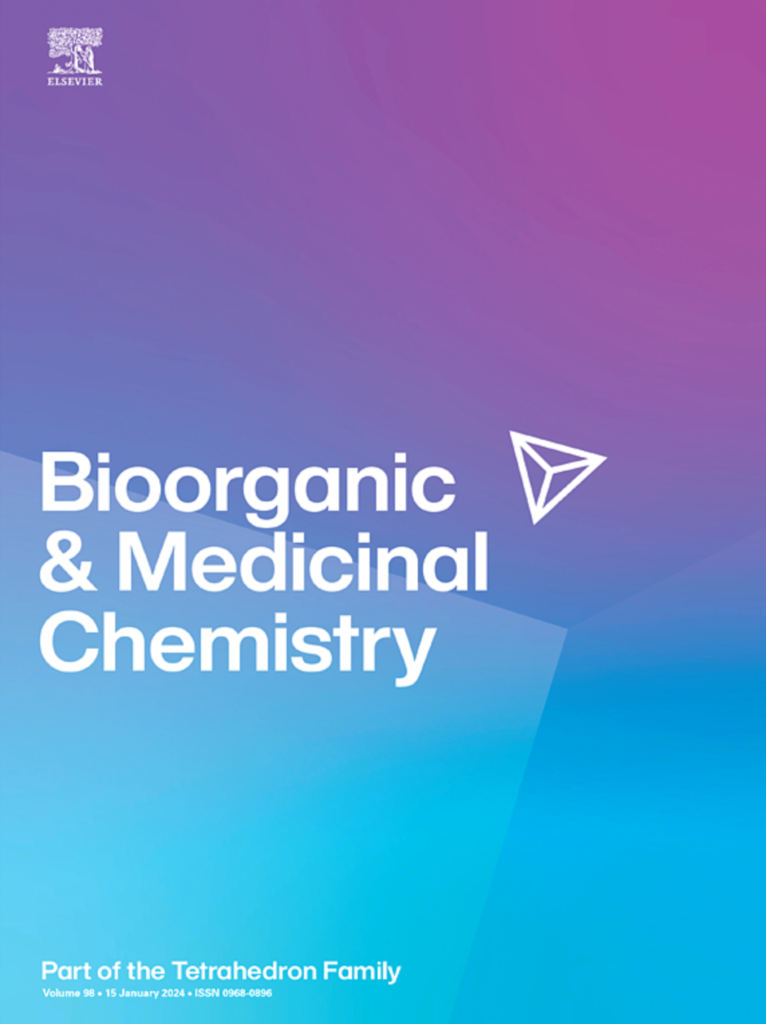
Yang H; Kang M; Jang S; Baek S Y; Kim J; Kim G U; Kim D; Ha J; Kim J S; Jung C; Kim N; Cho S; Shin W; Lee J; Ko J; Lee A; Keum G; Lee S; Kang T
Discovery of thiophen-2-ylmethylene bis-dimedone derivatives as novel WRN inhibitors for treating cancers with microsatellite instability Journal Article
In: Bioorganic & Medicinal Chemistry, vol. 100, 2024, ISSN: 0968-0896.
@article{Yang2024,
title = {Discovery of thiophen-2-ylmethylene bis-dimedone derivatives as novel WRN inhibitors for treating cancers with microsatellite instability},
author = {Hwasun Yang and Miso Kang and Seonyeong Jang and Soo Yeon Baek and Jiwon Kim and Gyeong Un Kim and Dongwoo Kim and Junsu Ha and Jong Seung Kim and Cheulhee Jung and Nam-Jung Kim and Sung-Yup Cho and Woong-Hee Shin and Juyong Lee and Junsu Ko and Ansoo Lee and Gyochang Keum and Sanghee Lee and Taek Kang},
doi = {10.1016/j.bmc.2024.117588},
issn = {0968-0896},
year = {2024},
date = {2024-02-00},
urldate = {2024-02-00},
journal = {Bioorganic & Medicinal Chemistry},
volume = {100},
publisher = {Elsevier BV},
abstract = {Microsatellite instability (MSI) is a hypermutable condition caused by DNA mismatch repair system defects, contributing to the development of various cancer types. Recent research has identified Werner syndrome ATP-dependent helicase (WRN) as a promising synthetic lethal target for MSI cancers. Herein, we report the first discovery of thiophen-2-ylmethylene bis-dimedone derivatives as novel WRN inhibitors for MSI cancer therapy. Initial computational analysis and biological evaluation identified a new scaffold for a WRN inhibitor. Subsequent SAR study led to the discovery of a highly potent WRN inhibitor. Furthermore, we demonstrated that the optimal compound induced DNA damage and apoptotic cell death in MSI cancer cells by inhibiting WRN. This study provides a new pharmacophore for WRN inhibitors, emphasizing their therapeutic potential for MSI cancers.},
keywords = {},
pubstate = {published},
tppubtype = {article}
}
2023
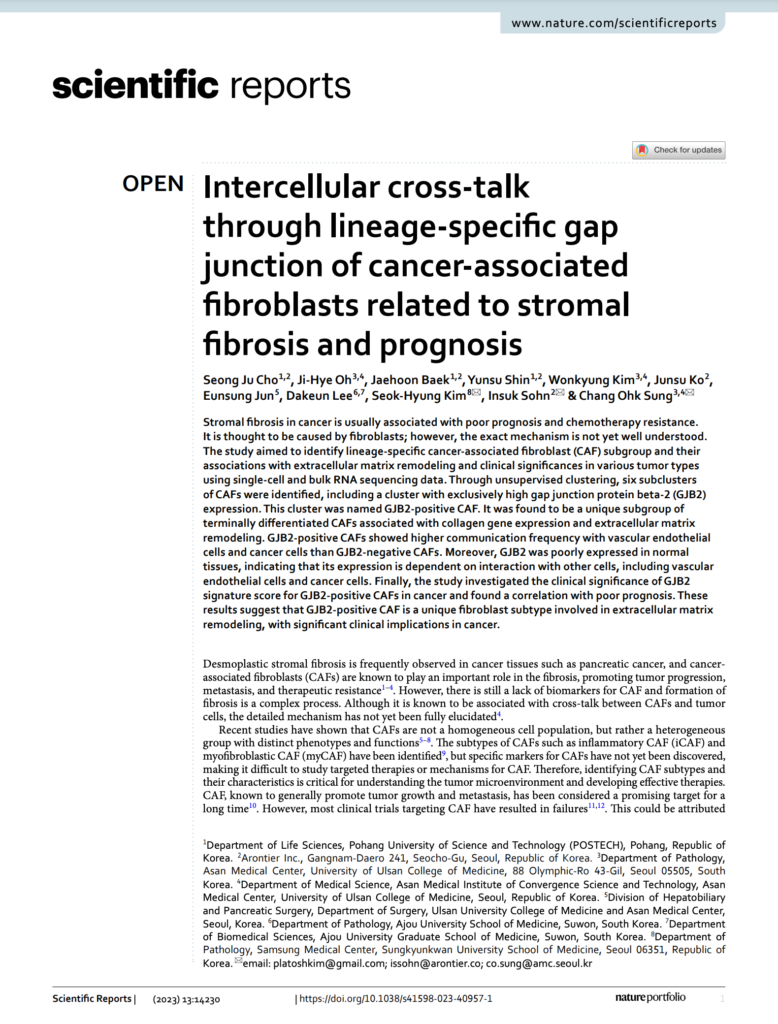
Cho S J; Oh J; Baek J; Shin Y; Kim W; Ko J; Jun E; Lee D; Kim S; Sohn I; Sung C O
Intercellular cross-talk through lineage-specific gap junction of cancer-associated fibroblasts related to stromal fibrosis and prognosis Journal Article
In: Sci Rep, vol. 13, no. 1, 2023, ISSN: 2045-2322.
@article{Cho2023,
title = {Intercellular cross-talk through lineage-specific gap junction of cancer-associated fibroblasts related to stromal fibrosis and prognosis},
author = {Seong Ju Cho and Ji-Hye Oh and Jaehoon Baek and Yunsu Shin and Wonkyung Kim and Junsu Ko and Eunsung Jun and Dakeun Lee and Seok-Hyung Kim and Insuk Sohn and Chang Ohk Sung},
doi = {10.1038/s41598-023-40957-1},
issn = {2045-2322},
year = {2023},
date = {2023-12-00},
urldate = {2023-12-00},
journal = {Sci Rep},
volume = {13},
number = {1},
publisher = {Springer Science and Business Media LLC},
abstract = {Stromal fibrosis in cancer is usually associated with poor prognosis and chemotherapy resistance. It is thought to be caused by fibroblasts; however, the exact mechanism is not yet well understood. The study aimed to identify lineage-specific cancer-associated fibroblast (CAF) subgroup and their associations with extracellular matrix remodeling and clinical significances in various tumor types using single-cell and bulk RNA sequencing data. Through unsupervised clustering, six subclusters of CAFs were identified, including a cluster with exclusively high gap junction protein beta-2 (GJB2) expression. This cluster was named GJB2-positive CAF. It was found to be a unique subgroup of terminally differentiated CAFs associated with collagen gene expression and extracellular matrix remodeling. GJB2-positive CAFs showed higher communication frequency with vascular endothelial cells and cancer cells than GJB2-negative CAFs. Moreover, GJB2 was poorly expressed in normal tissues, indicating that its expression is dependent on interaction with other cells, including vascular endothelial cells and cancer cells. Finally, the study investigated the clinical significance of GJB2 signature score for GJB2-positive CAFs in cancer and found a correlation with poor prognosis. These results suggest that GJB2-positive CAF is a unique fibroblast subtype involved in extracellular matrix remodeling, with significant clinical implications in cancer.},
keywords = {},
pubstate = {published},
tppubtype = {article}
}
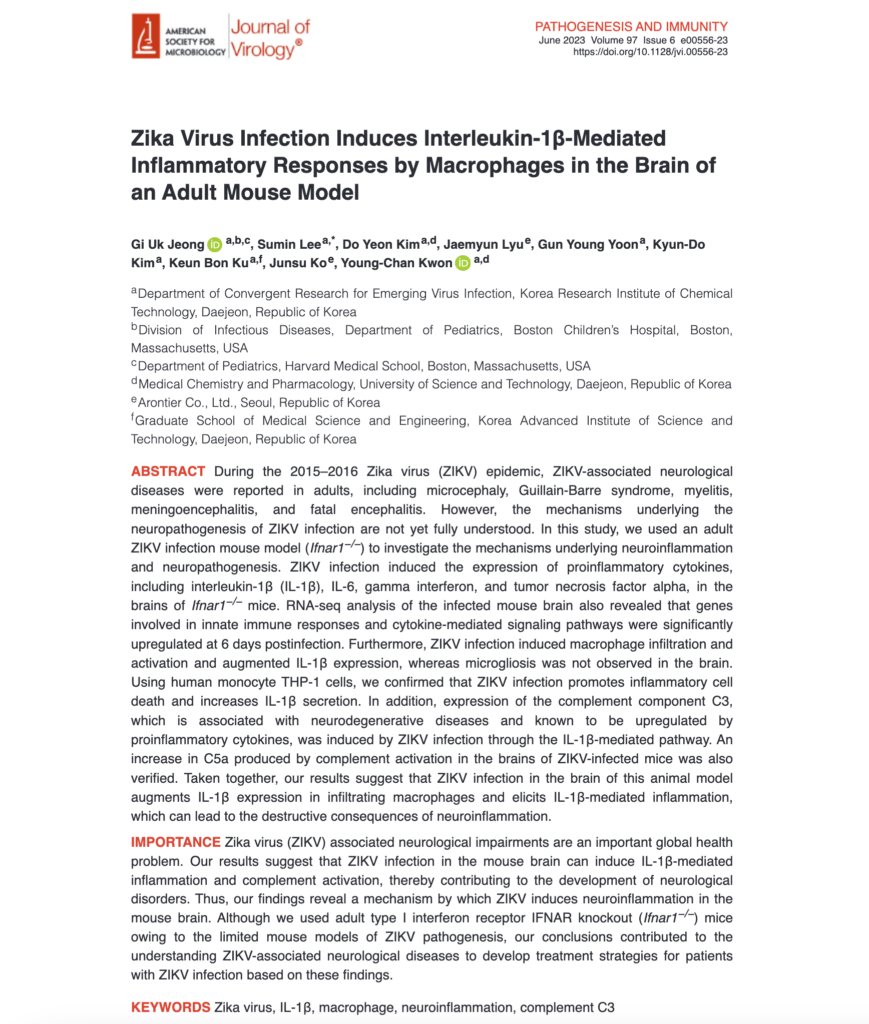
Jeong G U; Lee S; Kim D Y; Lyu J; Yoon G Y; Kim K; Ku K B; Ko J; Kwon Y
Zika Virus Infection Induces Interleukin-1β-Mediated Inflammatory Responses by Macrophages in the Brain of an Adult Mouse Model Journal Article
In: J Virol, vol. 97, no. 6, 2023, ISSN: 1098-5514.
@article{Jeong2023,
title = {Zika Virus Infection Induces Interleukin-1β-Mediated Inflammatory Responses by Macrophages in the Brain of an Adult Mouse Model},
author = {Gi Uk Jeong and Sumin Lee and Do Yeon Kim and Jaemyun Lyu and Gun Young Yoon and Kyun-Do Kim and Keun Bon Ku and Junsu Ko and Young-Chan Kwon},
editor = {Felicia Goodrum},
doi = {10.1128/jvi.00556-23},
issn = {1098-5514},
year = {2023},
date = {2023-06-29},
urldate = {2023-06-29},
journal = {J Virol},
volume = {97},
number = {6},
publisher = {American Society for Microbiology},
abstract = {During the 2015–2016 Zika virus (ZIKV) epidemic, ZIKV-associated neurological diseases were reported in adults, including microcephaly, Guillain-Barre syndrome, myelitis, meningoencephalitis, and fatal encephalitis. However, the mechanisms underlying the neuropathogenesis of ZIKV infection are not yet fully understood. In this study, we used an adult ZIKV infection mouse model (Ifnar1−/−) to investigate the mechanisms underlying neuroinflammation and neuropathogenesis. ZIKV infection induced the expression of proinflammatory cytokines, including interleukin-1β (IL-1β), IL-6, gamma interferon, and tumor necrosis factor alpha, in the brains of Ifnar1−/− mice. RNA-seq analysis of the infected mouse brain also revealed that genes involved in innate immune responses and cytokine-mediated signaling pathways were significantly upregulated at 6 days postinfection. Furthermore, ZIKV infection induced macrophage infiltration and activation and augmented IL-1β expression, whereas microgliosis was not observed in the brain. Using human monocyte THP-1 cells, we confirmed that ZIKV infection promotes inflammatory cell death and increases IL-1β secretion. In addition, expression of the complement component C3, which is associated with neurodegenerative diseases and known to be upregulated by proinflammatory cytokines, was induced by ZIKV infection through the IL-1β-mediated pathway. An increase in C5a produced by complement activation in the brains of ZIKV-infected mice was also verified. Taken together, our results suggest that ZIKV infection in the brain of this animal model augments IL-1β expression in infiltrating macrophages and elicits IL-1β-mediated inflammation, which can lead to the destructive consequences of neuroinflammation.
IMPORTANCE Zika virus (ZIKV) associated neurological impairments are an important global health problem. Our results suggest that ZIKV infection in the mouse brain can induce IL-1β-mediated inflammation and complement activation, thereby contributing to the development of neurological disorders. Thus, our findings reveal a mechanism by which ZIKV induces neuroinflammation in the mouse brain. Although we used adult type I interferon receptor IFNAR knockout (Ifnar1−/−) mice owing to the limited mouse models of ZIKV pathogenesis, our conclusions contributed to the understanding ZIKV-associated neurological diseases to develop treatment strategies for patients with ZIKV infection based on these findings.},
keywords = {},
pubstate = {published},
tppubtype = {article}
}
IMPORTANCE Zika virus (ZIKV) associated neurological impairments are an important global health problem. Our results suggest that ZIKV infection in the mouse brain can induce IL-1β-mediated inflammation and complement activation, thereby contributing to the development of neurological disorders. Thus, our findings reveal a mechanism by which ZIKV induces neuroinflammation in the mouse brain. Although we used adult type I interferon receptor IFNAR knockout (Ifnar1−/−) mice owing to the limited mouse models of ZIKV pathogenesis, our conclusions contributed to the understanding ZIKV-associated neurological diseases to develop treatment strategies for patients with ZIKV infection based on these findings.
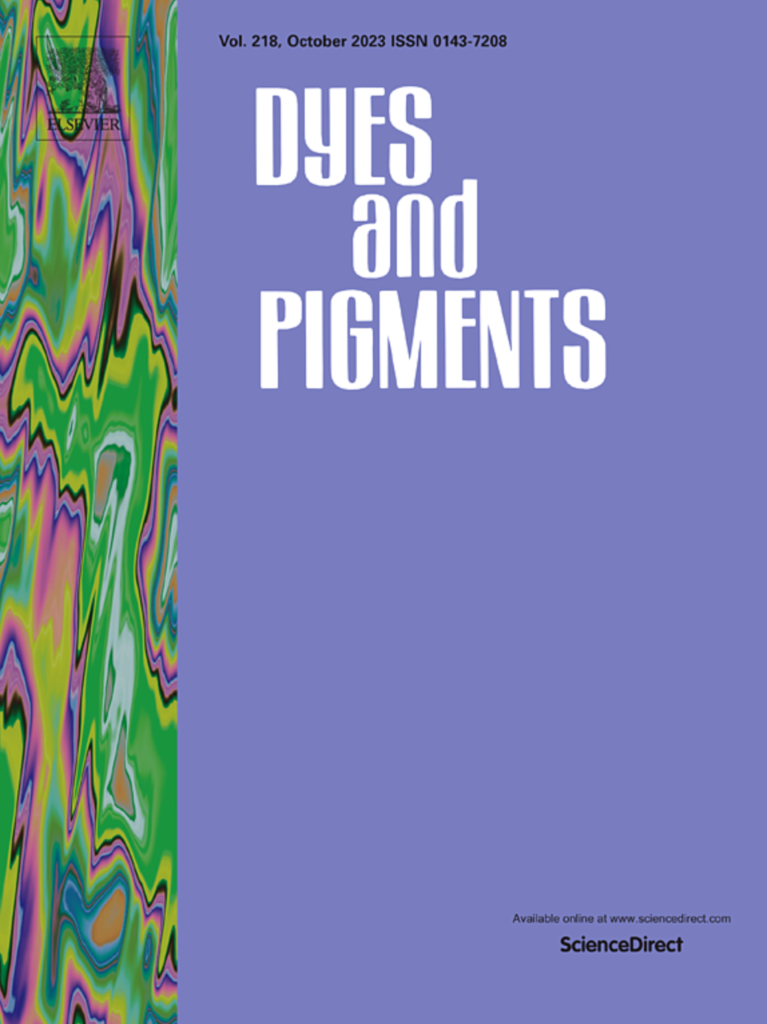
Lee J; Choi S; Aslam A S; Lim W; Lee J; Ko J; Ryu C H; Lee K M; Jung Y M; Yoo H S; Park J H; Lee S; Choi J; Kim E; Park J
N4-phenylquinazoline-4,6-diamine as a tunable fluorescent scaffold for the development of fluorescent probes Journal Article
In: Dyes and Pigments, vol. 210, 2023, ISSN: 0143-7208.
@article{Lee2023,
title = {N4-phenylquinazoline-4,6-diamine as a tunable fluorescent scaffold for the development of fluorescent probes},
author = {Jaeseok Lee and Sang-Kee Choi and Adil S. Aslam and Woojeong Lim and Juyong Lee and Junsu Ko and Chan Hee Ryu and Kang Mun Lee and Young Mee Jung and Hyuk Sang Yoo and Ju Hyun Park and Sanghee Lee and Junwon Choi and Eunha Kim and Jongmin Park},
doi = {10.1016/j.dyepig.2022.110987},
issn = {0143-7208},
year = {2023},
date = {2023-02-00},
urldate = {2023-02-00},
journal = {Dyes and Pigments},
volume = {210},
publisher = {Elsevier BV},
abstract = {Fluorescent molecules have been used for various applications in biological system monitoring. Considering the complexity of cellular systems, there is a huge demand for diverse fluorescent organic molecular scaffolds. However, only a limited number of fluorescent scaffolds have been reported due to the lack of a rational design strategy. We serendipitously discovered N4-phenylquinazoline-4,6-diamine as a fluorescent scaffold with turn-on characteristics. To improve the photophysical property of the initial fluorescence molecule, we synthesized derivatives of the N4-phenylquinazoline-4,6-diamine and found systematic correlation between electronic density of the phenyl substituent of the scaffold and fluorescence intensity. Through tuning the photophysical property of the scaffold and a rational design strategy, we developed N4-dichlorophenylquinazoline-4,6-diamine as a potential fluorophore for various biological applications. To prove the value of the developed fluorescent scaffold, we devised formaldehyde (FA) fluorescent sensor by tailoring amine on 6-position of N4-dichlorophenylquinazoline-4,6-diamine to employ a 2-aza-Cope rearrangement as the molecular detection mechanism with double-digit nanomolar detection limits. FA level in live HeLa cells were successfully visualized with the probe, exhibiting that N4-dichlorophenylquinazoline-4,6-diamine can serve as a useful molecular scaffold for offering various fluorescent sensors toward the investigation of physiological and pathological processes in live cells.},
keywords = {},
pubstate = {published},
tppubtype = {article}
}
2022
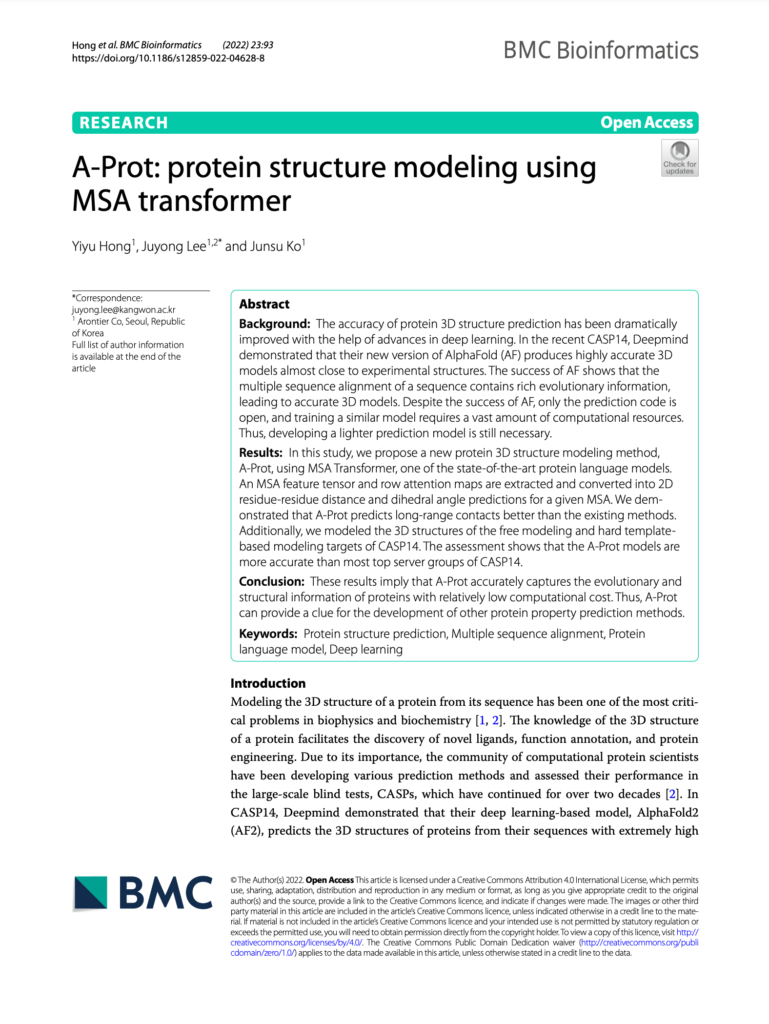
Hong Y; Lee J; Ko J
A-Prot: protein structure modeling using MSA transformer Journal Article
In: BMC Bioinformatics, vol. 23, no. 1, 2022, ISSN: 1471-2105.
@article{Hong2022,
title = {A-Prot: protein structure modeling using MSA transformer},
author = {Yiyu Hong and Juyong Lee and Junsu Ko},
doi = {10.1186/s12859-022-04628-8},
issn = {1471-2105},
year = {2022},
date = {2022-12-00},
urldate = {2022-12-00},
journal = {BMC Bioinformatics},
volume = {23},
number = {1},
publisher = {Springer Science and Business Media LLC},
abstract = {Background: The accuracy of protein 3D structure prediction has been dramatically improved with the help of advances in deep learning. In the recent CASP14, Deepmind demonstrated that their new version of AlphaFold (AF) produces highly accurate 3D models almost close to experimental structures. The success of AF shows that the multiple sequence alignment of a sequence contains rich evolutionary information, leading to accurate 3D models. Despite the success of AF, only the prediction code is open, and training a similar model requires a vast amount of computational resources. Thus, developing a lighter prediction model is still necessary.
Results: In this study, we propose a new protein 3D structure modeling method, A-Prot, using MSA Transformer, one of the state-of-the-art protein language models. An MSA feature tensor and row attention maps are extracted and converted into 2D residue-residue distance and dihedral angle redictions for a given MSA. We demonstrated that A-Prot predicts long-range contacts better than the existing methods.
Additionally, we modeled the 3D structures of the free modeling and hard templatebased modeling targets of CASP14. The assessment shows that the A-Prot models are more accurate than most top server groups of CASP14.
Conclusion: These results imply that A-Prot accurately captures the evolutionary and structural information of proteins with relatively low computational cost. Thus, A-Prot can provide a clue for the development of other protein property prediction methods. },
keywords = {},
pubstate = {published},
tppubtype = {article}
}
Results: In this study, we propose a new protein 3D structure modeling method, A-Prot, using MSA Transformer, one of the state-of-the-art protein language models. An MSA feature tensor and row attention maps are extracted and converted into 2D residue-residue distance and dihedral angle redictions for a given MSA. We demonstrated that A-Prot predicts long-range contacts better than the existing methods.
Additionally, we modeled the 3D structures of the free modeling and hard templatebased modeling targets of CASP14. The assessment shows that the A-Prot models are more accurate than most top server groups of CASP14.
Conclusion: These results imply that A-Prot accurately captures the evolutionary and structural information of proteins with relatively low computational cost. Thus, A-Prot can provide a clue for the development of other protein property prediction methods.
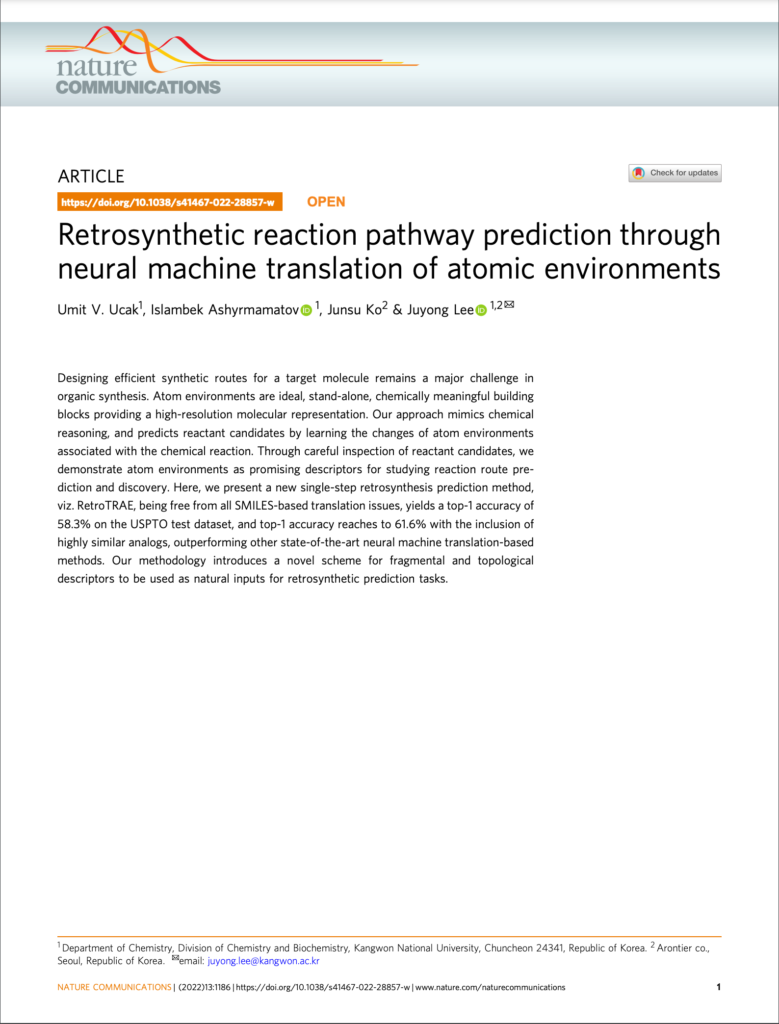
Ucak U V; Ashyrmamatov I; Ko J; Lee J
Retrosynthetic reaction pathway prediction through neural machine translation of atomic environments Journal Article
In: Nat Commun, vol. 13, no. 1, 2022, ISSN: 2041-1723.
@article{Ucak2022b,
title = {Retrosynthetic reaction pathway prediction through neural machine translation of atomic environments},
author = {Umit V. Ucak and Islambek Ashyrmamatov and Junsu Ko and Juyong Lee},
doi = {10.1038/s41467-022-28857-w},
issn = {2041-1723},
year = {2022},
date = {2022-12-00},
urldate = {2022-12-00},
journal = {Nat Commun},
volume = {13},
number = {1},
publisher = {Springer Science and Business Media LLC},
abstract = {Designing efficient synthetic routes for a target molecule remains a major challenge in organic synthesis. Atom environments are ideal, stand-alone, chemically meaningful building blocks providing a high-resolution molecular representation. Our approach mimics chemical reasoning, and predicts reactant candidates by learning the changes of atom environments associated with the chemical reaction. Through careful inspection of reactant candidates, we demonstrate atom environments as promising descriptors for studying reaction route prediction and discovery. Here, we present a new single-step retrosynthesis prediction method, viz. RetroTRAE, being free from all SMILES-based translation issues, yields a top-1 accuracy of 58.3% on the USPTO test dataset, and top-1 accuracy reaches to 61.6% with the inclusion of highly similar analogs, outperforming other state-of-the-art neural machine translation-based methods. Our methodology introduces a novel scheme for fragmental and topological descriptors to be used as natural inputs for retrosynthetic prediction tasks.},
keywords = {},
pubstate = {published},
tppubtype = {article}
}
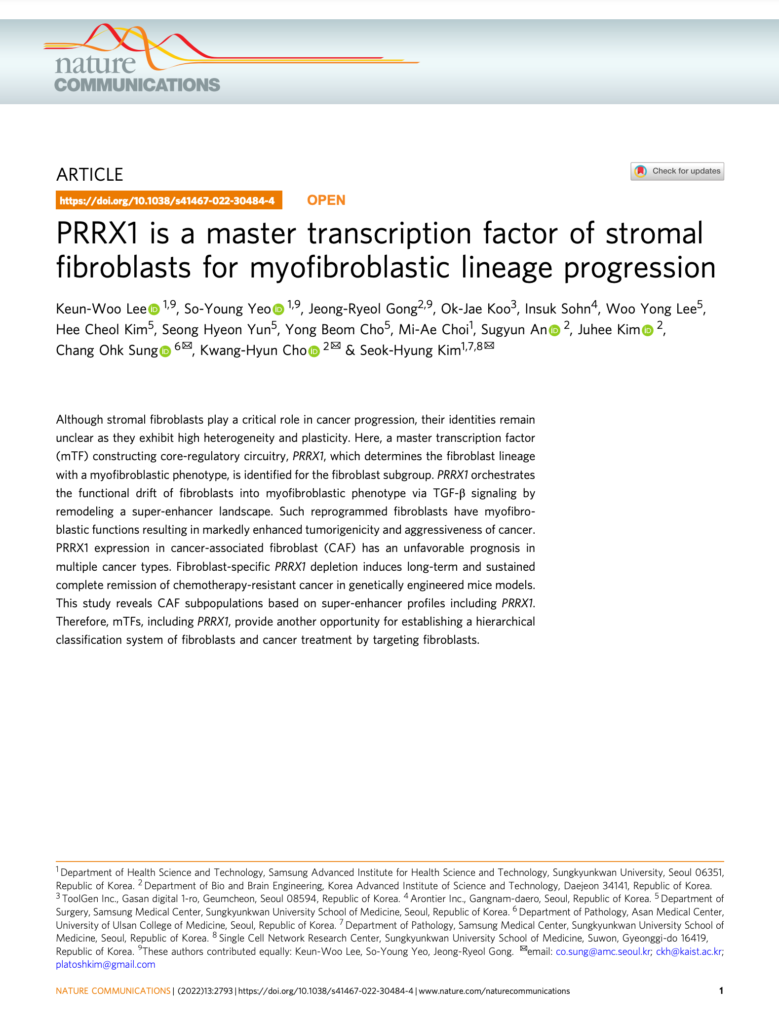
Lee K; Yeo S; Gong J; Koo O; Sohn I; Lee W Y; Kim H C; Yun S H; Cho Y B; Choi M; An S; Kim J; Sung C O; Cho K; Kim S
PRRX1 is a master transcription factor of stromal fibroblasts for myofibroblastic lineage progression Journal Article
In: Nat Commun, vol. 13, no. 1, 2022, ISSN: 2041-1723.
@article{Lee2022,
title = {PRRX1 is a master transcription factor of stromal fibroblasts for myofibroblastic lineage progression},
author = {Keun-Woo Lee and So-Young Yeo and Jeong-Ryeol Gong and Ok-Jae Koo and Insuk Sohn and Woo Yong Lee and Hee Cheol Kim and Seong Hyeon Yun and Yong Beom Cho and Mi-Ae Choi and Sugyun An and Juhee Kim and Chang Ohk Sung and Kwang-Hyun Cho and Seok-Hyung Kim},
doi = {10.1038/s41467-022-30484-4},
issn = {2041-1723},
year = {2022},
date = {2022-12-00},
urldate = {2022-12-00},
journal = {Nat Commun},
volume = {13},
number = {1},
publisher = {Springer Science and Business Media LLC},
abstract = {Although stromal fibroblasts play a critical role in cancer progression, their identities remain unclear as they exhibit high heterogeneity and plasticity. Here, a master transcription factor (mTF) constructing core-regulatory circuitry, PRRX1, which determines the fibroblast lineage with a myofibroblastic phenotype, is identified for the fibroblast subgroup. PRRX1 orchestrates the functional drift of fibroblasts into myofibroblastic phenotype via TGF-β signaling by remodeling a super-enhancer landscape. Such reprogrammed fibroblasts have myofibroblastic functions resulting in markedly enhanced tumorigenicity and aggressiveness of cancer. PRRX1 expression in cancer-associated fibroblast (CAF) has an unfavorable prognosis in multiple cancer types. Fibroblast-specific PRRX1 depletion induces long-term and sustained complete remission of chemotherapy-resistant cancer in genetically engineered mice models. This study reveals CAF subpopulations based on super-enhancer profiles including PRRX1. Therefore, mTFs, including PRRX1, provide another opportunity for establishing a hierarchical classification system of fibroblasts and cancer treatment by targeting fibroblasts.},
keywords = {},
pubstate = {published},
tppubtype = {article}
}
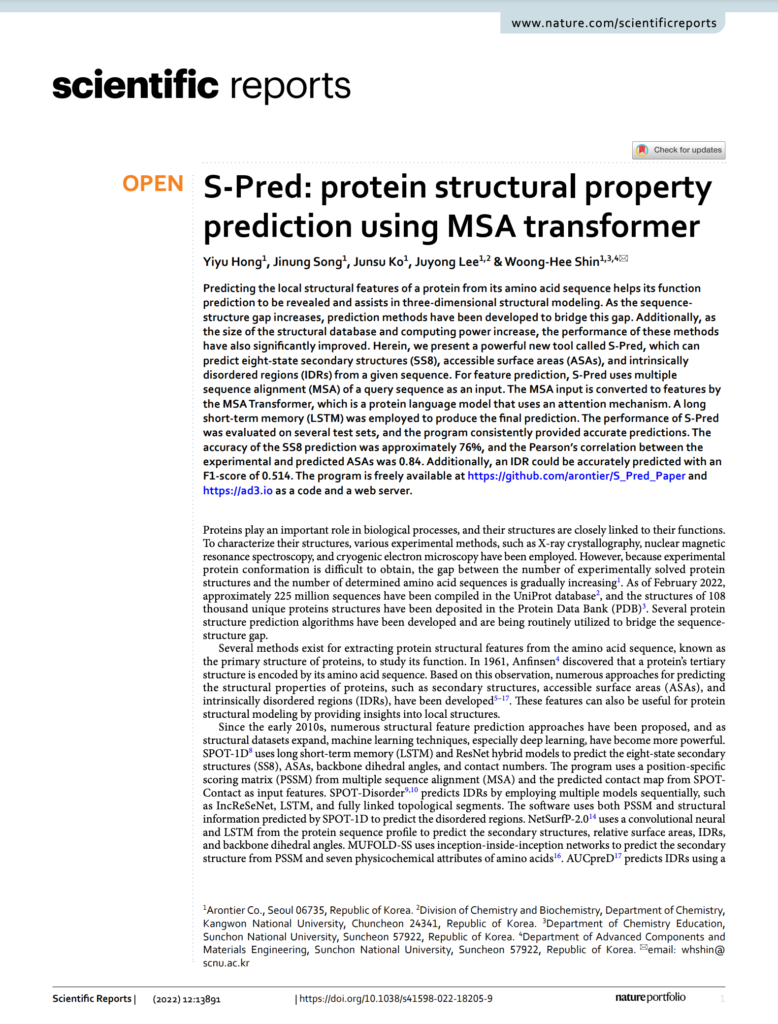
Hong Y; Song J; Ko J; Lee J; Shin W
S-Pred: protein structural property prediction using MSA transformer Journal Article
In: Sci Rep, vol. 12, no. 1, 2022, ISSN: 2045-2322.
@article{Hong2022b,
title = {S-Pred: protein structural property prediction using MSA transformer},
author = {Yiyu Hong and Jinung Song and Junsu Ko and Juyong Lee and Woong-Hee Shin},
doi = {10.1038/s41598-022-18205-9},
issn = {2045-2322},
year = {2022},
date = {2022-12-00},
urldate = {2022-12-00},
journal = {Sci Rep},
volume = {12},
number = {1},
publisher = {Springer Science and Business Media LLC},
abstract = {Predicting the local structural features of a protein from its amino acid sequence helps its function prediction to be revealed and assists in three-dimensional structural modeling. As the sequence-structure gap increases, prediction methods have been developed to bridge this gap. Additionally, as the size of the structural database and computing power increase, the performance of these methods have also significantly improved. Herein, we present a powerful new tool called S-Pred, which can predict eight-state secondary structures (SS8), accessible surface areas (ASAs), and intrinsically disordered regions (IDRs) from a given sequence. For feature prediction, S-Pred uses multiple sequence alignment (MSA) of a query sequence as an input. The MSA input is converted to features by the MSA Transformer, which is a protein language model that uses an attention mechanism. A long short-term memory (LSTM) was employed to produce the final prediction. The performance of S-Pred was evaluated on several test sets, and the program consistently provided accurate predictions. The accuracy of the SS8 prediction was approximately 76%, and the Pearson’s correlation between the experimental and predicted ASAs was 0.84. Additionally, an IDR could be accurately predicted with an F1-score of 0.514. The program is freely available at https://github.com/arontier/S_Pred_Paper and https://ad3.io as a code and a web server.},
keywords = {},
pubstate = {published},
tppubtype = {article}
}
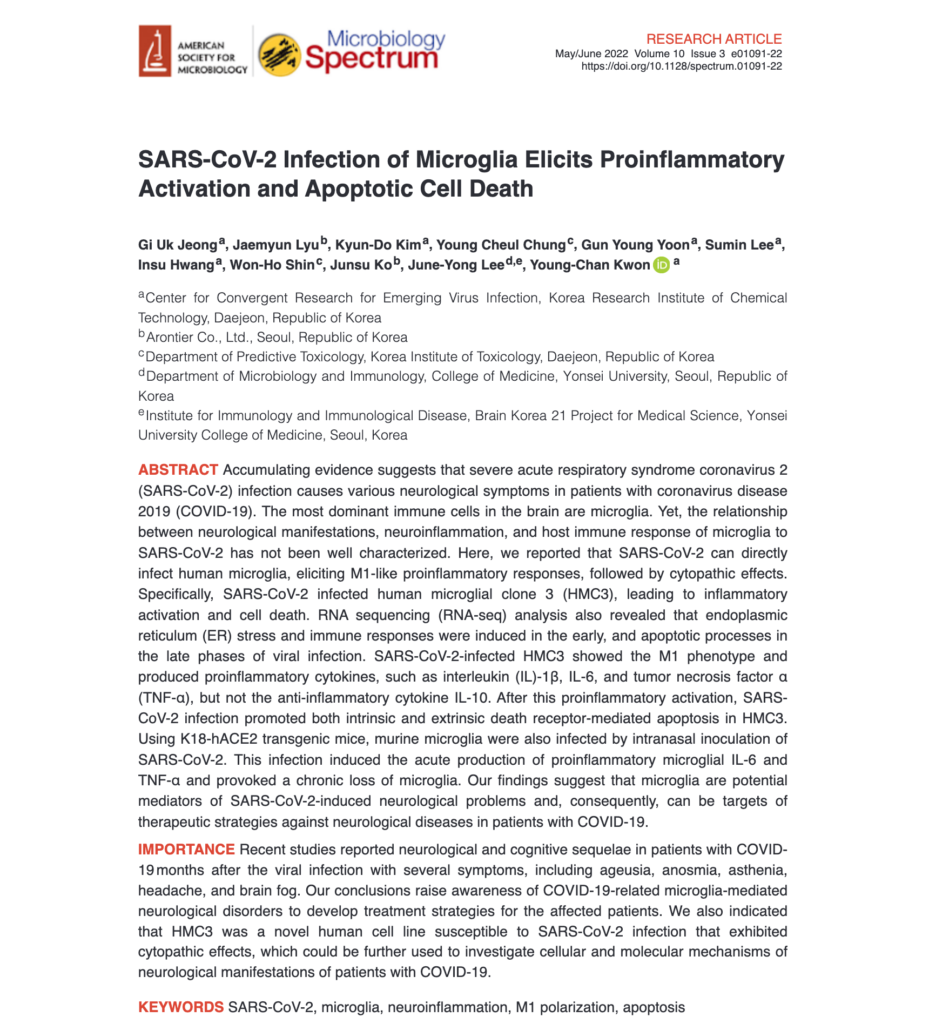
Jeong G U; Lyu J; Kim K; Chung Y C; Yoon G Y; Lee S; Hwang I; Shin W; Ko J; Lee J; Kwon Y
SARS-CoV-2 Infection of Microglia Elicits Proinflammatory Activation and Apoptotic Cell Death Journal Article
In: Microbiol Spectr, vol. 10, no. 3, 2022, ISSN: 2165-0497.
@article{Jeong2022,
title = {SARS-CoV-2 Infection of Microglia Elicits Proinflammatory Activation and Apoptotic Cell Death},
author = {Gi Uk Jeong and Jaemyun Lyu and Kyun-Do Kim and Young Cheul Chung and Gun Young Yoon and Sumin Lee and Insu Hwang and Won-Ho Shin and Junsu Ko and June-Yong Lee and Young-Chan Kwon},
editor = {Ujjwal Neogi},
doi = {10.1128/spectrum.01091-22},
issn = {2165-0497},
year = {2022},
date = {2022-06-29},
urldate = {2022-06-29},
journal = {Microbiol Spectr},
volume = {10},
number = {3},
publisher = {American Society for Microbiology},
abstract = {Accumulating evidence suggests that severe acute respiratory syndrome coronavirus 2 (SARS-CoV-2) infection causes various neurological symptoms in patients with coronavirus disease 2019 (COVID-19). The most dominant immune cells in the brain are microglia. Yet, the relationship between neurological manifestations, neuroinflammation, and host immune response of microglia to SARS-CoV-2 has not been well characterized. Here, we reported that SARS-CoV-2 can directly infect human microglia, eliciting M1-like proinflammatory responses, followed by cytopathic effects. Specifically, SARS-CoV-2 infected human microglial clone 3 (HMC3), leading to inflammatory activation and cell death. RNA sequencing (RNA-seq) analysis also revealed that endoplasmic reticulum (ER) stress and immune responses were induced in the early, and apoptotic processes in the late phases of viral infection. SARS-CoV-2-infected HMC3 showed the M1 phenotype and produced proinflammatory cytokines, such as interleukin (IL)-1β, IL-6, and tumor necrosis factor α (TNF-α), but not the anti-inflammatory cytokine IL-10. After this proinflammatory activation, SARS-CoV-2 infection promoted both intrinsic and extrinsic death receptor-mediated apoptosis in HMC3. Using K18-hACE2 transgenic mice, murine microglia were also infected by intranasal inoculation of SARS-CoV-2. This infection induced the acute production of proinflammatory microglial IL-6 and TNF-α and provoked a chronic loss of microglia. Our findings suggest that microglia are potential mediators of SARS-CoV-2-induced neurological problems and, consequently, can be targets of therapeutic strategies against neurological diseases in patients with COVID-19.
IMPORTANCE Recent studies reported neurological and cognitive sequelae in patients with COVID-19 months after the viral infection with several symptoms, including ageusia, anosmia, asthenia, headache, and brain fog. Our conclusions raise awareness of COVID-19-related microglia-mediated neurological disorders to develop treatment strategies for the affected patients. We also indicated that HMC3 was a novel human cell line susceptible to SARS-CoV-2 infection that exhibited cytopathic effects, which could be further used to investigate cellular and molecular mechanisms of neurological manifestations of patients with COVID-19.},
keywords = {},
pubstate = {published},
tppubtype = {article}
}
IMPORTANCE Recent studies reported neurological and cognitive sequelae in patients with COVID-19 months after the viral infection with several symptoms, including ageusia, anosmia, asthenia, headache, and brain fog. Our conclusions raise awareness of COVID-19-related microglia-mediated neurological disorders to develop treatment strategies for the affected patients. We also indicated that HMC3 was a novel human cell line susceptible to SARS-CoV-2 infection that exhibited cytopathic effects, which could be further used to investigate cellular and molecular mechanisms of neurological manifestations of patients with COVID-19.
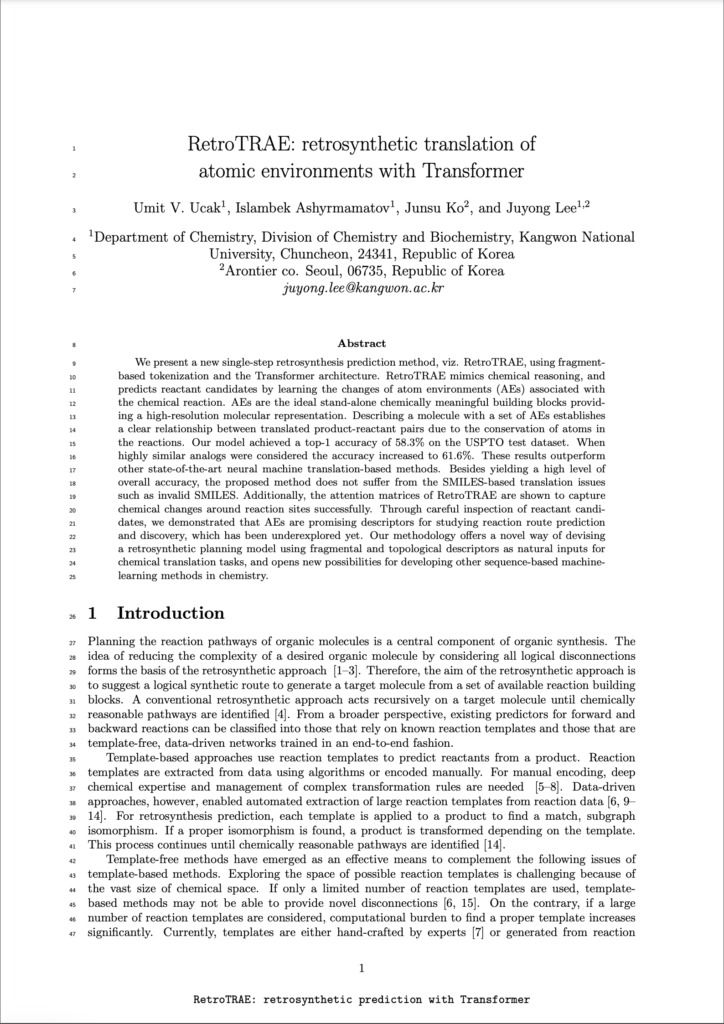
Ucak U V; Ashyrmamatov I; Ko J; Lee J
RetroTRAE: retrosynthetic translation of atomic environments with Transformer Unpublished
2022.
@unpublished{Ucak2022,
title = {RetroTRAE: retrosynthetic translation of atomic environments with Transformer},
author = {Umit Volkan Ucak and Islambek Ashyrmamatov and Junsu Ko and Juyong Lee},
url = {https://chemrxiv.org/engage/chemrxiv/article-details/61f177d7e59d8731714a6a9d},
doi = {10.26434/chemrxiv-2021-9g274-v3},
year = {2022},
date = {2022-01-27},
urldate = {2022-01-27},
publisher = {American Chemical Society (ACS)},
abstract = {We present a new single-step retrosynthesis prediction method, viz. RetroTRAE, using fragment-based tokenization and the Transformer architecture. RetroTRAE mimics chemical reasoning, and predicts reactant candidates by learning the changes of atom environments (AEs) associated with the chemical reaction. AEs are the ideal stand-alone chemically meaningful building blocks providing a high-resolution molecular representation. Describing a molecule with a set of AEs establishes a clear relationship between translated product-reactant pairs due to the conservation of atoms in the reactions. Our model achieved a top-1 accuracy of 58.3% on the USPTO test dataset. When highly similar analogs were considered the accuracy increased to 61.6%. These results outperform other state-of-the-art neural machine translation-based methods. Besides yielding a high level of overall accuracy, the proposed method does not suffer from the SMILES-based translation issues such as invalid SMILES. Additionally, the attention matrices of RetroTRAE are shown to capture chemical changes around reaction sites successfully. Through careful inspection of reactant candidates, we demonstrated that AEs are promising descriptors for studying reaction route prediction and discovery, which has been underexplored yet. Our methodology offers a novel way of devising a retrosynthetic planning model using fragmental and topological descriptors as natural inputs for chemical translation tasks, and opens new possibilities for developing other sequence-based machine-learning methods in chemistry.},
keywords = {},
pubstate = {published},
tppubtype = {unpublished}
}
2021
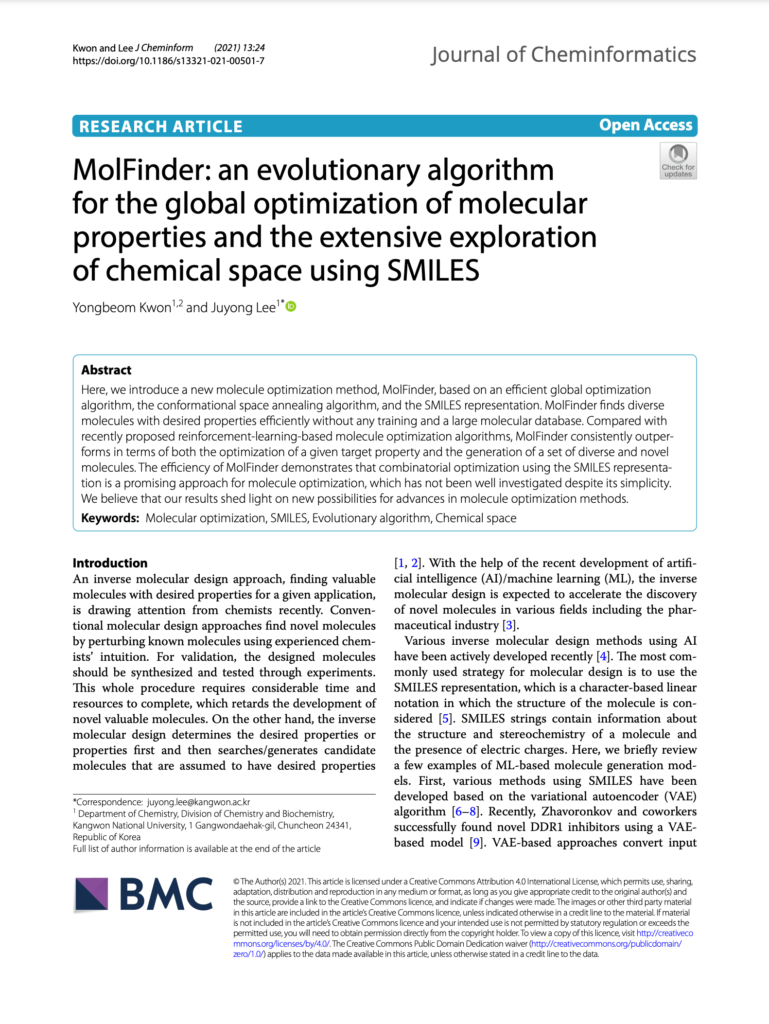
Kwon Y; Lee J
In: J Cheminform, vol. 13, no. 1, 2021, ISSN: 1758-2946.
@article{Kwon2021,
title = {MolFinder: an evolutionary algorithm for the global optimization of molecular properties and the extensive exploration of chemical space using SMILES},
author = {Yongbeom Kwon and Juyong Lee},
doi = {10.1186/s13321-021-00501-7},
issn = {1758-2946},
year = {2021},
date = {2021-12-00},
urldate = {2021-12-00},
journal = {J Cheminform},
volume = {13},
number = {1},
publisher = {Springer Science and Business Media LLC},
abstract = {Here, we introduce a new molecule optimization method, MolFinder, based on an efficient global optimization algorithm, the conformational space annealing algorithm, and the SMILES representation. MolFinder finds diverse molecules with desired properties efficiently without any training and a large molecular database. Compared with recently proposed reinforcement-learning-based molecule optimization algorithms, MolFinder consistently outperforms in terms of both the optimization of a given target property and the generation of a set of diverse and novel molecules. The efficiency of MolFinder demonstrates that combinatorial optimization using the SMILES representation is a promising approach for molecule optimization, which has not been well investigated despite its simplicity. We believe that our results shed light on new possibilities for advances in molecule optimization methods.},
keywords = {},
pubstate = {published},
tppubtype = {article}
}
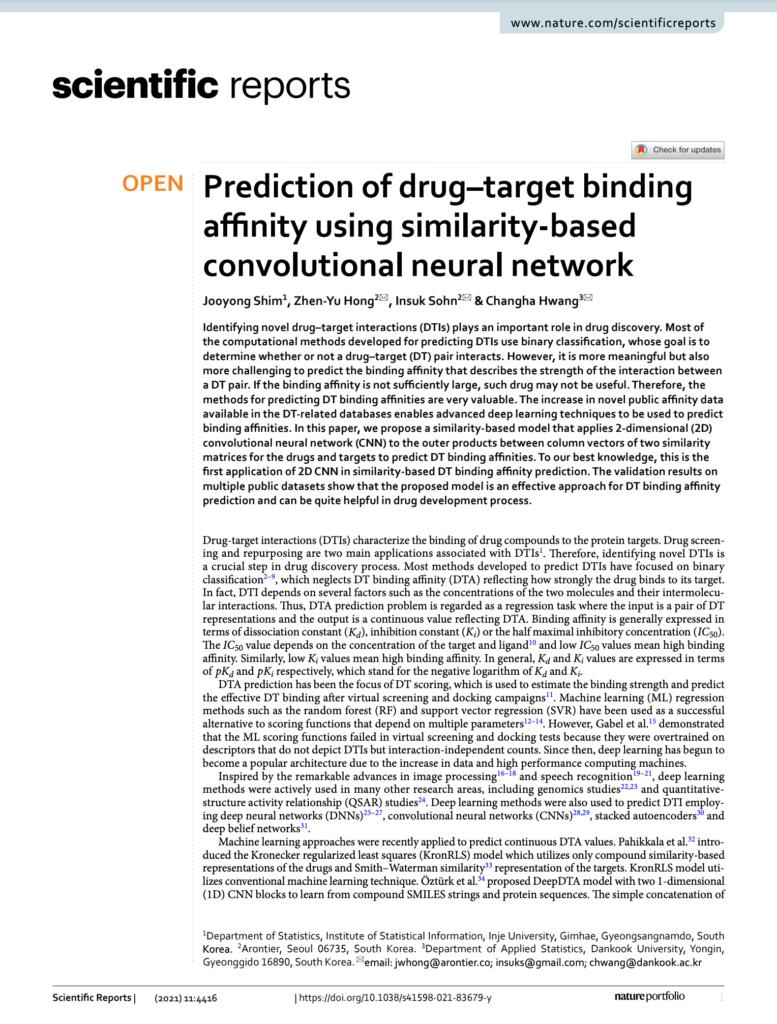
Shim J; Hong Z; Sohn I; Hwang C
Prediction of drug–target binding affinity using similarity-based convolutional neural network Journal Article
In: Sci Rep, vol. 11, no. 1, 2021, ISSN: 2045-2322.
@article{Shim2021,
title = {Prediction of drug–target binding affinity using similarity-based convolutional neural network},
author = {Jooyong Shim and Zhen-Yu Hong and Insuk Sohn and Changha Hwang},
doi = {10.1038/s41598-021-83679-y},
issn = {2045-2322},
year = {2021},
date = {2021-12-00},
urldate = {2021-12-00},
journal = {Sci Rep},
volume = {11},
number = {1},
publisher = {Springer Science and Business Media LLC},
abstract = {Identifying novel drug–target interactions (DTIs) plays an important role in drug discovery. Most of the computational methods developed for predicting DTIs use binary classification, whose goal is to determine whether or not a drug–target (DT) pair interacts. However, it is more meaningful but also more challenging to predict the binding affinity that describes the strength of the interaction between a DT pair. If the binding affinity is not sufficiently large, such drug may not be useful. Therefore, the methods for predicting DT binding affinities are very valuable. The increase in novel public affinity data available in the DT-related databases enables advanced deep learning techniques to be used to predict binding affinities. In this paper, we propose a similarity-based model that applies 2-dimensional (2D) convolutional neural network (CNN) to the outer products between column vectors of two similarity matrices for the drugs and targets to predict DT binding affinities. To our best knowledge, this is the first application of 2D CNN in similarity-based DT binding affinity prediction. The validation results on multiple public datasets show that the proposed model is an effective approach for DT binding affinity prediction and can be quite helpful in drug development process.},
keywords = {},
pubstate = {published},
tppubtype = {article}
}
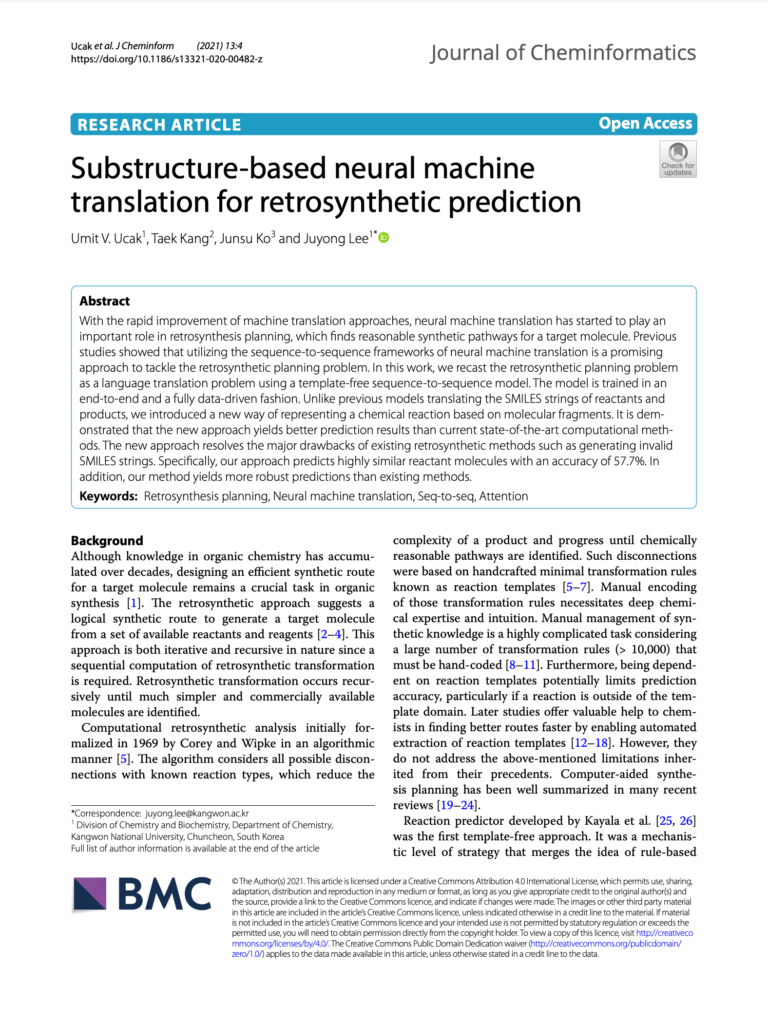
Ucak U V; Kang T; Ko J; Lee J
Substructure-based neural machine translation for retrosynthetic prediction Journal Article
In: J Cheminform, vol. 13, no. 1, 2021, ISSN: 1758-2946.
@article{Ucak2021,
title = {Substructure-based neural machine translation for retrosynthetic prediction},
author = {Umit V. Ucak and Taek Kang and Junsu Ko and Juyong Lee},
doi = {10.1186/s13321-020-00482-z},
issn = {1758-2946},
year = {2021},
date = {2021-12-00},
urldate = {2021-12-00},
journal = {J Cheminform},
volume = {13},
number = {1},
publisher = {Springer Science and Business Media LLC},
abstract = {With the rapid improvement of machine translation approaches, neural machine translation has started to play an important role in retrosynthesis planning, which finds reasonable synthetic pathways for a target molecule. Previous studies showed that utilizing the sequence-to-sequence frameworks of neural machine translation is a promising approach to tackle the retrosynthetic planning problem. In this work, we recast the retrosynthetic planning problem as a language translation problem using a template-free sequence-to-sequence model. The model is trained in an end-to-end and a fully data-driven fashion. Unlike previous models translating the SMILES strings of reactants and products, we introduced a new way of representing a chemical reaction based on molecular fragments. It is demonstrated that the new approach yields better prediction results than current state-of-the-art computational methods. The new approach resolves the major drawbacks of existing retrosynthetic methods such as generating invalid SMILES strings. Specifically, our approach predicts highly similar reactant molecules with an accuracy of 57.7%. In addition, our method yields more robust predictions than existing methods.},
keywords = {},
pubstate = {published},
tppubtype = {article}
}
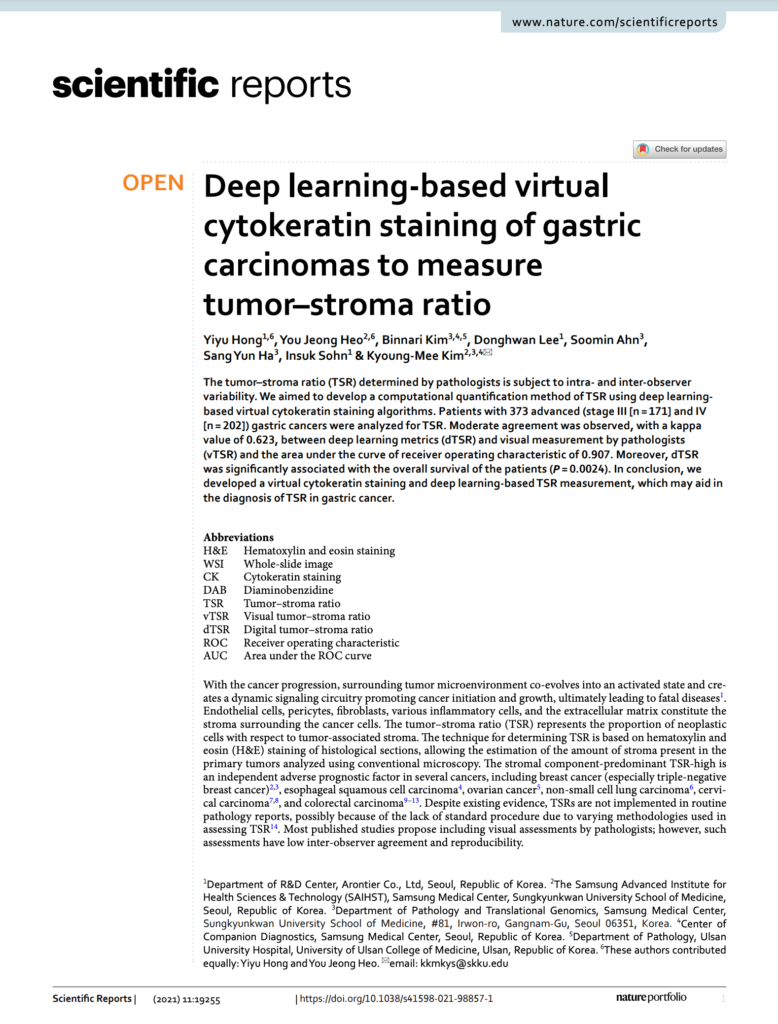
Hong Y; Heo Y J; Kim B; Lee D; Ahn S; Ha S Y; Sohn I; Kim K
Deep learning-based virtual cytokeratin staining of gastric carcinomas to measure tumor–stroma ratio Journal Article
In: Sci Rep, vol. 11, no. 1, 2021, ISSN: 2045-2322.
@article{Hong2021,
title = {Deep learning-based virtual cytokeratin staining of gastric carcinomas to measure tumor–stroma ratio},
author = {Yiyu Hong and You Jeong Heo and Binnari Kim and Donghwan Lee and Soomin Ahn and Sang Yun Ha and Insuk Sohn and Kyoung-Mee Kim},
doi = {10.1038/s41598-021-98857-1},
issn = {2045-2322},
year = {2021},
date = {2021-12-00},
urldate = {2021-12-00},
journal = {Sci Rep},
volume = {11},
number = {1},
publisher = {Springer Science and Business Media LLC},
abstract = {The tumor–stroma ratio (TSR) determined by pathologists is subject to intra- and inter-observer variability. We aimed to develop a computational quantification method of TSR using deep learning-based virtual cytokeratin staining algorithms. Patients with 373 advanced (stage III [n = 171] and IV [n = 202]) gastric cancers were analyzed for TSR. Moderate agreement was observed, with a kappa value of 0.623, between deep learning metrics (dTSR) and visual measurement by pathologists (vTSR) and the area under the curve of receiver operating characteristic of 0.907. Moreover, dTSR was significantly associated with the overall survival of the patients (P = 0.0024). In conclusion, we developed a virtual cytokeratin staining and deep learning-based TSR measurement, which may aid in the diagnosis of TSR in gastric cancer.},
keywords = {},
pubstate = {published},
tppubtype = {article}
}
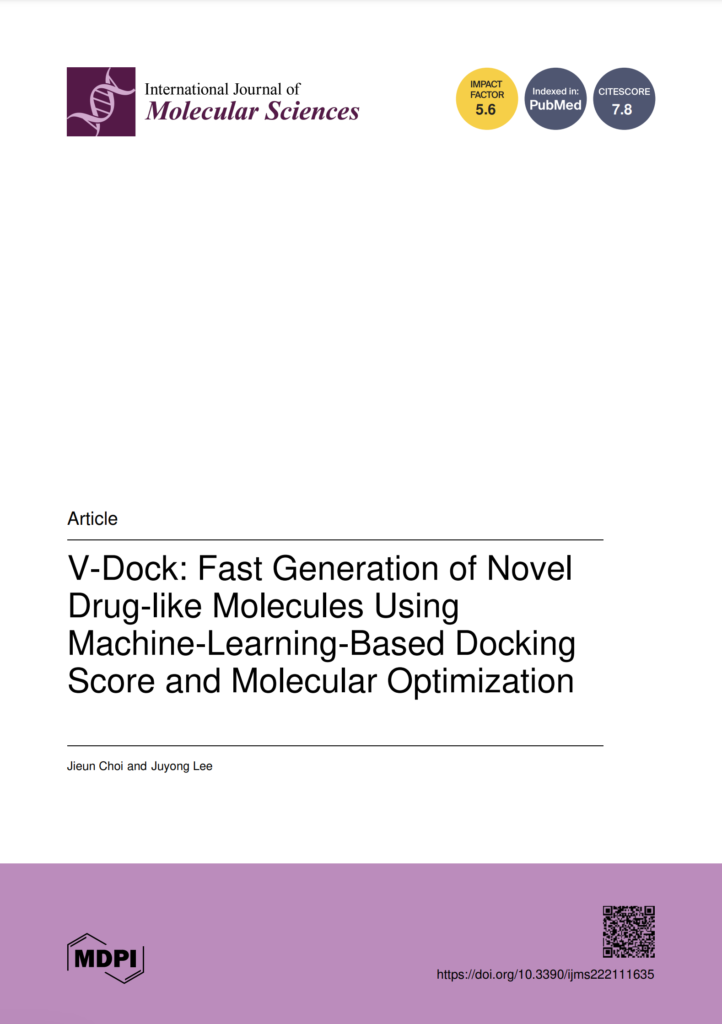
Choi J; Lee J
V-Dock: Fast Generation of Novel Drug-like Molecules Using Machine-Learning-Based Docking Score and Molecular Optimization Journal Article
In: IJMS, vol. 22, no. 21, 2021, ISSN: 1422-0067.
@article{Choi2021,
title = {V-Dock: Fast Generation of Novel Drug-like Molecules Using Machine-Learning-Based Docking Score and Molecular Optimization},
author = {Jieun Choi and Juyong Lee},
doi = {10.3390/ijms222111635},
issn = {1422-0067},
year = {2021},
date = {2021-11-00},
urldate = {2021-11-00},
journal = {IJMS},
volume = {22},
number = {21},
publisher = {MDPI AG},
abstract = {We propose a computational workflow to design novel drug-like molecules by combining the global optimization of molecular properties and protein-ligand docking with machine learning. However, most existing methods depend heavily on experimental data, and many targets do not have sufficient data to train reliable activity prediction models. To overcome this limitation, protein-ligand docking calculations must be performed using the limited data available. Such docking calculations during molecular generation require considerable computational time, preventing extensive exploration of the chemical space. To address this problem, we trained a machine-learning-based model that predicted the docking energy using SMILES to accelerate the molecular generation process. Docking scores could be accurately predicted using only a SMILES string. We combined this docking score prediction model with the global molecular property optimization approach, MolFinder, to find novel molecules exhibiting the desired properties with high values of predicted docking scores. We named this design approach V-dock. Using V-dock, we efficiently generated many novel molecules with high docking scores for a target protein, a similarity to the reference molecule, and desirable drug-like and bespoke properties, such as QED. The predicted docking scores of the generated molecules were verified by correlating them with the actual docking scores.},
keywords = {},
pubstate = {published},
tppubtype = {article}
}
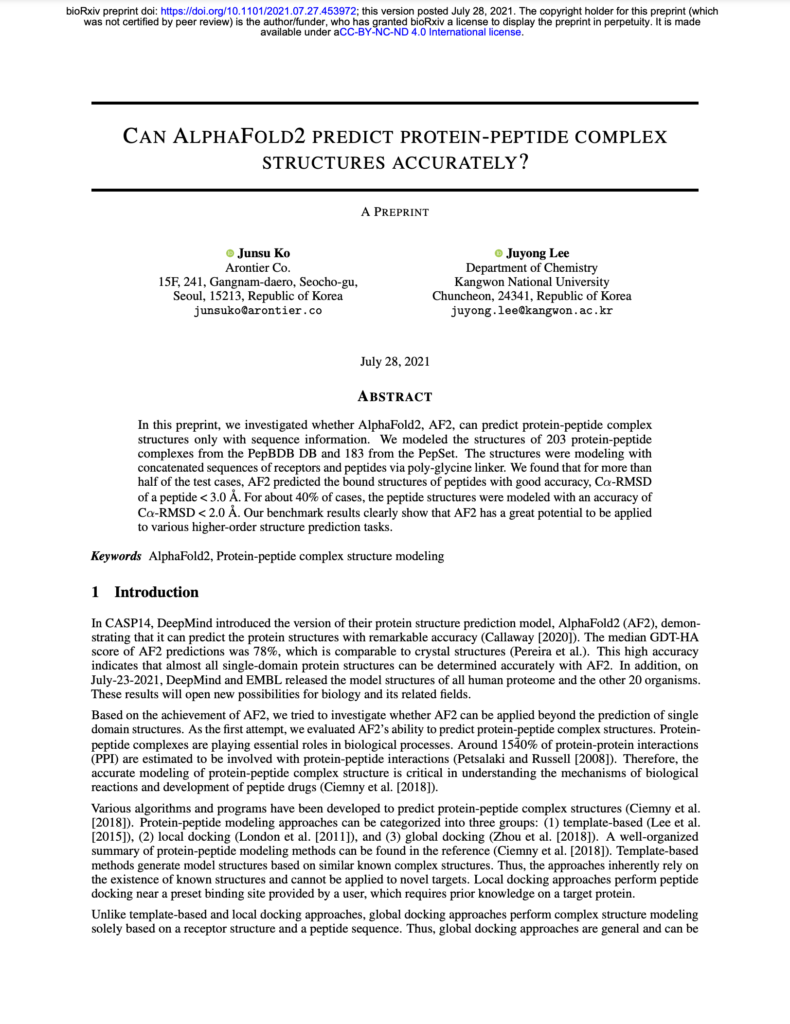
Ko J; Lee J
Can AlphaFold2 predict protein-peptide complex structures accurately? Unpublished
bioRxiv, 2021.
@unpublished{Ko2021,
title = {Can AlphaFold2 predict protein-peptide complex structures accurately?},
author = {Junsu Ko and Juyong Lee},
url = {http://biorxiv.org/lookup/doi/10.1101/2021.07.27.453972},
doi = {10.1101/2021.07.27.453972},
year = {2021},
date = {2021-07-27},
urldate = {2021-07-27},
publisher = {Cold Spring Harbor Laboratory},
abstract = {In this preprint, we investigated whether AlphaFold2, AF2, can predict protein-peptide complex structures only with sequence information. We modeled the structures of 203 protein-peptide complexes from the PepBDB DB and 183 from the PepSet. The structures were modeling with concatenated sequences of receptors and peptides via poly-glycine linker. We found that for more than half of the test cases, AF2 predicted the bound structures of peptides with good accuracy, Cα-RMSD of a peptide < 3.0 Å. For about 40% of cases, the peptide structures were modeled with an accuracy of Cα-RMSD < 2.0 Å. Our benchmark results clearly show that AF2 has a great potential to be applied to various higher-order structure prediction tasks.},
howpublished = {bioRxiv},
keywords = {},
pubstate = {published},
tppubtype = {unpublished}
}
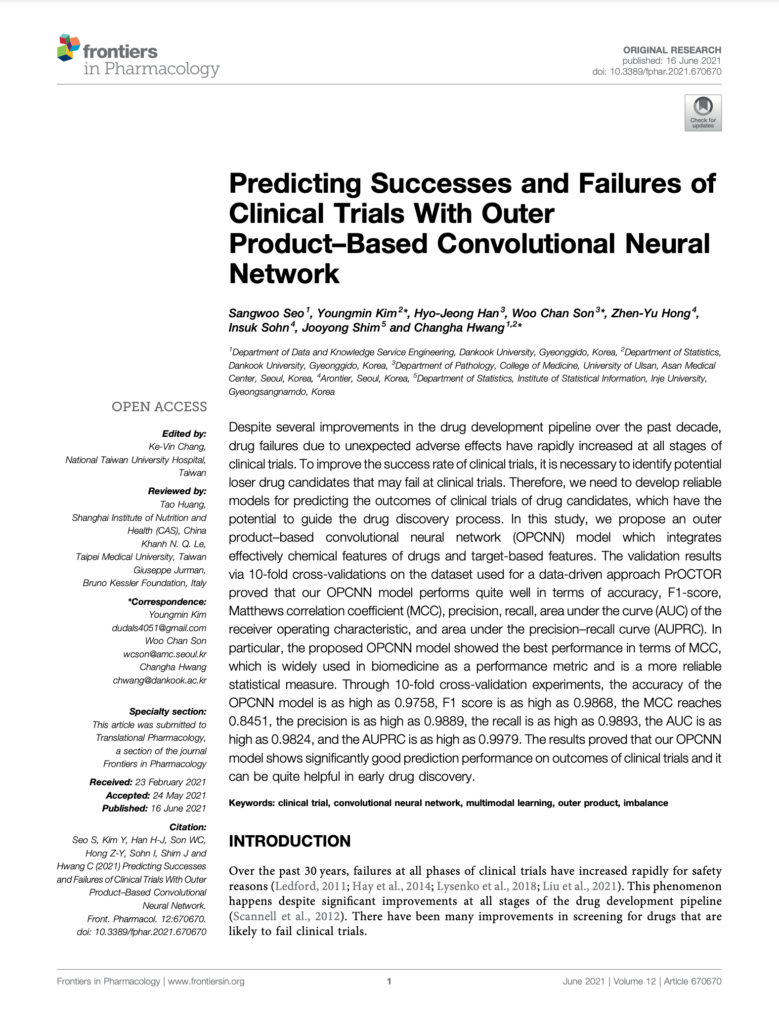
Seo S; Kim Y; Han H; Son W C; Hong Z; Sohn I; Shim J; Hwang C
Predicting Successes and Failures of Clinical Trials With Outer Product–Based Convolutional Neural Network Journal Article
In: Front. Pharmacol., vol. 12, 2021, ISSN: 1663-9812.
@article{Seo2021,
title = {Predicting Successes and Failures of Clinical Trials With Outer Product–Based Convolutional Neural Network},
author = {Sangwoo Seo and Youngmin Kim and Hyo-Jeong Han and Woo Chan Son and Zhen-Yu Hong and Insuk Sohn and Jooyong Shim and Changha Hwang},
doi = {10.3389/fphar.2021.670670},
issn = {1663-9812},
year = {2021},
date = {2021-06-16},
urldate = {2021-06-16},
journal = {Front. Pharmacol.},
volume = {12},
publisher = {Frontiers Media SA},
abstract = {Despite several improvements in the drug development pipeline over the past decade, drug failures due to unexpected adverse effects have rapidly increased at all stages of clinical trials. To improve the success rate of clinical trials, it is necessary to identify potential loser drug candidates that may fail at clinical trials. Therefore, we need to develop reliable models for predicting the outcomes of clinical trials of drug candidates, which have the potential to guide the drug discovery process. In this study, we propose an outer product–based convolutional neural network (OPCNN) model which integrates effectively chemical features of drugs and target-based features. The validation results via 10-fold cross-validations on the dataset used for a data-driven approach PrOCTOR proved that our OPCNN model performs quite well in terms of accuracy, F1-score, Matthews correlation coefficient (MCC), precision, recall, area under the curve (AUC) of the receiver operating characteristic, and area under the precision–recall curve (AUPRC). In particular, the proposed OPCNN model showed the best performance in terms of MCC, which is widely used in biomedicine as a performance metric and is a more reliable statistical measure. Through 10-fold cross-validation experiments, the accuracy of the OPCNN model is as high as 0.9758, F1 score is as high as 0.9868, the MCC reaches 0.8451, the precision is as high as 0.9889, the recall is as high as 0.9893, the AUC is as high as 0.9824, and the AUPRC is as high as 0.9979. The results proved that our OPCNN model shows significantly good prediction performance on outcomes of clinical trials and it can be quite helpful in early drug discovery.},
keywords = {},
pubstate = {published},
tppubtype = {article}
}
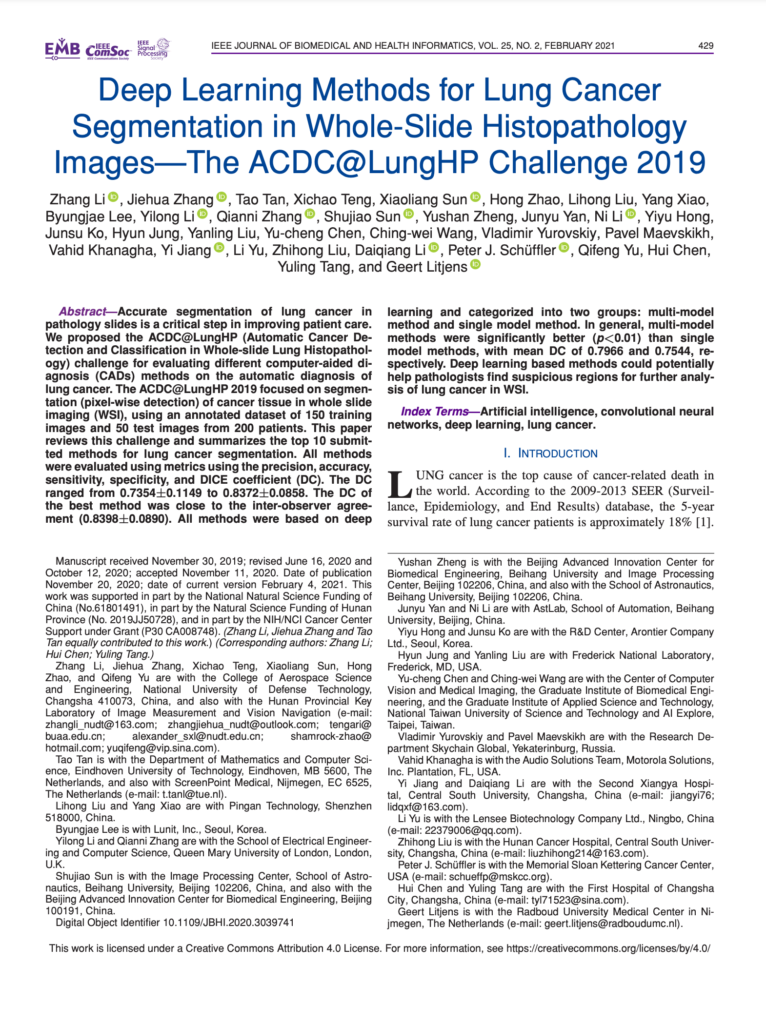
Li Z; Zhang J; Tan T; Teng X; Sun X; Zhao H; Liu L; Xiao Y; Lee B; Li Y; Zhang Q; Sun S; Zheng Y; Yan J; Li N; Hong Y; Ko J; Jung H; Liu Y; Chen Y; Wang C; Yurovskiy V; Maevskikh P; Khanagha V; Jiang Y; Yu L; Liu Z; Li D; Schuffler P J; Yu Q; Chen H; Tang Y; Litjens G
Deep Learning Methods for Lung Cancer Segmentation in Whole-Slide Histopathology Images—The ACDC@LungHP Challenge 2019 Journal Article
In: IEEE J. Biomed. Health Inform., vol. 25, no. 2, pp. 429–440, 2021, ISSN: 2168-2208.
@article{Li2021,
title = {Deep Learning Methods for Lung Cancer Segmentation in Whole-Slide Histopathology Images—The ACDC@LungHP Challenge 2019},
author = {Zhang Li and Jiehua Zhang and Tao Tan and Xichao Teng and Xiaoliang Sun and Hong Zhao and Lihong Liu and Yang Xiao and Byungjae Lee and Yilong Li and Qianni Zhang and Shujiao Sun and Yushan Zheng and Junyu Yan and Ni Li and Yiyu Hong and Junsu Ko and Hyun Jung and Yanling Liu and Yu-cheng Chen and Ching-wei Wang and Vladimir Yurovskiy and Pavel Maevskikh and Vahid Khanagha and Yi Jiang and Li Yu and Zhihong Liu and Daiqiang Li and Peter J. Schuffler and Qifeng Yu and Hui Chen and Yuling Tang and Geert Litjens},
doi = {10.1109/jbhi.2020.3039741},
issn = {2168-2208},
year = {2021},
date = {2021-02-00},
urldate = {2021-02-00},
journal = {IEEE J. Biomed. Health Inform.},
volume = {25},
number = {2},
pages = {429--440},
publisher = {Institute of Electrical and Electronics Engineers (IEEE)},
abstract = {Accurate segmentation of lung cancer in pathology slides is a critical step in improving patient care. We proposed the ACDC@LungHP (Automatic Cancer Detection and Classification in Whole-slide Lung Histopathology) challenge for evaluating different computer-aided diagnosis (CADs) methods on the automatic diagnosis of lung cancer. The ACDC@LungHP 2019 focused on segmentation (pixel-wise detection) of cancer tissue in whole slide imaging (WSI), using an annotated dataset of 150 training images and 50 test images from 200 patients. This paper reviews this challenge and summarizes the top 10 submitted methods for lung cancer segmentation. All methods were evaluated using metrics using the precision, accuracy, sensitivity, specificity, and DICE coefficient (DC). The DC ranged from 0.7354 ± 0.1149 to 0.8372 ± 0.0858. The DC of the best method was close to the inter-observer agreement (0.8398 ± 0.0890). All methods were based on deep learning and categorized into two groups: multi-model method and single model method. In general, multi-model methods were significantly better ( p < 0.01) than single model methods, with mean DC of 0.7966 and 0.7544, respectively. Deep learning based methods could potentially help pathologists find suspicious regions for further analysis of lung cancer in WSI.},
keywords = {},
pubstate = {published},
tppubtype = {article}
}
2020
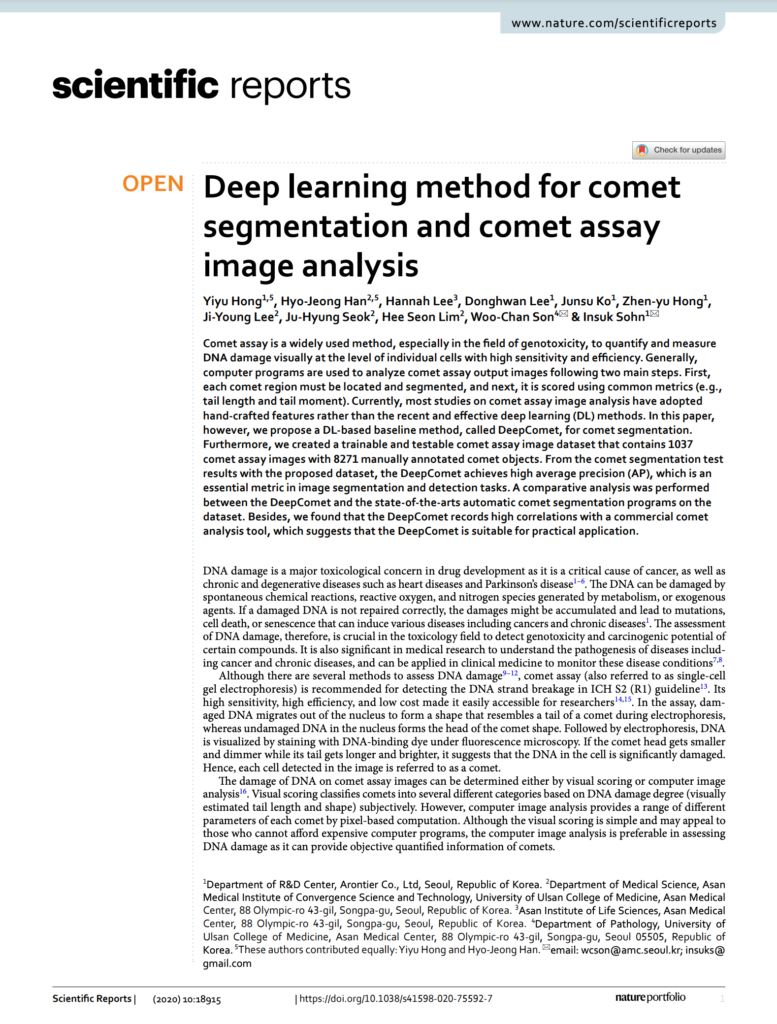
Hong Y; Han H; Lee H; Lee D; Ko J; Hong Z; Lee J; Seok J; Lim H S; Son W; Sohn I
Deep learning method for comet segmentation and comet assay image analysis Journal Article
In: Sci Rep, vol. 10, no. 1, 2020, ISSN: 2045-2322.
@article{Hong2020,
title = {Deep learning method for comet segmentation and comet assay image analysis},
author = {Yiyu Hong and Hyo-Jeong Han and Hannah Lee and Donghwan Lee and Junsu Ko and Zhen-yu Hong and Ji-Young Lee and Ju-Hyung Seok and Hee Seon Lim and Woo-Chan Son and Insuk Sohn},
doi = {10.1038/s41598-020-75592-7},
issn = {2045-2322},
year = {2020},
date = {2020-12-00},
urldate = {2020-12-00},
journal = {Sci Rep},
volume = {10},
number = {1},
publisher = {Springer Science and Business Media LLC},
abstract = {Comet assay is a widely used method, especially in the field of genotoxicity, to quantify and measure DNA damage visually at the level of individual cells with high sensitivity and efficiency. Generally, computer programs are used to analyze comet assay output images following two main steps. First, each comet region must be located and segmented, and next, it is scored using common metrics (e.g., tail length and tail moment). Currently, most studies on comet assay image analysis have adopted hand-crafted features rather than the recent and effective deep learning (DL) methods. In this paper, however, we propose a DL-based baseline method, called DeepComet, for comet segmentation. Furthermore, we created a trainable and testable comet assay image dataset that contains 1037 comet assay images with 8271 manually annotated comet objects. From the comet segmentation test results with the proposed dataset, the DeepComet achieves high average precision (AP), which is an essential metric in image segmentation and detection tasks. A comparative analysis was performed between the DeepComet and the state-of-the-arts automatic comet segmentation programs on the dataset. Besides, we found that the DeepComet records high correlations with a commercial comet analysis tool, which suggests that the DeepComet is suitable for practical application.},
keywords = {},
pubstate = {published},
tppubtype = {article}
}
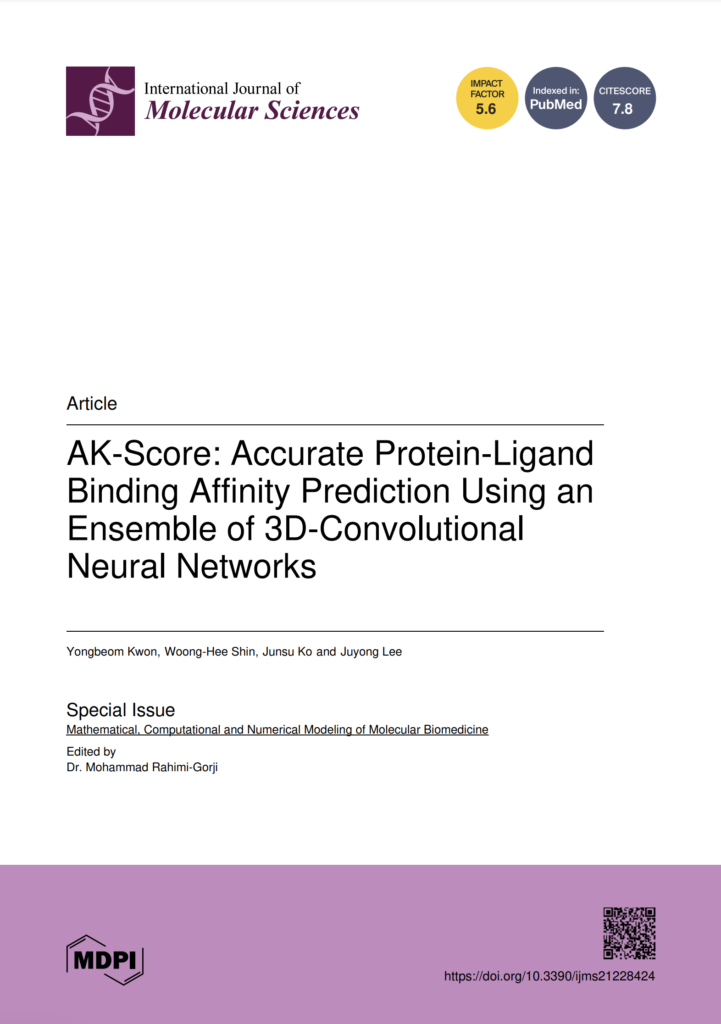
Kwon Y; Shin W; Ko J; Lee J
AK-Score: Accurate Protein-Ligand Binding Affinity Prediction Using an Ensemble of 3D-Convolutional Neural Networks Journal Article
In: IJMS, vol. 21, no. 22, 2020, ISSN: 1422-0067.
@article{Kwon2020,
title = {AK-Score: Accurate Protein-Ligand Binding Affinity Prediction Using an Ensemble of 3D-Convolutional Neural Networks},
author = {Yongbeom Kwon and Woong-Hee Shin and Junsu Ko and Juyong Lee},
doi = {10.3390/ijms21228424},
issn = {1422-0067},
year = {2020},
date = {2020-11-00},
urldate = {2020-11-00},
journal = {IJMS},
volume = {21},
number = {22},
publisher = {MDPI AG},
abstract = {Accurate prediction of the binding affinity of a protein-ligand complex is essential for efficient and successful rational drug design. Therefore, many binding affinity prediction methods have been developed. In recent years, since deep learning technology has become powerful, it is also implemented to predict affinity. In this work, a new neural network model that predicts the binding affinity of a protein-ligand complex structure is developed. Our model predicts the binding affinity of a complex using the ensemble of multiple independently trained networks that consist of multiple channels of 3-D convolutional neural network layers. Our model was trained using the 3772 protein-ligand complexes from the refined set of the PDBbind-2016 database and tested using the core set of 285 complexes. The benchmark results show that the Pearson correlation coefficient between the predicted binding affinities by our model and the experimental data is 0.827, which is higher than the state-of-the-art binding affinity prediction scoring functions. Additionally, our method ranks the relative binding affinities of possible multiple binders of a protein quite accurately, comparable to the other scoring functions. Last, we measured which structural information is critical for predicting binding affinity and found that the complementarity between the protein and ligand is most important.},
keywords = {},
pubstate = {published},
tppubtype = {article}
}
2024
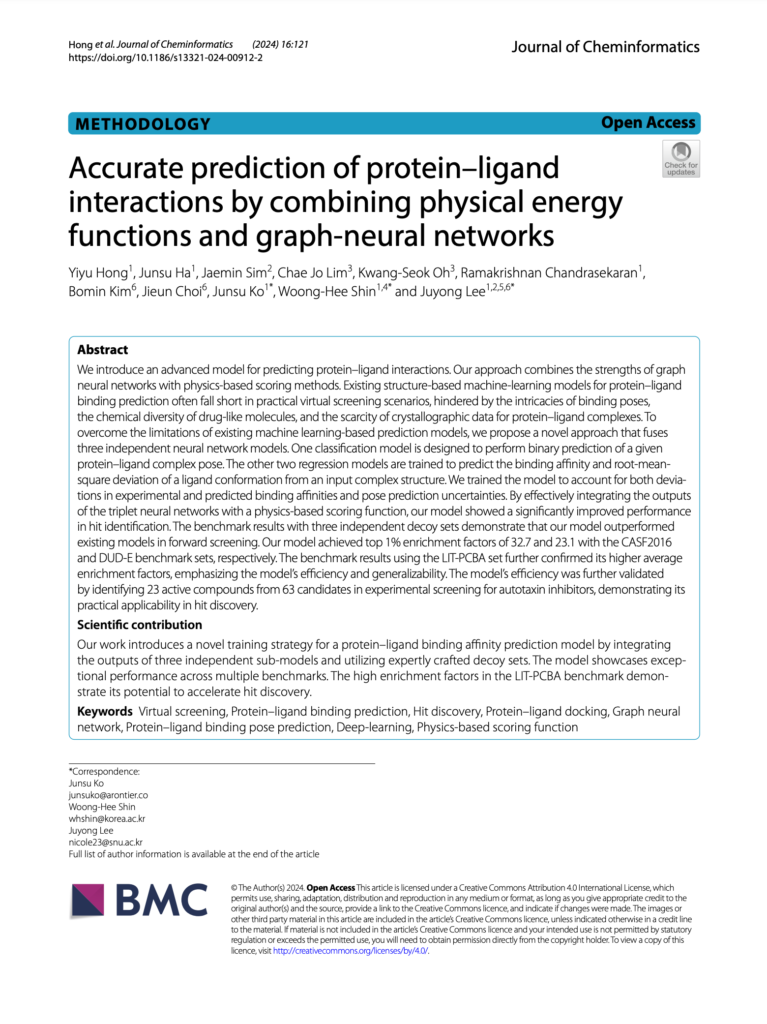
Hong Y; Ha J; Sim J; Lim C J; Oh K; Chandrasekaran R; Kim B; Choi J; Ko J; Shin W; Lee J
Accurate prediction of protein–ligand interactions by combining physical energy functions and graph-neural networks Journal Article
In: J Cheminform, vol. 16, no. 1, 2024, ISSN: 1758-2946.
@article{Hong2024,
title = {Accurate prediction of protein–ligand interactions by combining physical energy functions and graph-neural networks},
author = {Yiyu Hong and Junsu Ha and Jaemin Sim and Chae Jo Lim and Kwang-Seok Oh and Ramakrishnan Chandrasekaran and Bomin Kim and Jieun Choi and Junsu Ko and Woong-Hee Shin and Juyong Lee},
doi = {10.1186/s13321-024-00912-2},
issn = {1758-2946},
year = {2024},
date = {2024-11-04},
urldate = {2024-11-04},
journal = {J Cheminform},
volume = {16},
number = {1},
publisher = {Springer Science and Business Media LLC},
abstract = {We introduce an advanced model for predicting protein–ligand interactions. Our approach combines the strengths of graph neural networks with physics-based scoring methods. Existing structure-based machine-learning models for protein–ligand binding prediction often fall short in practical virtual screening scenarios, hindered by the intricacies of binding poses, the chemical diversity of drug-like molecules, and the scarcity of crystallographic data for protein–ligand complexes. To overcome the limitations of existing machine learning-based prediction models, we propose a novel approach that fuses three independent neural network models. One classification model is designed to perform binary prediction of a given protein–ligand complex pose. The other two regression models are trained to predict the binding affinity and root-mean-square deviation of a ligand conformation from an input complex structure. We trained the model to account for both deviations in experimental and predicted binding affinities and pose prediction uncertainties. By effectively integrating the outputs of the triplet neural networks with a physics-based scoring function, our model showed a significantly improved performance in hit identification. The benchmark results with three independent decoy sets demonstrate that our model outperformed existing models in forward screening. Our model achieved top 1% enrichment factors of 32.7 and 23.1 with the CASF2016 and DUD-E benchmark sets, respectively. The benchmark results using the LIT-PCBA set further confirmed its higher average enrichment factors, emphasizing the model’s efficiency and generalizability. The model’s efficiency was further validated by identifying 23 active compounds from 63 candidates in experimental screening for autotaxin inhibitors, demonstrating its practical applicability in hit discovery.
Scientific contribution Our work introduces a novel training strategy for a protein–ligand binding affinity prediction model by integrating the outputs of three independent sub-models and utilizing expertly crafted decoy sets. The model showcases exceptional performance across multiple benchmarks. The high enrichment factors in the LIT-PCBA benchmark demonstrate its potential to accelerate hit discovery.},
keywords = {},
pubstate = {published},
tppubtype = {article}
}
Scientific contribution Our work introduces a novel training strategy for a protein–ligand binding affinity prediction model by integrating the outputs of three independent sub-models and utilizing expertly crafted decoy sets. The model showcases exceptional performance across multiple benchmarks. The high enrichment factors in the LIT-PCBA benchmark demonstrate its potential to accelerate hit discovery.
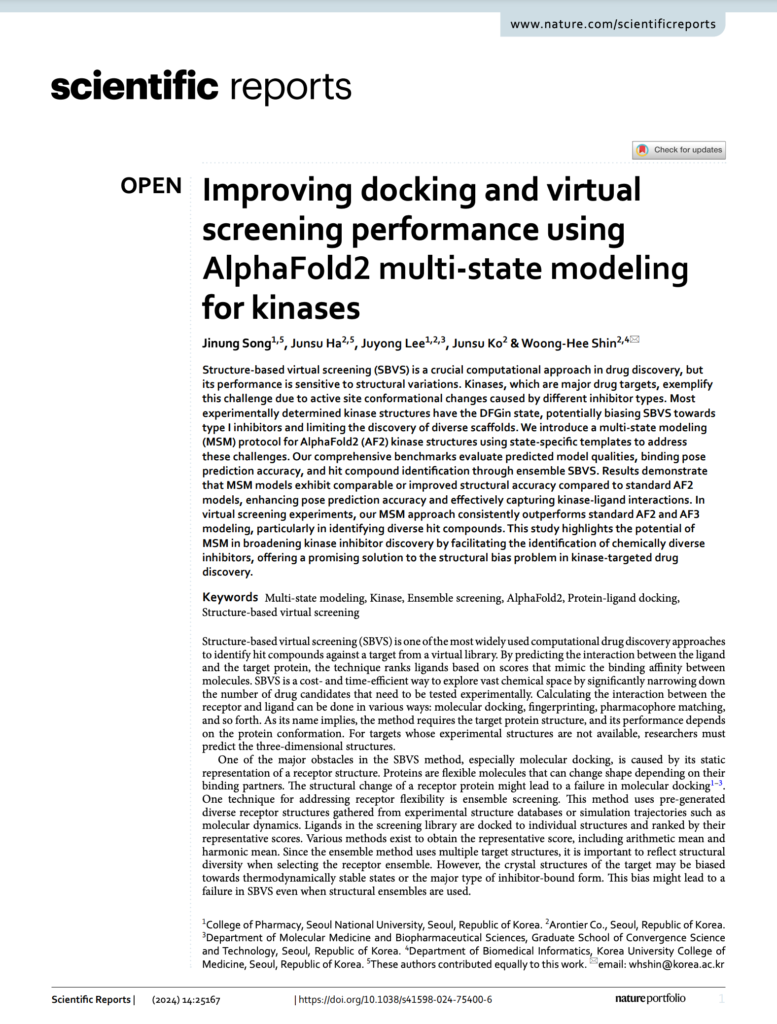
Song J; Ha J; Lee J; Ko J; Shin W
Improving docking and virtual screening performance using AlphaFold2 multi-state modeling for kinases Journal Article
In: Sci Rep, vol. 14, no. 1, 2024, ISSN: 2045-2322.
@article{Song2024b,
title = {Improving docking and virtual screening performance using AlphaFold2 multi-state modeling for kinases},
author = {Jinung Song and Junsu Ha and Juyong Lee and Junsu Ko and Woong-Hee Shin},
doi = {10.1038/s41598-024-75400-6},
issn = {2045-2322},
year = {2024},
date = {2024-10-24},
urldate = {2024-10-24},
journal = {Sci Rep},
volume = {14},
number = {1},
publisher = {Springer Science and Business Media LLC},
abstract = {Structure-based virtual screening (SBVS) is a crucial computational approach in drug discovery, but its performance is sensitive to structural variations. Kinases, which are major drug targets, exemplify this challenge due to active site conformational changes caused by different inhibitor types. Most experimentally determined kinase structures have the DFGin state, potentially biasing SBVS towards type I inhibitors and limiting the discovery of diverse scaffolds. We introduce a multi-state modeling (MSM) protocol for AlphaFold2 (AF2) kinase structures using state-specific templates to address these challenges. Our comprehensive benchmarks evaluate predicted model qualities, binding pose prediction accuracy, and hit compound identification through ensemble SBVS. Results demonstrate that MSM models exhibit comparable or improved structural accuracy compared to standard AF2 models, enhancing pose prediction accuracy and effectively capturing kinase-ligand interactions. In virtual screening experiments, our MSM approach consistently outperforms standard AF2 and AF3 modeling, particularly in identifying diverse hit compounds. This study highlights the potential of MSM in broadening kinase inhibitor discovery by facilitating the identification of chemically diverse inhibitors, offering a promising solution to the structural bias problem in kinase-targeted drug discovery.},
keywords = {},
pubstate = {published},
tppubtype = {article}
}
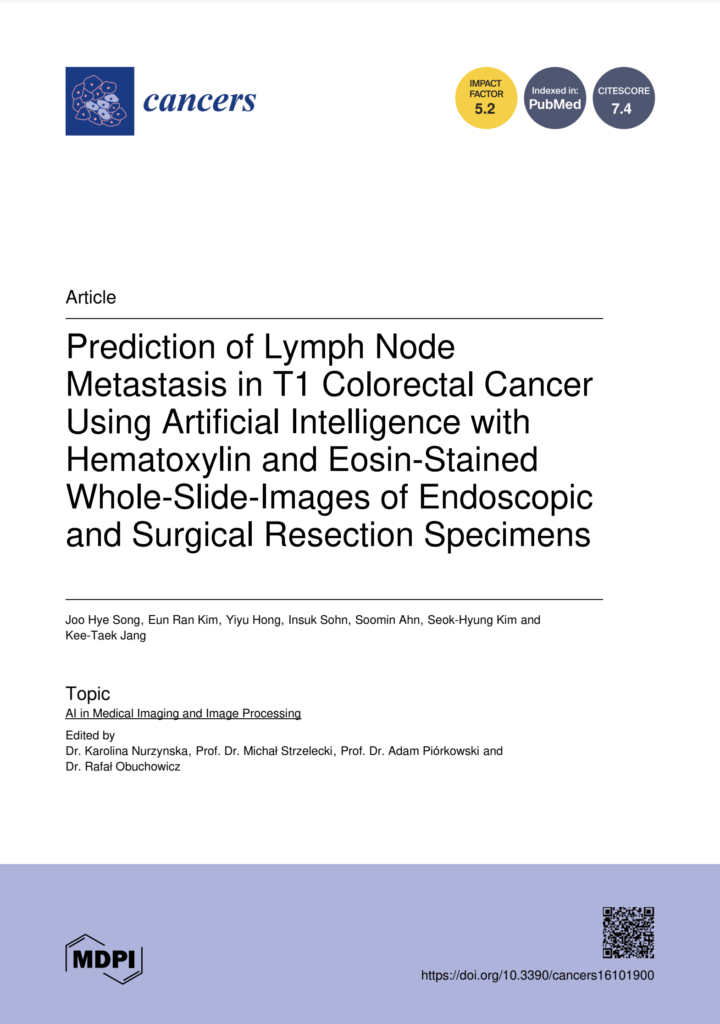
Song J H; Kim E R; Hong Y; Sohn I; Ahn S; Kim S; Jang K
In: Cancers, vol. 16, no. 10, 2024, ISSN: 2072-6694.
@article{Song2024,
title = {Prediction of Lymph Node Metastasis in T1 Colorectal Cancer Using Artificial Intelligence with Hematoxylin and Eosin-Stained Whole-Slide-Images of Endoscopic and Surgical Resection Specimens},
author = {Joo Hye Song and Eun Ran Kim and Yiyu Hong and Insuk Sohn and Soomin Ahn and Seok-Hyung Kim and Kee-Taek Jang},
doi = {10.3390/cancers16101900},
issn = {2072-6694},
year = {2024},
date = {2024-05-16},
urldate = {2024-05-16},
journal = {Cancers},
volume = {16},
number = {10},
publisher = {MDPI AG},
abstract = {According to the current guidelines, additional surgery is performed for endoscopically resected specimens of early colorectal cancer (CRC) with a high risk of lymph node metastasis (LNM). However, the rate of LNM is 2.1–25.0% in cases treated endoscopically followed by surgery, indicating a high rate of unnecessary surgeries. Therefore, this study aimed to develop an artificial intelligence (AI) model using H&E-stained whole slide images (WSIs) without handcrafted features employing surgically and endoscopically resected specimens to predict LNM in T1 CRC. To validate with an independent cohort, we developed a model with four versions comprising various combinations of training and test sets using H&E-stained WSIs from endoscopically (400 patients) and surgically resected specimens (881 patients): Version 1, Train and Test: surgical specimens; Version 2, Train and Test: endoscopic and surgically resected specimens; Version 3, Train: endoscopic and surgical specimens and Test: surgical specimens; Version 4, Train: endoscopic and surgical specimens and Test: endoscopic specimens. The area under the curve (AUC) of the receiver operating characteristic curve was used to determine the accuracy of the AI model for predicting LNM with a 5-fold cross-validation in the training set. Our AI model with H&E-stained WSIs and without annotations showed good performance power with the validation of an independent cohort in a single center. The AUC of our model was 0.758–0.830 in the training set and 0.781–0.824 in the test set, higher than that of previous AI studies with only WSI. Moreover, the AI model with Version 4, which showed the highest sensitivity (92.9%), reduced unnecessary additional surgery by 14.2% more than using the current guidelines (68.3% vs. 82.5%). This revealed the feasibility of using an AI model with only H&E-stained WSIs to predict LNM in T1 CRC.},
keywords = {},
pubstate = {published},
tppubtype = {article}
}
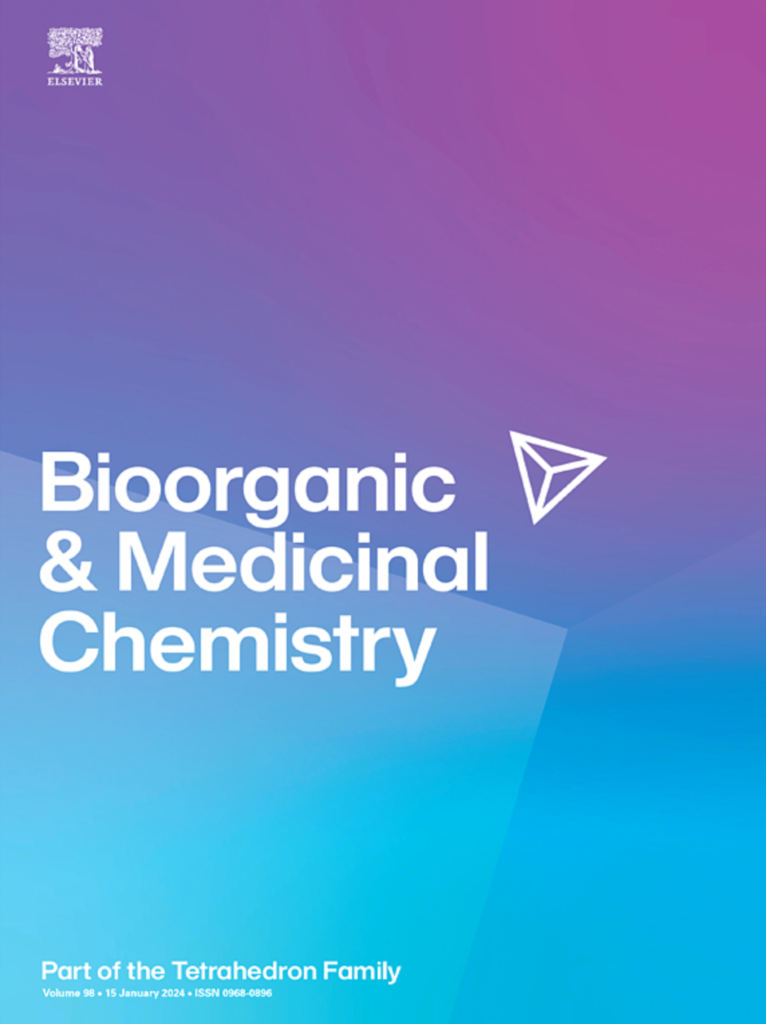
Yang H; Kang M; Jang S; Baek S Y; Kim J; Kim G U; Kim D; Ha J; Kim J S; Jung C; Kim N; Cho S; Shin W; Lee J; Ko J; Lee A; Keum G; Lee S; Kang T
Discovery of thiophen-2-ylmethylene bis-dimedone derivatives as novel WRN inhibitors for treating cancers with microsatellite instability Journal Article
In: Bioorganic & Medicinal Chemistry, vol. 100, 2024, ISSN: 0968-0896.
@article{Yang2024,
title = {Discovery of thiophen-2-ylmethylene bis-dimedone derivatives as novel WRN inhibitors for treating cancers with microsatellite instability},
author = {Hwasun Yang and Miso Kang and Seonyeong Jang and Soo Yeon Baek and Jiwon Kim and Gyeong Un Kim and Dongwoo Kim and Junsu Ha and Jong Seung Kim and Cheulhee Jung and Nam-Jung Kim and Sung-Yup Cho and Woong-Hee Shin and Juyong Lee and Junsu Ko and Ansoo Lee and Gyochang Keum and Sanghee Lee and Taek Kang},
doi = {10.1016/j.bmc.2024.117588},
issn = {0968-0896},
year = {2024},
date = {2024-02-00},
urldate = {2024-02-00},
journal = {Bioorganic & Medicinal Chemistry},
volume = {100},
publisher = {Elsevier BV},
abstract = {Microsatellite instability (MSI) is a hypermutable condition caused by DNA mismatch repair system defects, contributing to the development of various cancer types. Recent research has identified Werner syndrome ATP-dependent helicase (WRN) as a promising synthetic lethal target for MSI cancers. Herein, we report the first discovery of thiophen-2-ylmethylene bis-dimedone derivatives as novel WRN inhibitors for MSI cancer therapy. Initial computational analysis and biological evaluation identified a new scaffold for a WRN inhibitor. Subsequent SAR study led to the discovery of a highly potent WRN inhibitor. Furthermore, we demonstrated that the optimal compound induced DNA damage and apoptotic cell death in MSI cancer cells by inhibiting WRN. This study provides a new pharmacophore for WRN inhibitors, emphasizing their therapeutic potential for MSI cancers.},
keywords = {},
pubstate = {published},
tppubtype = {article}
}
2023
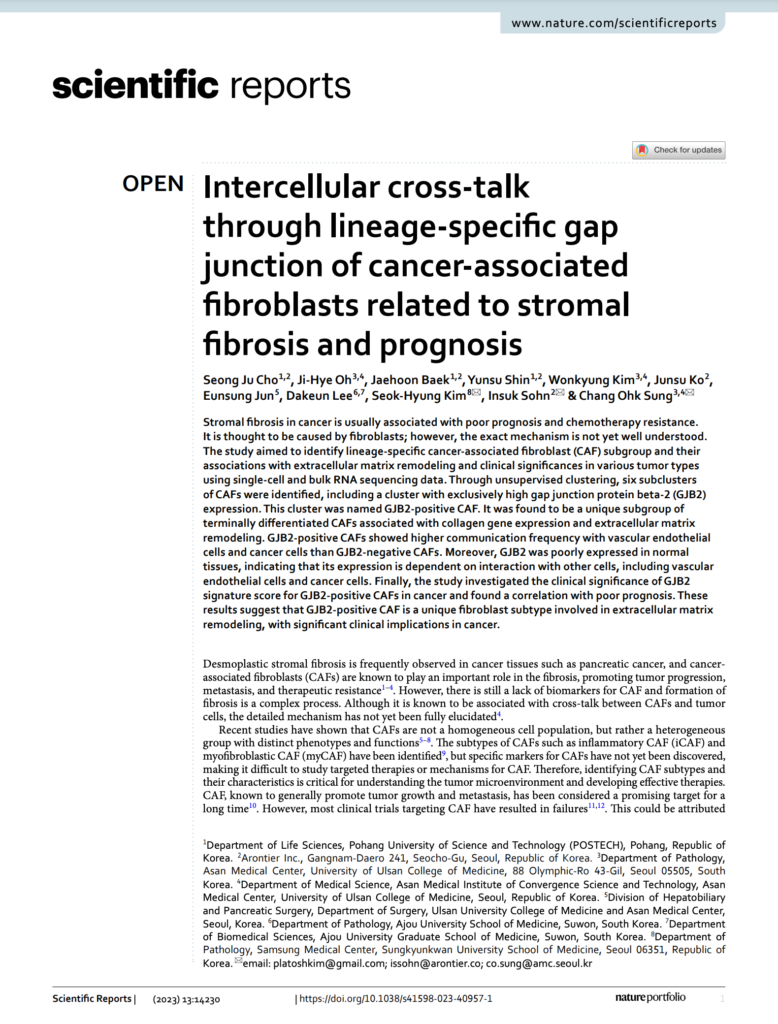
Cho S J; Oh J; Baek J; Shin Y; Kim W; Ko J; Jun E; Lee D; Kim S; Sohn I; Sung C O
Intercellular cross-talk through lineage-specific gap junction of cancer-associated fibroblasts related to stromal fibrosis and prognosis Journal Article
In: Sci Rep, vol. 13, no. 1, 2023, ISSN: 2045-2322.
@article{Cho2023,
title = {Intercellular cross-talk through lineage-specific gap junction of cancer-associated fibroblasts related to stromal fibrosis and prognosis},
author = {Seong Ju Cho and Ji-Hye Oh and Jaehoon Baek and Yunsu Shin and Wonkyung Kim and Junsu Ko and Eunsung Jun and Dakeun Lee and Seok-Hyung Kim and Insuk Sohn and Chang Ohk Sung},
doi = {10.1038/s41598-023-40957-1},
issn = {2045-2322},
year = {2023},
date = {2023-12-00},
urldate = {2023-12-00},
journal = {Sci Rep},
volume = {13},
number = {1},
publisher = {Springer Science and Business Media LLC},
abstract = {Stromal fibrosis in cancer is usually associated with poor prognosis and chemotherapy resistance. It is thought to be caused by fibroblasts; however, the exact mechanism is not yet well understood. The study aimed to identify lineage-specific cancer-associated fibroblast (CAF) subgroup and their associations with extracellular matrix remodeling and clinical significances in various tumor types using single-cell and bulk RNA sequencing data. Through unsupervised clustering, six subclusters of CAFs were identified, including a cluster with exclusively high gap junction protein beta-2 (GJB2) expression. This cluster was named GJB2-positive CAF. It was found to be a unique subgroup of terminally differentiated CAFs associated with collagen gene expression and extracellular matrix remodeling. GJB2-positive CAFs showed higher communication frequency with vascular endothelial cells and cancer cells than GJB2-negative CAFs. Moreover, GJB2 was poorly expressed in normal tissues, indicating that its expression is dependent on interaction with other cells, including vascular endothelial cells and cancer cells. Finally, the study investigated the clinical significance of GJB2 signature score for GJB2-positive CAFs in cancer and found a correlation with poor prognosis. These results suggest that GJB2-positive CAF is a unique fibroblast subtype involved in extracellular matrix remodeling, with significant clinical implications in cancer.},
keywords = {},
pubstate = {published},
tppubtype = {article}
}
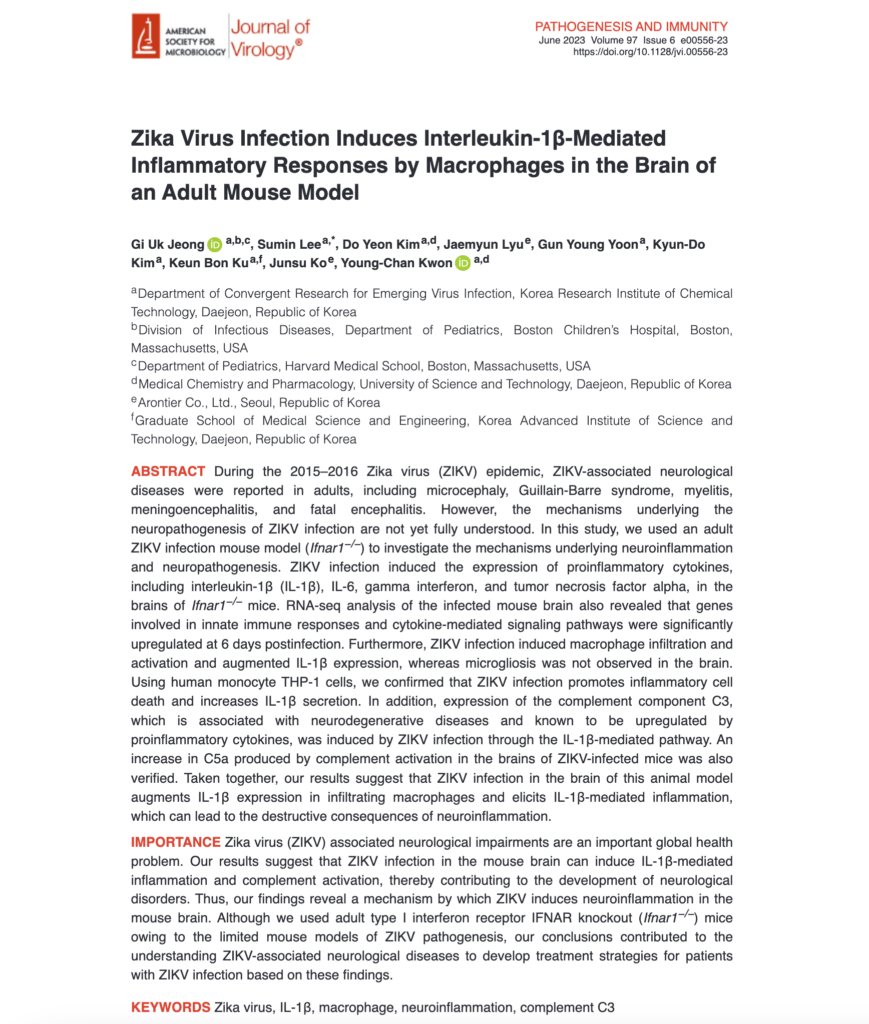
Jeong G U; Lee S; Kim D Y; Lyu J; Yoon G Y; Kim K; Ku K B; Ko J; Kwon Y
Zika Virus Infection Induces Interleukin-1β-Mediated Inflammatory Responses by Macrophages in the Brain of an Adult Mouse Model Journal Article
In: J Virol, vol. 97, no. 6, 2023, ISSN: 1098-5514.
@article{Jeong2023,
title = {Zika Virus Infection Induces Interleukin-1β-Mediated Inflammatory Responses by Macrophages in the Brain of an Adult Mouse Model},
author = {Gi Uk Jeong and Sumin Lee and Do Yeon Kim and Jaemyun Lyu and Gun Young Yoon and Kyun-Do Kim and Keun Bon Ku and Junsu Ko and Young-Chan Kwon},
editor = {Felicia Goodrum},
doi = {10.1128/jvi.00556-23},
issn = {1098-5514},
year = {2023},
date = {2023-06-29},
urldate = {2023-06-29},
journal = {J Virol},
volume = {97},
number = {6},
publisher = {American Society for Microbiology},
abstract = {During the 2015–2016 Zika virus (ZIKV) epidemic, ZIKV-associated neurological diseases were reported in adults, including microcephaly, Guillain-Barre syndrome, myelitis, meningoencephalitis, and fatal encephalitis. However, the mechanisms underlying the neuropathogenesis of ZIKV infection are not yet fully understood. In this study, we used an adult ZIKV infection mouse model (Ifnar1−/−) to investigate the mechanisms underlying neuroinflammation and neuropathogenesis. ZIKV infection induced the expression of proinflammatory cytokines, including interleukin-1β (IL-1β), IL-6, gamma interferon, and tumor necrosis factor alpha, in the brains of Ifnar1−/− mice. RNA-seq analysis of the infected mouse brain also revealed that genes involved in innate immune responses and cytokine-mediated signaling pathways were significantly upregulated at 6 days postinfection. Furthermore, ZIKV infection induced macrophage infiltration and activation and augmented IL-1β expression, whereas microgliosis was not observed in the brain. Using human monocyte THP-1 cells, we confirmed that ZIKV infection promotes inflammatory cell death and increases IL-1β secretion. In addition, expression of the complement component C3, which is associated with neurodegenerative diseases and known to be upregulated by proinflammatory cytokines, was induced by ZIKV infection through the IL-1β-mediated pathway. An increase in C5a produced by complement activation in the brains of ZIKV-infected mice was also verified. Taken together, our results suggest that ZIKV infection in the brain of this animal model augments IL-1β expression in infiltrating macrophages and elicits IL-1β-mediated inflammation, which can lead to the destructive consequences of neuroinflammation.
IMPORTANCE Zika virus (ZIKV) associated neurological impairments are an important global health problem. Our results suggest that ZIKV infection in the mouse brain can induce IL-1β-mediated inflammation and complement activation, thereby contributing to the development of neurological disorders. Thus, our findings reveal a mechanism by which ZIKV induces neuroinflammation in the mouse brain. Although we used adult type I interferon receptor IFNAR knockout (Ifnar1−/−) mice owing to the limited mouse models of ZIKV pathogenesis, our conclusions contributed to the understanding ZIKV-associated neurological diseases to develop treatment strategies for patients with ZIKV infection based on these findings.},
keywords = {},
pubstate = {published},
tppubtype = {article}
}
IMPORTANCE Zika virus (ZIKV) associated neurological impairments are an important global health problem. Our results suggest that ZIKV infection in the mouse brain can induce IL-1β-mediated inflammation and complement activation, thereby contributing to the development of neurological disorders. Thus, our findings reveal a mechanism by which ZIKV induces neuroinflammation in the mouse brain. Although we used adult type I interferon receptor IFNAR knockout (Ifnar1−/−) mice owing to the limited mouse models of ZIKV pathogenesis, our conclusions contributed to the understanding ZIKV-associated neurological diseases to develop treatment strategies for patients with ZIKV infection based on these findings.
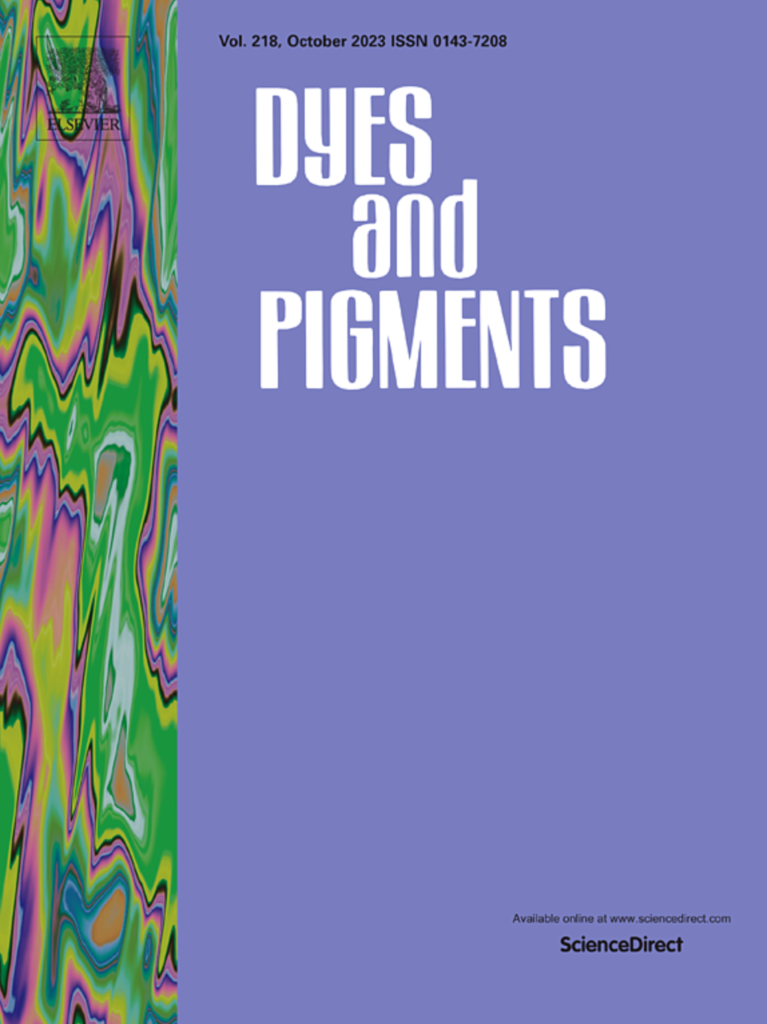
Lee J; Choi S; Aslam A S; Lim W; Lee J; Ko J; Ryu C H; Lee K M; Jung Y M; Yoo H S; Park J H; Lee S; Choi J; Kim E; Park J
N4-phenylquinazoline-4,6-diamine as a tunable fluorescent scaffold for the development of fluorescent probes Journal Article
In: Dyes and Pigments, vol. 210, 2023, ISSN: 0143-7208.
@article{Lee2023,
title = {N4-phenylquinazoline-4,6-diamine as a tunable fluorescent scaffold for the development of fluorescent probes},
author = {Jaeseok Lee and Sang-Kee Choi and Adil S. Aslam and Woojeong Lim and Juyong Lee and Junsu Ko and Chan Hee Ryu and Kang Mun Lee and Young Mee Jung and Hyuk Sang Yoo and Ju Hyun Park and Sanghee Lee and Junwon Choi and Eunha Kim and Jongmin Park},
doi = {10.1016/j.dyepig.2022.110987},
issn = {0143-7208},
year = {2023},
date = {2023-02-00},
urldate = {2023-02-00},
journal = {Dyes and Pigments},
volume = {210},
publisher = {Elsevier BV},
abstract = {Fluorescent molecules have been used for various applications in biological system monitoring. Considering the complexity of cellular systems, there is a huge demand for diverse fluorescent organic molecular scaffolds. However, only a limited number of fluorescent scaffolds have been reported due to the lack of a rational design strategy. We serendipitously discovered N4-phenylquinazoline-4,6-diamine as a fluorescent scaffold with turn-on characteristics. To improve the photophysical property of the initial fluorescence molecule, we synthesized derivatives of the N4-phenylquinazoline-4,6-diamine and found systematic correlation between electronic density of the phenyl substituent of the scaffold and fluorescence intensity. Through tuning the photophysical property of the scaffold and a rational design strategy, we developed N4-dichlorophenylquinazoline-4,6-diamine as a potential fluorophore for various biological applications. To prove the value of the developed fluorescent scaffold, we devised formaldehyde (FA) fluorescent sensor by tailoring amine on 6-position of N4-dichlorophenylquinazoline-4,6-diamine to employ a 2-aza-Cope rearrangement as the molecular detection mechanism with double-digit nanomolar detection limits. FA level in live HeLa cells were successfully visualized with the probe, exhibiting that N4-dichlorophenylquinazoline-4,6-diamine can serve as a useful molecular scaffold for offering various fluorescent sensors toward the investigation of physiological and pathological processes in live cells.},
keywords = {},
pubstate = {published},
tppubtype = {article}
}
2022
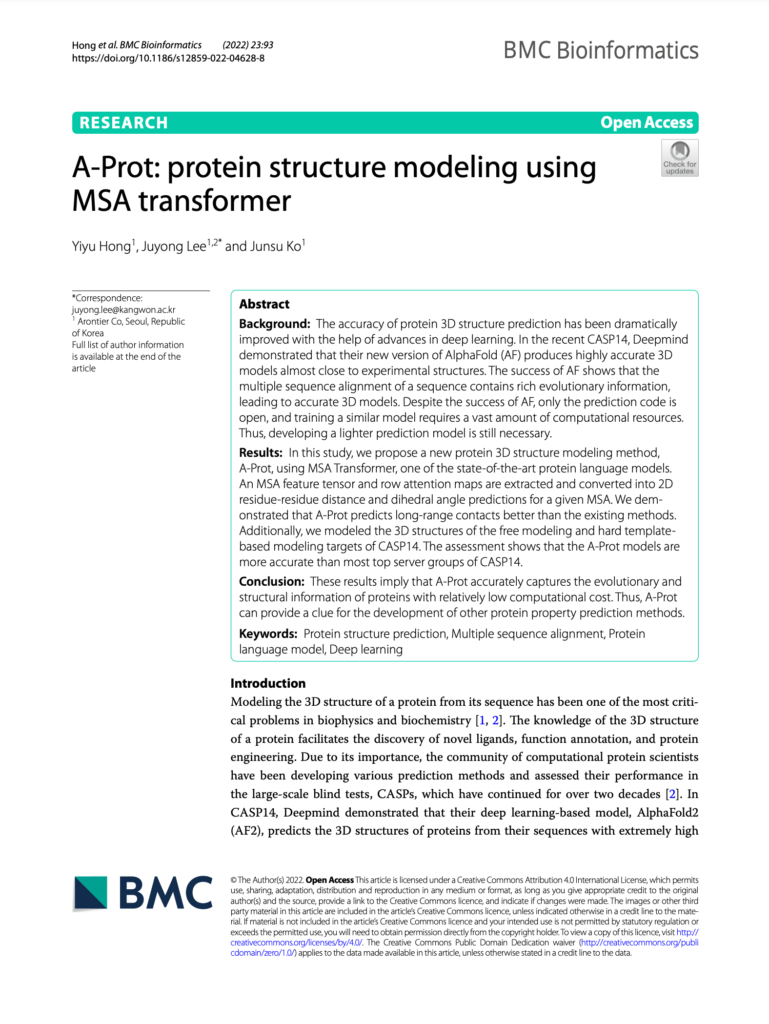
Hong Y; Lee J; Ko J
A-Prot: protein structure modeling using MSA transformer Journal Article
In: BMC Bioinformatics, vol. 23, no. 1, 2022, ISSN: 1471-2105.
@article{Hong2022,
title = {A-Prot: protein structure modeling using MSA transformer},
author = {Yiyu Hong and Juyong Lee and Junsu Ko},
doi = {10.1186/s12859-022-04628-8},
issn = {1471-2105},
year = {2022},
date = {2022-12-00},
urldate = {2022-12-00},
journal = {BMC Bioinformatics},
volume = {23},
number = {1},
publisher = {Springer Science and Business Media LLC},
abstract = {Background: The accuracy of protein 3D structure prediction has been dramatically improved with the help of advances in deep learning. In the recent CASP14, Deepmind demonstrated that their new version of AlphaFold (AF) produces highly accurate 3D models almost close to experimental structures. The success of AF shows that the multiple sequence alignment of a sequence contains rich evolutionary information, leading to accurate 3D models. Despite the success of AF, only the prediction code is open, and training a similar model requires a vast amount of computational resources. Thus, developing a lighter prediction model is still necessary.
Results: In this study, we propose a new protein 3D structure modeling method, A-Prot, using MSA Transformer, one of the state-of-the-art protein language models. An MSA feature tensor and row attention maps are extracted and converted into 2D residue-residue distance and dihedral angle redictions for a given MSA. We demonstrated that A-Prot predicts long-range contacts better than the existing methods.
Additionally, we modeled the 3D structures of the free modeling and hard templatebased modeling targets of CASP14. The assessment shows that the A-Prot models are more accurate than most top server groups of CASP14.
Conclusion: These results imply that A-Prot accurately captures the evolutionary and structural information of proteins with relatively low computational cost. Thus, A-Prot can provide a clue for the development of other protein property prediction methods. },
keywords = {},
pubstate = {published},
tppubtype = {article}
}
Results: In this study, we propose a new protein 3D structure modeling method, A-Prot, using MSA Transformer, one of the state-of-the-art protein language models. An MSA feature tensor and row attention maps are extracted and converted into 2D residue-residue distance and dihedral angle redictions for a given MSA. We demonstrated that A-Prot predicts long-range contacts better than the existing methods.
Additionally, we modeled the 3D structures of the free modeling and hard templatebased modeling targets of CASP14. The assessment shows that the A-Prot models are more accurate than most top server groups of CASP14.
Conclusion: These results imply that A-Prot accurately captures the evolutionary and structural information of proteins with relatively low computational cost. Thus, A-Prot can provide a clue for the development of other protein property prediction methods.
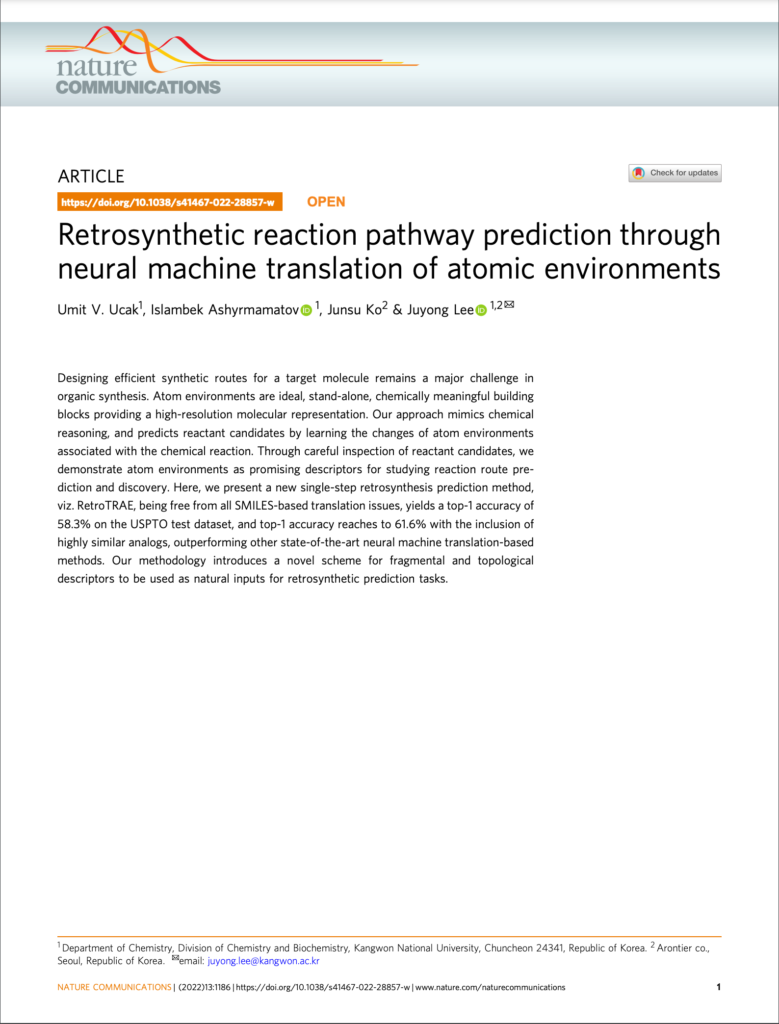
Ucak U V; Ashyrmamatov I; Ko J; Lee J
Retrosynthetic reaction pathway prediction through neural machine translation of atomic environments Journal Article
In: Nat Commun, vol. 13, no. 1, 2022, ISSN: 2041-1723.
@article{Ucak2022b,
title = {Retrosynthetic reaction pathway prediction through neural machine translation of atomic environments},
author = {Umit V. Ucak and Islambek Ashyrmamatov and Junsu Ko and Juyong Lee},
doi = {10.1038/s41467-022-28857-w},
issn = {2041-1723},
year = {2022},
date = {2022-12-00},
urldate = {2022-12-00},
journal = {Nat Commun},
volume = {13},
number = {1},
publisher = {Springer Science and Business Media LLC},
abstract = {Designing efficient synthetic routes for a target molecule remains a major challenge in organic synthesis. Atom environments are ideal, stand-alone, chemically meaningful building blocks providing a high-resolution molecular representation. Our approach mimics chemical reasoning, and predicts reactant candidates by learning the changes of atom environments associated with the chemical reaction. Through careful inspection of reactant candidates, we demonstrate atom environments as promising descriptors for studying reaction route prediction and discovery. Here, we present a new single-step retrosynthesis prediction method, viz. RetroTRAE, being free from all SMILES-based translation issues, yields a top-1 accuracy of 58.3% on the USPTO test dataset, and top-1 accuracy reaches to 61.6% with the inclusion of highly similar analogs, outperforming other state-of-the-art neural machine translation-based methods. Our methodology introduces a novel scheme for fragmental and topological descriptors to be used as natural inputs for retrosynthetic prediction tasks.},
keywords = {},
pubstate = {published},
tppubtype = {article}
}
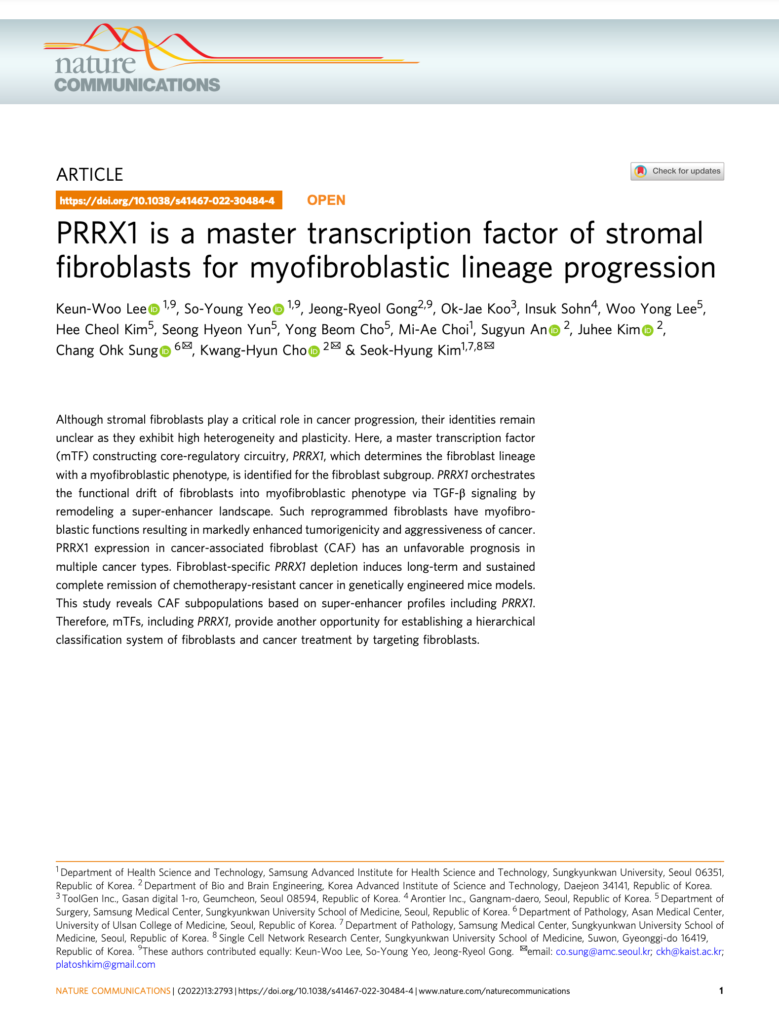
Lee K; Yeo S; Gong J; Koo O; Sohn I; Lee W Y; Kim H C; Yun S H; Cho Y B; Choi M; An S; Kim J; Sung C O; Cho K; Kim S
PRRX1 is a master transcription factor of stromal fibroblasts for myofibroblastic lineage progression Journal Article
In: Nat Commun, vol. 13, no. 1, 2022, ISSN: 2041-1723.
@article{Lee2022,
title = {PRRX1 is a master transcription factor of stromal fibroblasts for myofibroblastic lineage progression},
author = {Keun-Woo Lee and So-Young Yeo and Jeong-Ryeol Gong and Ok-Jae Koo and Insuk Sohn and Woo Yong Lee and Hee Cheol Kim and Seong Hyeon Yun and Yong Beom Cho and Mi-Ae Choi and Sugyun An and Juhee Kim and Chang Ohk Sung and Kwang-Hyun Cho and Seok-Hyung Kim},
doi = {10.1038/s41467-022-30484-4},
issn = {2041-1723},
year = {2022},
date = {2022-12-00},
urldate = {2022-12-00},
journal = {Nat Commun},
volume = {13},
number = {1},
publisher = {Springer Science and Business Media LLC},
abstract = {Although stromal fibroblasts play a critical role in cancer progression, their identities remain unclear as they exhibit high heterogeneity and plasticity. Here, a master transcription factor (mTF) constructing core-regulatory circuitry, PRRX1, which determines the fibroblast lineage with a myofibroblastic phenotype, is identified for the fibroblast subgroup. PRRX1 orchestrates the functional drift of fibroblasts into myofibroblastic phenotype via TGF-β signaling by remodeling a super-enhancer landscape. Such reprogrammed fibroblasts have myofibroblastic functions resulting in markedly enhanced tumorigenicity and aggressiveness of cancer. PRRX1 expression in cancer-associated fibroblast (CAF) has an unfavorable prognosis in multiple cancer types. Fibroblast-specific PRRX1 depletion induces long-term and sustained complete remission of chemotherapy-resistant cancer in genetically engineered mice models. This study reveals CAF subpopulations based on super-enhancer profiles including PRRX1. Therefore, mTFs, including PRRX1, provide another opportunity for establishing a hierarchical classification system of fibroblasts and cancer treatment by targeting fibroblasts.},
keywords = {},
pubstate = {published},
tppubtype = {article}
}
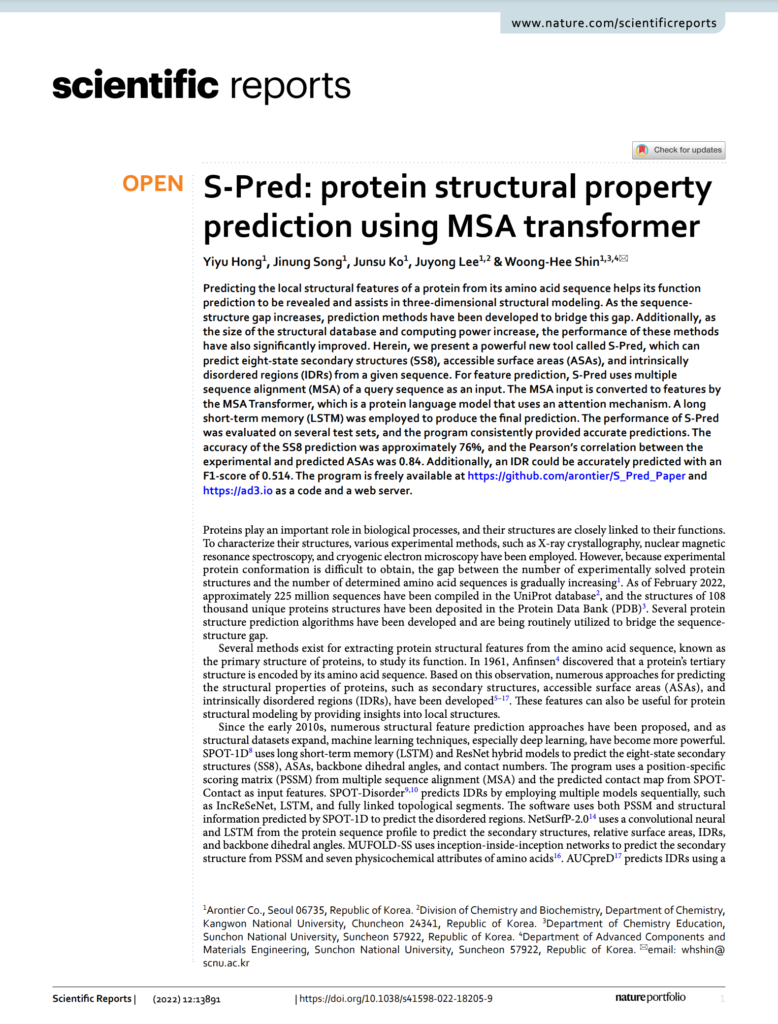
Hong Y; Song J; Ko J; Lee J; Shin W
S-Pred: protein structural property prediction using MSA transformer Journal Article
In: Sci Rep, vol. 12, no. 1, 2022, ISSN: 2045-2322.
@article{Hong2022b,
title = {S-Pred: protein structural property prediction using MSA transformer},
author = {Yiyu Hong and Jinung Song and Junsu Ko and Juyong Lee and Woong-Hee Shin},
doi = {10.1038/s41598-022-18205-9},
issn = {2045-2322},
year = {2022},
date = {2022-12-00},
urldate = {2022-12-00},
journal = {Sci Rep},
volume = {12},
number = {1},
publisher = {Springer Science and Business Media LLC},
abstract = {Predicting the local structural features of a protein from its amino acid sequence helps its function prediction to be revealed and assists in three-dimensional structural modeling. As the sequence-structure gap increases, prediction methods have been developed to bridge this gap. Additionally, as the size of the structural database and computing power increase, the performance of these methods have also significantly improved. Herein, we present a powerful new tool called S-Pred, which can predict eight-state secondary structures (SS8), accessible surface areas (ASAs), and intrinsically disordered regions (IDRs) from a given sequence. For feature prediction, S-Pred uses multiple sequence alignment (MSA) of a query sequence as an input. The MSA input is converted to features by the MSA Transformer, which is a protein language model that uses an attention mechanism. A long short-term memory (LSTM) was employed to produce the final prediction. The performance of S-Pred was evaluated on several test sets, and the program consistently provided accurate predictions. The accuracy of the SS8 prediction was approximately 76%, and the Pearson’s correlation between the experimental and predicted ASAs was 0.84. Additionally, an IDR could be accurately predicted with an F1-score of 0.514. The program is freely available at https://github.com/arontier/S_Pred_Paper and https://ad3.io as a code and a web server.},
keywords = {},
pubstate = {published},
tppubtype = {article}
}
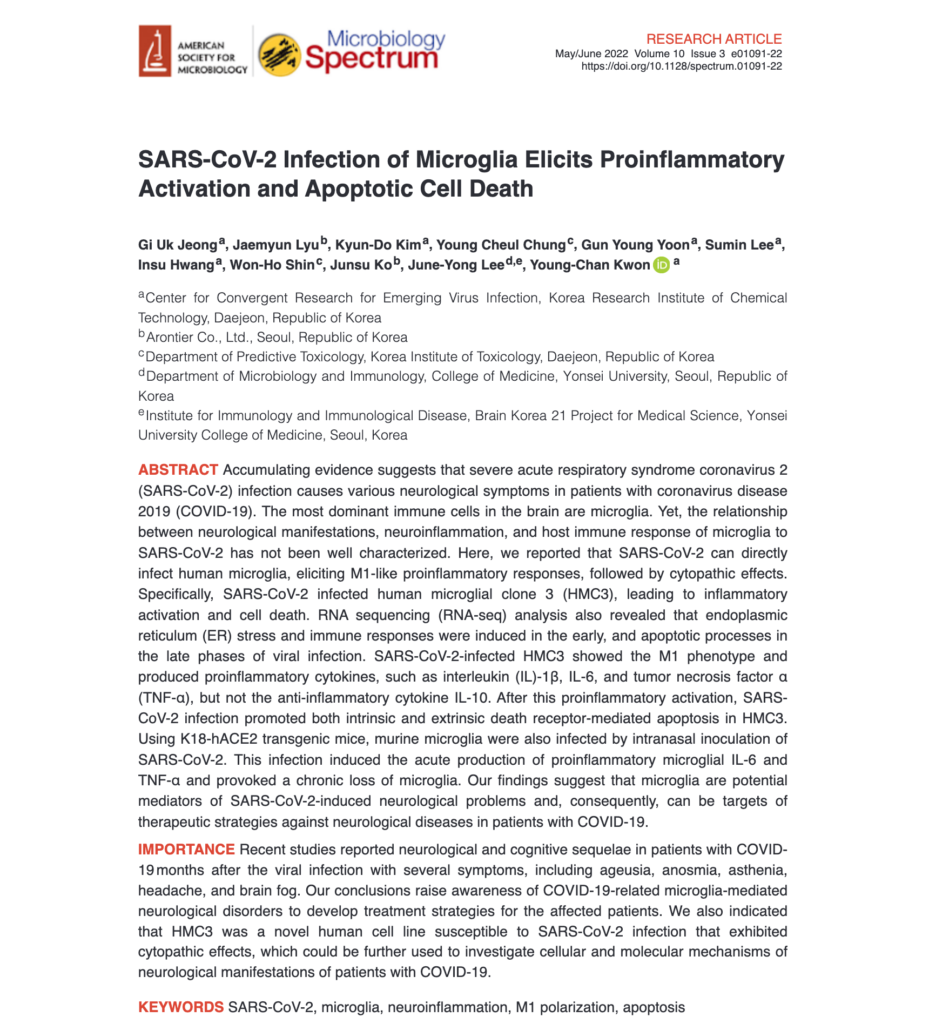
Jeong G U; Lyu J; Kim K; Chung Y C; Yoon G Y; Lee S; Hwang I; Shin W; Ko J; Lee J; Kwon Y
SARS-CoV-2 Infection of Microglia Elicits Proinflammatory Activation and Apoptotic Cell Death Journal Article
In: Microbiol Spectr, vol. 10, no. 3, 2022, ISSN: 2165-0497.
@article{Jeong2022,
title = {SARS-CoV-2 Infection of Microglia Elicits Proinflammatory Activation and Apoptotic Cell Death},
author = {Gi Uk Jeong and Jaemyun Lyu and Kyun-Do Kim and Young Cheul Chung and Gun Young Yoon and Sumin Lee and Insu Hwang and Won-Ho Shin and Junsu Ko and June-Yong Lee and Young-Chan Kwon},
editor = {Ujjwal Neogi},
doi = {10.1128/spectrum.01091-22},
issn = {2165-0497},
year = {2022},
date = {2022-06-29},
urldate = {2022-06-29},
journal = {Microbiol Spectr},
volume = {10},
number = {3},
publisher = {American Society for Microbiology},
abstract = {Accumulating evidence suggests that severe acute respiratory syndrome coronavirus 2 (SARS-CoV-2) infection causes various neurological symptoms in patients with coronavirus disease 2019 (COVID-19). The most dominant immune cells in the brain are microglia. Yet, the relationship between neurological manifestations, neuroinflammation, and host immune response of microglia to SARS-CoV-2 has not been well characterized. Here, we reported that SARS-CoV-2 can directly infect human microglia, eliciting M1-like proinflammatory responses, followed by cytopathic effects. Specifically, SARS-CoV-2 infected human microglial clone 3 (HMC3), leading to inflammatory activation and cell death. RNA sequencing (RNA-seq) analysis also revealed that endoplasmic reticulum (ER) stress and immune responses were induced in the early, and apoptotic processes in the late phases of viral infection. SARS-CoV-2-infected HMC3 showed the M1 phenotype and produced proinflammatory cytokines, such as interleukin (IL)-1β, IL-6, and tumor necrosis factor α (TNF-α), but not the anti-inflammatory cytokine IL-10. After this proinflammatory activation, SARS-CoV-2 infection promoted both intrinsic and extrinsic death receptor-mediated apoptosis in HMC3. Using K18-hACE2 transgenic mice, murine microglia were also infected by intranasal inoculation of SARS-CoV-2. This infection induced the acute production of proinflammatory microglial IL-6 and TNF-α and provoked a chronic loss of microglia. Our findings suggest that microglia are potential mediators of SARS-CoV-2-induced neurological problems and, consequently, can be targets of therapeutic strategies against neurological diseases in patients with COVID-19.
IMPORTANCE Recent studies reported neurological and cognitive sequelae in patients with COVID-19 months after the viral infection with several symptoms, including ageusia, anosmia, asthenia, headache, and brain fog. Our conclusions raise awareness of COVID-19-related microglia-mediated neurological disorders to develop treatment strategies for the affected patients. We also indicated that HMC3 was a novel human cell line susceptible to SARS-CoV-2 infection that exhibited cytopathic effects, which could be further used to investigate cellular and molecular mechanisms of neurological manifestations of patients with COVID-19.},
keywords = {},
pubstate = {published},
tppubtype = {article}
}
IMPORTANCE Recent studies reported neurological and cognitive sequelae in patients with COVID-19 months after the viral infection with several symptoms, including ageusia, anosmia, asthenia, headache, and brain fog. Our conclusions raise awareness of COVID-19-related microglia-mediated neurological disorders to develop treatment strategies for the affected patients. We also indicated that HMC3 was a novel human cell line susceptible to SARS-CoV-2 infection that exhibited cytopathic effects, which could be further used to investigate cellular and molecular mechanisms of neurological manifestations of patients with COVID-19.
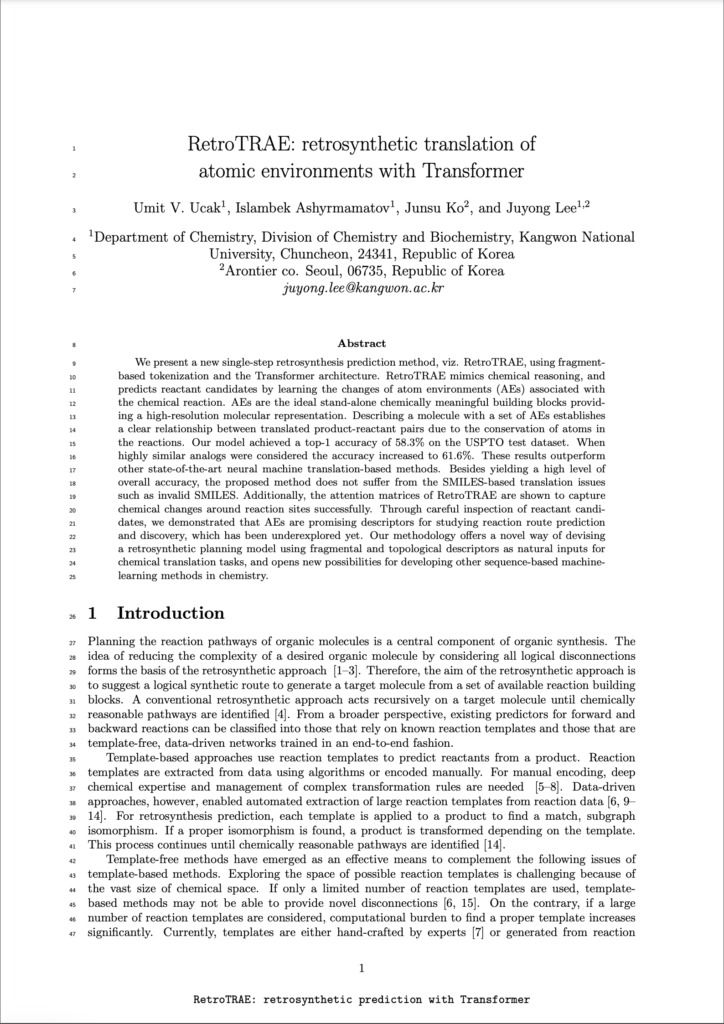
Ucak U V; Ashyrmamatov I; Ko J; Lee J
RetroTRAE: retrosynthetic translation of atomic environments with Transformer Unpublished
2022.
@unpublished{Ucak2022,
title = {RetroTRAE: retrosynthetic translation of atomic environments with Transformer},
author = {Umit Volkan Ucak and Islambek Ashyrmamatov and Junsu Ko and Juyong Lee},
url = {https://chemrxiv.org/engage/chemrxiv/article-details/61f177d7e59d8731714a6a9d},
doi = {10.26434/chemrxiv-2021-9g274-v3},
year = {2022},
date = {2022-01-27},
urldate = {2022-01-27},
publisher = {American Chemical Society (ACS)},
abstract = {We present a new single-step retrosynthesis prediction method, viz. RetroTRAE, using fragment-based tokenization and the Transformer architecture. RetroTRAE mimics chemical reasoning, and predicts reactant candidates by learning the changes of atom environments (AEs) associated with the chemical reaction. AEs are the ideal stand-alone chemically meaningful building blocks providing a high-resolution molecular representation. Describing a molecule with a set of AEs establishes a clear relationship between translated product-reactant pairs due to the conservation of atoms in the reactions. Our model achieved a top-1 accuracy of 58.3% on the USPTO test dataset. When highly similar analogs were considered the accuracy increased to 61.6%. These results outperform other state-of-the-art neural machine translation-based methods. Besides yielding a high level of overall accuracy, the proposed method does not suffer from the SMILES-based translation issues such as invalid SMILES. Additionally, the attention matrices of RetroTRAE are shown to capture chemical changes around reaction sites successfully. Through careful inspection of reactant candidates, we demonstrated that AEs are promising descriptors for studying reaction route prediction and discovery, which has been underexplored yet. Our methodology offers a novel way of devising a retrosynthetic planning model using fragmental and topological descriptors as natural inputs for chemical translation tasks, and opens new possibilities for developing other sequence-based machine-learning methods in chemistry.},
keywords = {},
pubstate = {published},
tppubtype = {unpublished}
}
2021
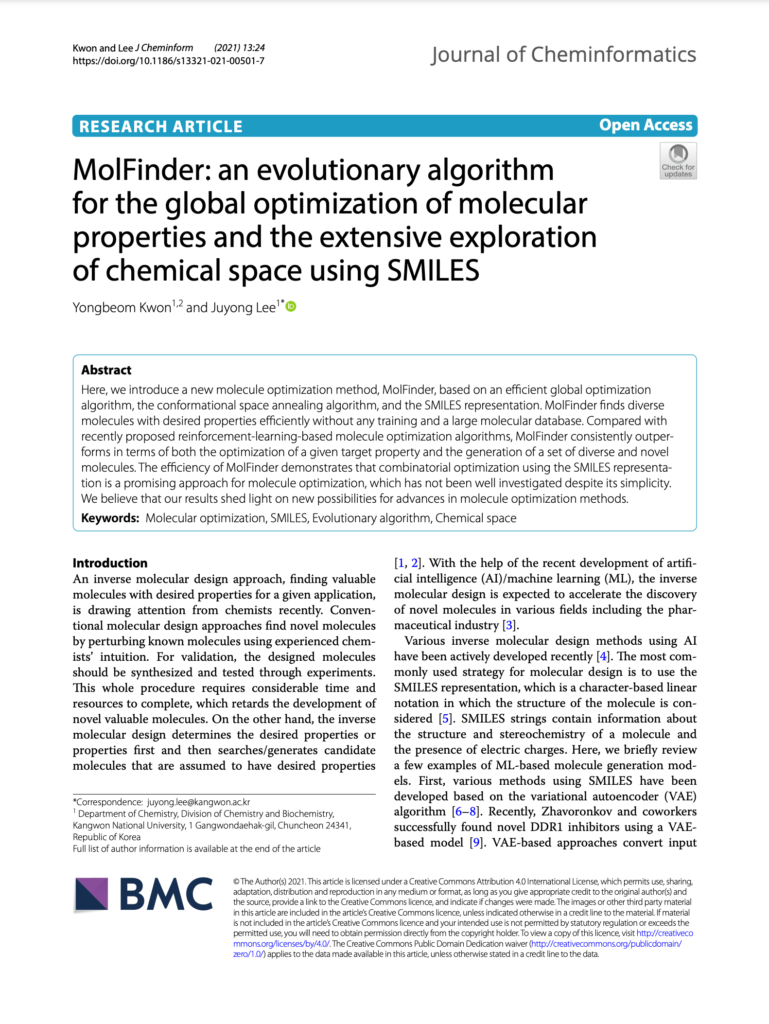
Kwon Y; Lee J
In: J Cheminform, vol. 13, no. 1, 2021, ISSN: 1758-2946.
@article{Kwon2021,
title = {MolFinder: an evolutionary algorithm for the global optimization of molecular properties and the extensive exploration of chemical space using SMILES},
author = {Yongbeom Kwon and Juyong Lee},
doi = {10.1186/s13321-021-00501-7},
issn = {1758-2946},
year = {2021},
date = {2021-12-00},
urldate = {2021-12-00},
journal = {J Cheminform},
volume = {13},
number = {1},
publisher = {Springer Science and Business Media LLC},
abstract = {Here, we introduce a new molecule optimization method, MolFinder, based on an efficient global optimization algorithm, the conformational space annealing algorithm, and the SMILES representation. MolFinder finds diverse molecules with desired properties efficiently without any training and a large molecular database. Compared with recently proposed reinforcement-learning-based molecule optimization algorithms, MolFinder consistently outperforms in terms of both the optimization of a given target property and the generation of a set of diverse and novel molecules. The efficiency of MolFinder demonstrates that combinatorial optimization using the SMILES representation is a promising approach for molecule optimization, which has not been well investigated despite its simplicity. We believe that our results shed light on new possibilities for advances in molecule optimization methods.},
keywords = {},
pubstate = {published},
tppubtype = {article}
}
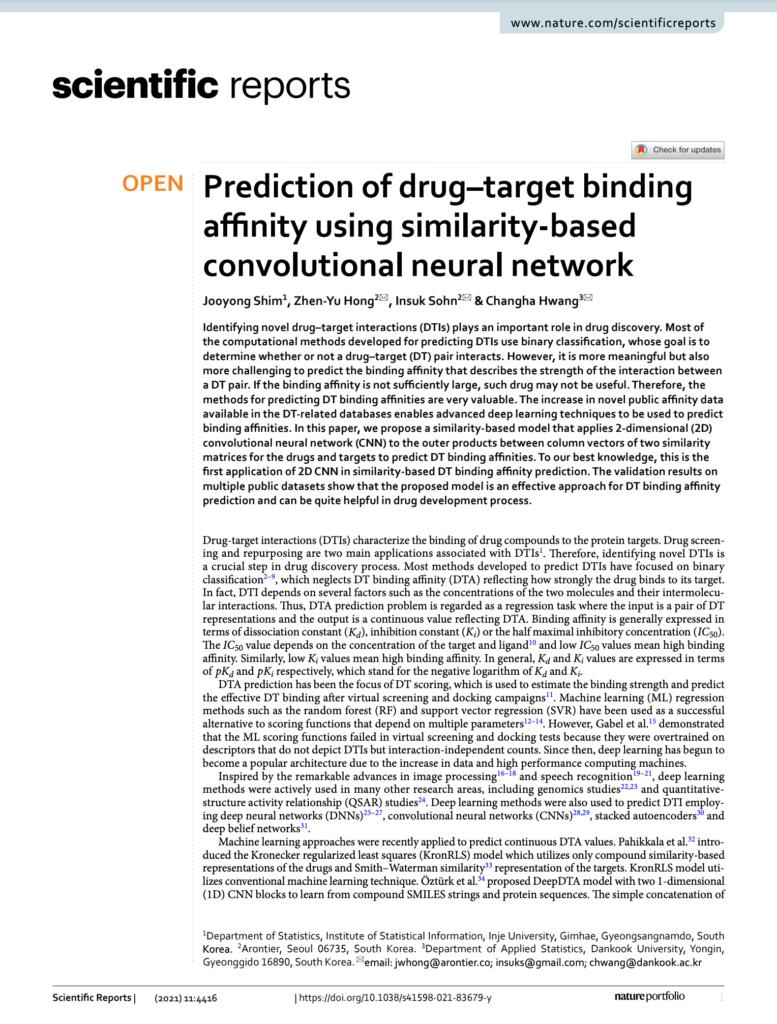
Shim J; Hong Z; Sohn I; Hwang C
Prediction of drug–target binding affinity using similarity-based convolutional neural network Journal Article
In: Sci Rep, vol. 11, no. 1, 2021, ISSN: 2045-2322.
@article{Shim2021,
title = {Prediction of drug–target binding affinity using similarity-based convolutional neural network},
author = {Jooyong Shim and Zhen-Yu Hong and Insuk Sohn and Changha Hwang},
doi = {10.1038/s41598-021-83679-y},
issn = {2045-2322},
year = {2021},
date = {2021-12-00},
urldate = {2021-12-00},
journal = {Sci Rep},
volume = {11},
number = {1},
publisher = {Springer Science and Business Media LLC},
abstract = {Identifying novel drug–target interactions (DTIs) plays an important role in drug discovery. Most of the computational methods developed for predicting DTIs use binary classification, whose goal is to determine whether or not a drug–target (DT) pair interacts. However, it is more meaningful but also more challenging to predict the binding affinity that describes the strength of the interaction between a DT pair. If the binding affinity is not sufficiently large, such drug may not be useful. Therefore, the methods for predicting DT binding affinities are very valuable. The increase in novel public affinity data available in the DT-related databases enables advanced deep learning techniques to be used to predict binding affinities. In this paper, we propose a similarity-based model that applies 2-dimensional (2D) convolutional neural network (CNN) to the outer products between column vectors of two similarity matrices for the drugs and targets to predict DT binding affinities. To our best knowledge, this is the first application of 2D CNN in similarity-based DT binding affinity prediction. The validation results on multiple public datasets show that the proposed model is an effective approach for DT binding affinity prediction and can be quite helpful in drug development process.},
keywords = {},
pubstate = {published},
tppubtype = {article}
}
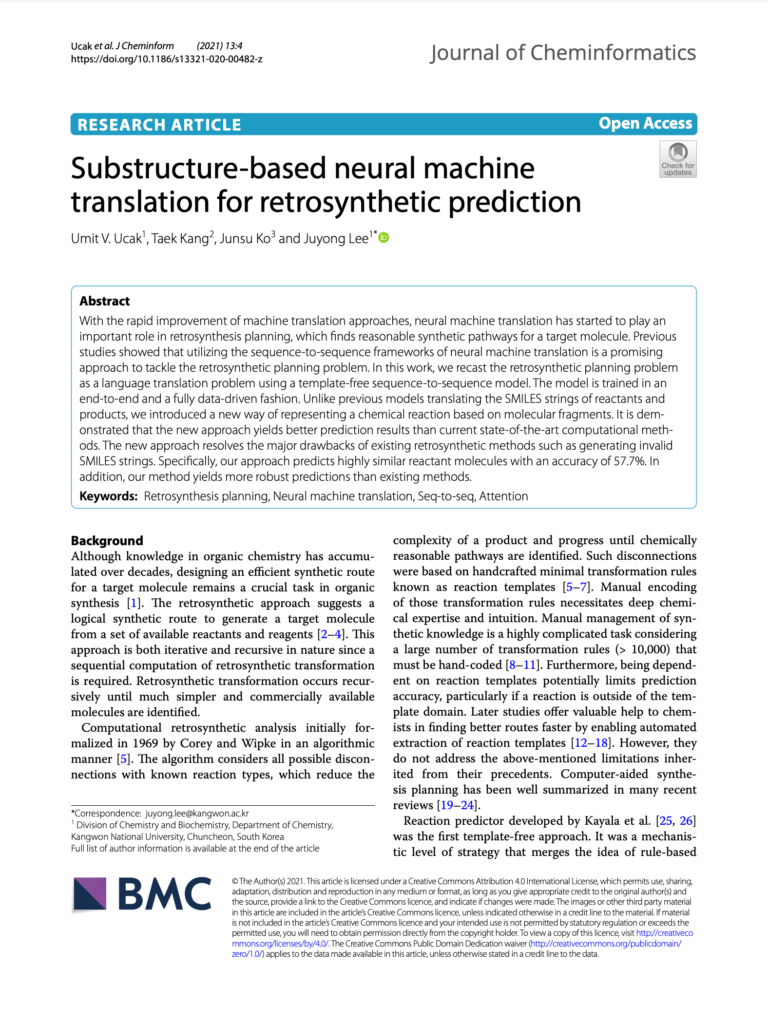
Ucak U V; Kang T; Ko J; Lee J
Substructure-based neural machine translation for retrosynthetic prediction Journal Article
In: J Cheminform, vol. 13, no. 1, 2021, ISSN: 1758-2946.
@article{Ucak2021,
title = {Substructure-based neural machine translation for retrosynthetic prediction},
author = {Umit V. Ucak and Taek Kang and Junsu Ko and Juyong Lee},
doi = {10.1186/s13321-020-00482-z},
issn = {1758-2946},
year = {2021},
date = {2021-12-00},
urldate = {2021-12-00},
journal = {J Cheminform},
volume = {13},
number = {1},
publisher = {Springer Science and Business Media LLC},
abstract = {With the rapid improvement of machine translation approaches, neural machine translation has started to play an important role in retrosynthesis planning, which finds reasonable synthetic pathways for a target molecule. Previous studies showed that utilizing the sequence-to-sequence frameworks of neural machine translation is a promising approach to tackle the retrosynthetic planning problem. In this work, we recast the retrosynthetic planning problem as a language translation problem using a template-free sequence-to-sequence model. The model is trained in an end-to-end and a fully data-driven fashion. Unlike previous models translating the SMILES strings of reactants and products, we introduced a new way of representing a chemical reaction based on molecular fragments. It is demonstrated that the new approach yields better prediction results than current state-of-the-art computational methods. The new approach resolves the major drawbacks of existing retrosynthetic methods such as generating invalid SMILES strings. Specifically, our approach predicts highly similar reactant molecules with an accuracy of 57.7%. In addition, our method yields more robust predictions than existing methods.},
keywords = {},
pubstate = {published},
tppubtype = {article}
}
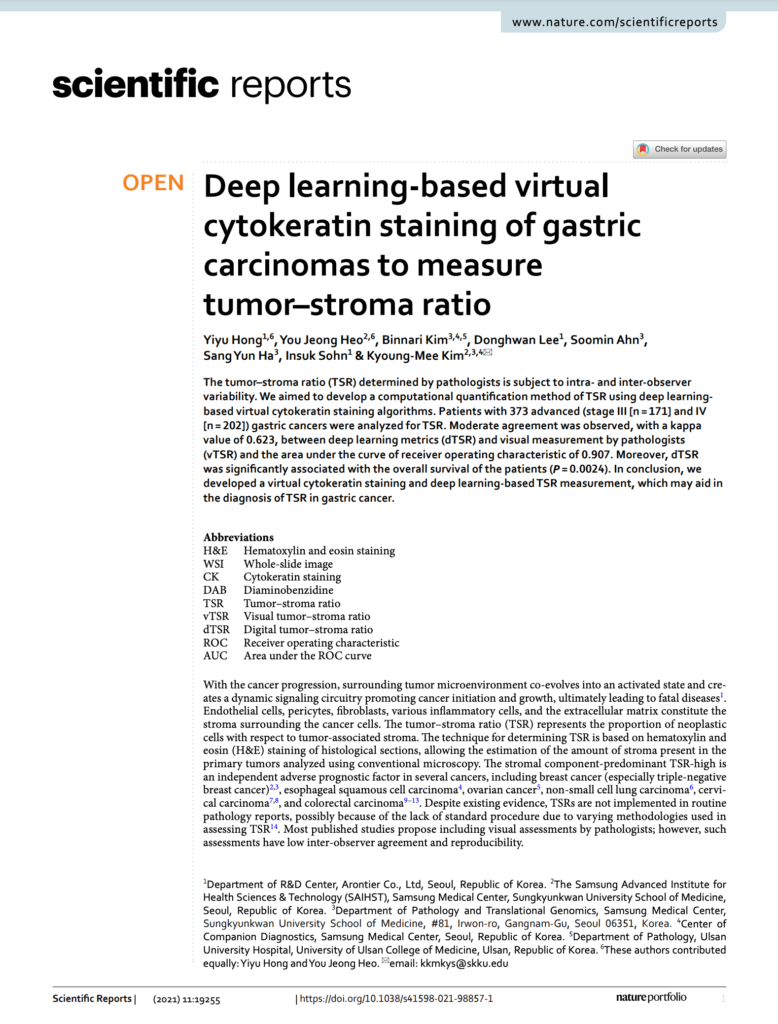
Hong Y; Heo Y J; Kim B; Lee D; Ahn S; Ha S Y; Sohn I; Kim K
Deep learning-based virtual cytokeratin staining of gastric carcinomas to measure tumor–stroma ratio Journal Article
In: Sci Rep, vol. 11, no. 1, 2021, ISSN: 2045-2322.
@article{Hong2021,
title = {Deep learning-based virtual cytokeratin staining of gastric carcinomas to measure tumor–stroma ratio},
author = {Yiyu Hong and You Jeong Heo and Binnari Kim and Donghwan Lee and Soomin Ahn and Sang Yun Ha and Insuk Sohn and Kyoung-Mee Kim},
doi = {10.1038/s41598-021-98857-1},
issn = {2045-2322},
year = {2021},
date = {2021-12-00},
urldate = {2021-12-00},
journal = {Sci Rep},
volume = {11},
number = {1},
publisher = {Springer Science and Business Media LLC},
abstract = {The tumor–stroma ratio (TSR) determined by pathologists is subject to intra- and inter-observer variability. We aimed to develop a computational quantification method of TSR using deep learning-based virtual cytokeratin staining algorithms. Patients with 373 advanced (stage III [n = 171] and IV [n = 202]) gastric cancers were analyzed for TSR. Moderate agreement was observed, with a kappa value of 0.623, between deep learning metrics (dTSR) and visual measurement by pathologists (vTSR) and the area under the curve of receiver operating characteristic of 0.907. Moreover, dTSR was significantly associated with the overall survival of the patients (P = 0.0024). In conclusion, we developed a virtual cytokeratin staining and deep learning-based TSR measurement, which may aid in the diagnosis of TSR in gastric cancer.},
keywords = {},
pubstate = {published},
tppubtype = {article}
}
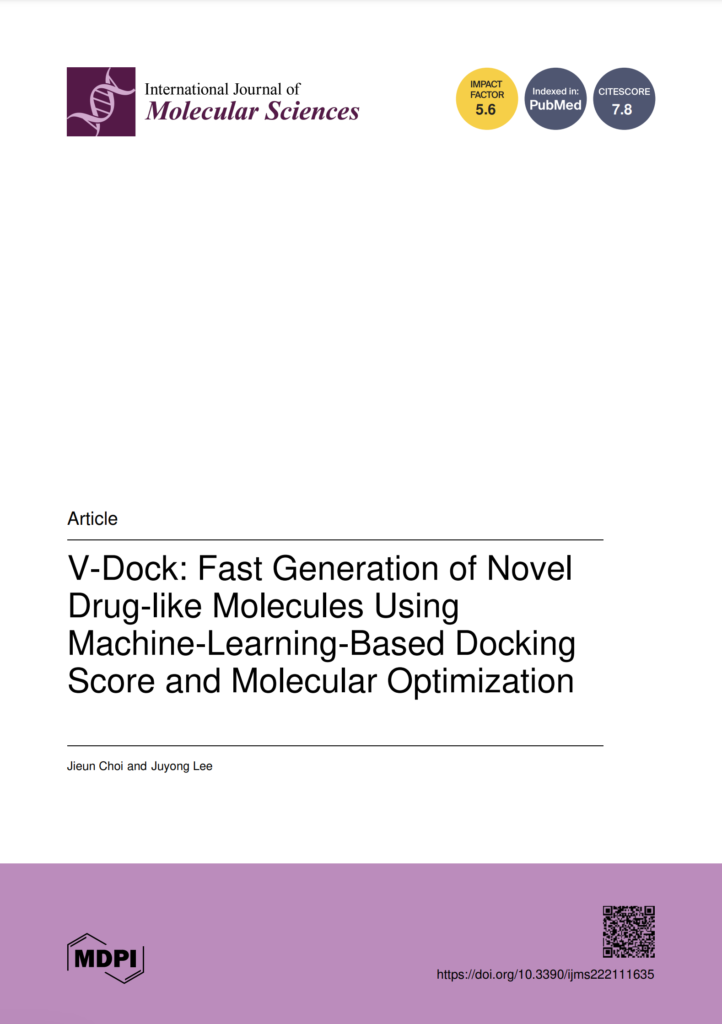
Choi J; Lee J
V-Dock: Fast Generation of Novel Drug-like Molecules Using Machine-Learning-Based Docking Score and Molecular Optimization Journal Article
In: IJMS, vol. 22, no. 21, 2021, ISSN: 1422-0067.
@article{Choi2021,
title = {V-Dock: Fast Generation of Novel Drug-like Molecules Using Machine-Learning-Based Docking Score and Molecular Optimization},
author = {Jieun Choi and Juyong Lee},
doi = {10.3390/ijms222111635},
issn = {1422-0067},
year = {2021},
date = {2021-11-00},
urldate = {2021-11-00},
journal = {IJMS},
volume = {22},
number = {21},
publisher = {MDPI AG},
abstract = {We propose a computational workflow to design novel drug-like molecules by combining the global optimization of molecular properties and protein-ligand docking with machine learning. However, most existing methods depend heavily on experimental data, and many targets do not have sufficient data to train reliable activity prediction models. To overcome this limitation, protein-ligand docking calculations must be performed using the limited data available. Such docking calculations during molecular generation require considerable computational time, preventing extensive exploration of the chemical space. To address this problem, we trained a machine-learning-based model that predicted the docking energy using SMILES to accelerate the molecular generation process. Docking scores could be accurately predicted using only a SMILES string. We combined this docking score prediction model with the global molecular property optimization approach, MolFinder, to find novel molecules exhibiting the desired properties with high values of predicted docking scores. We named this design approach V-dock. Using V-dock, we efficiently generated many novel molecules with high docking scores for a target protein, a similarity to the reference molecule, and desirable drug-like and bespoke properties, such as QED. The predicted docking scores of the generated molecules were verified by correlating them with the actual docking scores.},
keywords = {},
pubstate = {published},
tppubtype = {article}
}
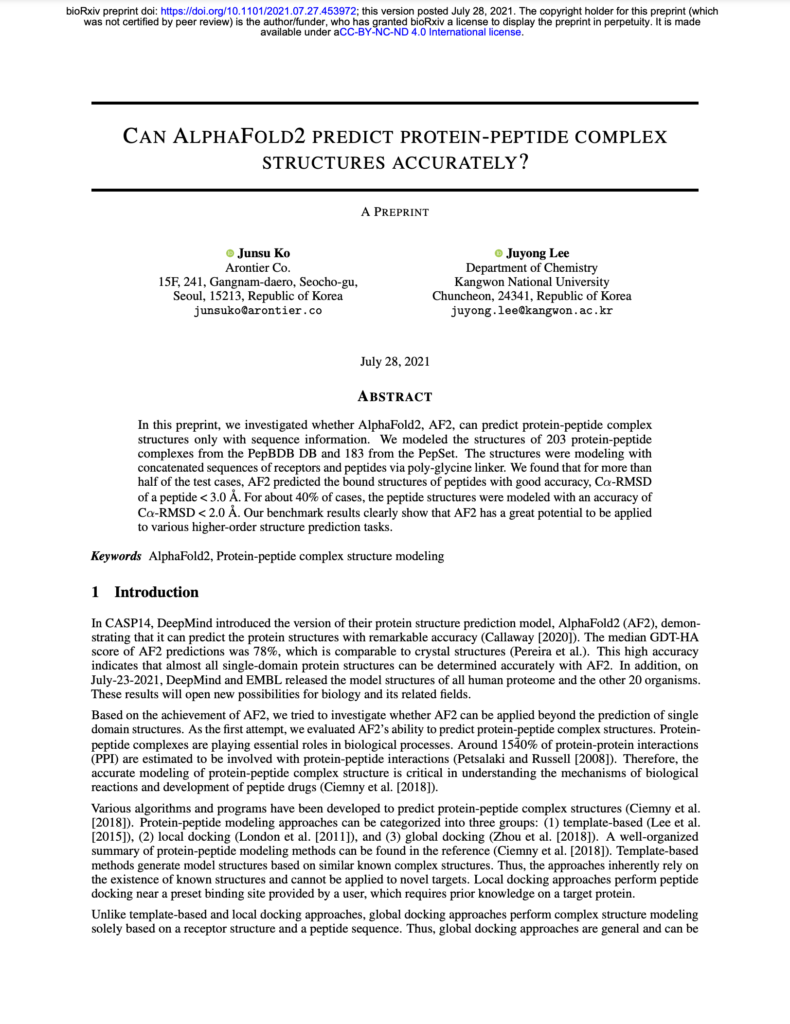
Ko J; Lee J
Can AlphaFold2 predict protein-peptide complex structures accurately? Unpublished
bioRxiv, 2021.
@unpublished{Ko2021,
title = {Can AlphaFold2 predict protein-peptide complex structures accurately?},
author = {Junsu Ko and Juyong Lee},
url = {http://biorxiv.org/lookup/doi/10.1101/2021.07.27.453972},
doi = {10.1101/2021.07.27.453972},
year = {2021},
date = {2021-07-27},
urldate = {2021-07-27},
publisher = {Cold Spring Harbor Laboratory},
abstract = {In this preprint, we investigated whether AlphaFold2, AF2, can predict protein-peptide complex structures only with sequence information. We modeled the structures of 203 protein-peptide complexes from the PepBDB DB and 183 from the PepSet. The structures were modeling with concatenated sequences of receptors and peptides via poly-glycine linker. We found that for more than half of the test cases, AF2 predicted the bound structures of peptides with good accuracy, Cα-RMSD of a peptide < 3.0 Å. For about 40% of cases, the peptide structures were modeled with an accuracy of Cα-RMSD < 2.0 Å. Our benchmark results clearly show that AF2 has a great potential to be applied to various higher-order structure prediction tasks.},
howpublished = {bioRxiv},
keywords = {},
pubstate = {published},
tppubtype = {unpublished}
}
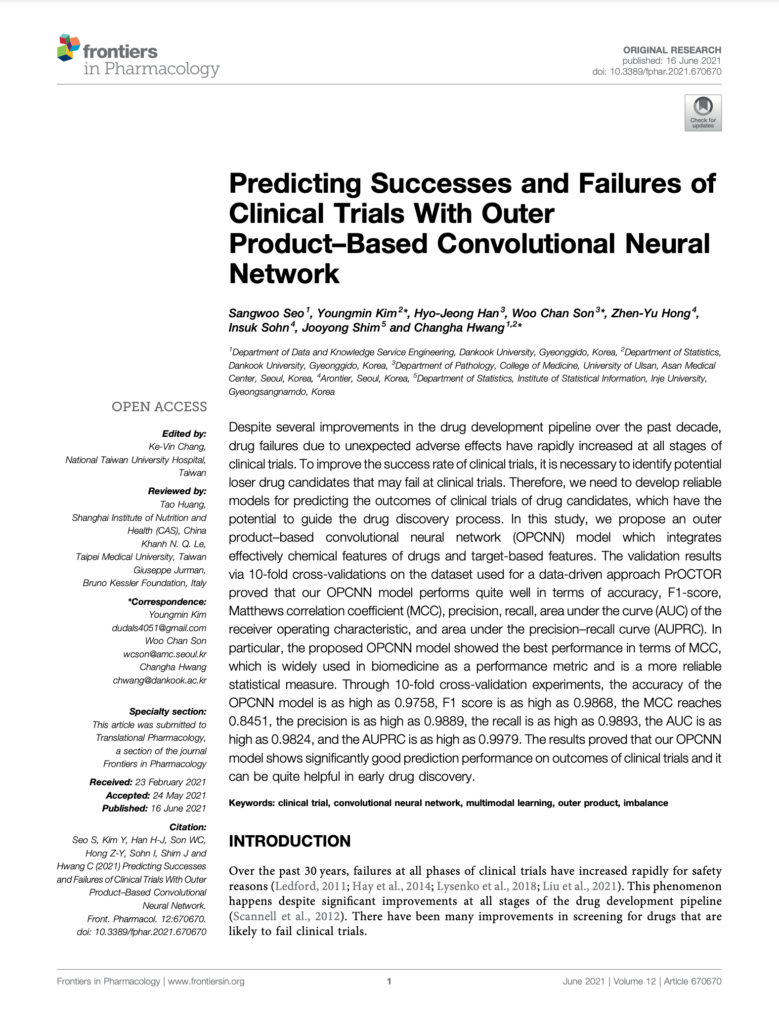
Seo S; Kim Y; Han H; Son W C; Hong Z; Sohn I; Shim J; Hwang C
Predicting Successes and Failures of Clinical Trials With Outer Product–Based Convolutional Neural Network Journal Article
In: Front. Pharmacol., vol. 12, 2021, ISSN: 1663-9812.
@article{Seo2021,
title = {Predicting Successes and Failures of Clinical Trials With Outer Product–Based Convolutional Neural Network},
author = {Sangwoo Seo and Youngmin Kim and Hyo-Jeong Han and Woo Chan Son and Zhen-Yu Hong and Insuk Sohn and Jooyong Shim and Changha Hwang},
doi = {10.3389/fphar.2021.670670},
issn = {1663-9812},
year = {2021},
date = {2021-06-16},
urldate = {2021-06-16},
journal = {Front. Pharmacol.},
volume = {12},
publisher = {Frontiers Media SA},
abstract = {Despite several improvements in the drug development pipeline over the past decade, drug failures due to unexpected adverse effects have rapidly increased at all stages of clinical trials. To improve the success rate of clinical trials, it is necessary to identify potential loser drug candidates that may fail at clinical trials. Therefore, we need to develop reliable models for predicting the outcomes of clinical trials of drug candidates, which have the potential to guide the drug discovery process. In this study, we propose an outer product–based convolutional neural network (OPCNN) model which integrates effectively chemical features of drugs and target-based features. The validation results via 10-fold cross-validations on the dataset used for a data-driven approach PrOCTOR proved that our OPCNN model performs quite well in terms of accuracy, F1-score, Matthews correlation coefficient (MCC), precision, recall, area under the curve (AUC) of the receiver operating characteristic, and area under the precision–recall curve (AUPRC). In particular, the proposed OPCNN model showed the best performance in terms of MCC, which is widely used in biomedicine as a performance metric and is a more reliable statistical measure. Through 10-fold cross-validation experiments, the accuracy of the OPCNN model is as high as 0.9758, F1 score is as high as 0.9868, the MCC reaches 0.8451, the precision is as high as 0.9889, the recall is as high as 0.9893, the AUC is as high as 0.9824, and the AUPRC is as high as 0.9979. The results proved that our OPCNN model shows significantly good prediction performance on outcomes of clinical trials and it can be quite helpful in early drug discovery.},
keywords = {},
pubstate = {published},
tppubtype = {article}
}
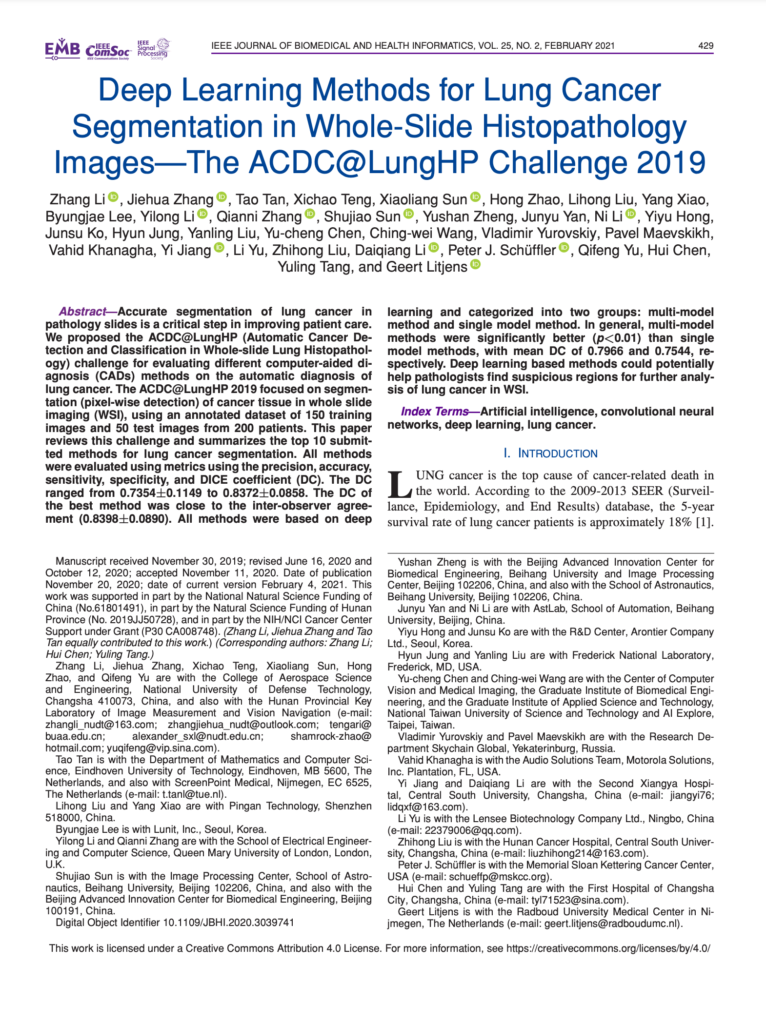
Li Z; Zhang J; Tan T; Teng X; Sun X; Zhao H; Liu L; Xiao Y; Lee B; Li Y; Zhang Q; Sun S; Zheng Y; Yan J; Li N; Hong Y; Ko J; Jung H; Liu Y; Chen Y; Wang C; Yurovskiy V; Maevskikh P; Khanagha V; Jiang Y; Yu L; Liu Z; Li D; Schuffler P J; Yu Q; Chen H; Tang Y; Litjens G
Deep Learning Methods for Lung Cancer Segmentation in Whole-Slide Histopathology Images—The ACDC@LungHP Challenge 2019 Journal Article
In: IEEE J. Biomed. Health Inform., vol. 25, no. 2, pp. 429–440, 2021, ISSN: 2168-2208.
@article{Li2021,
title = {Deep Learning Methods for Lung Cancer Segmentation in Whole-Slide Histopathology Images—The ACDC@LungHP Challenge 2019},
author = {Zhang Li and Jiehua Zhang and Tao Tan and Xichao Teng and Xiaoliang Sun and Hong Zhao and Lihong Liu and Yang Xiao and Byungjae Lee and Yilong Li and Qianni Zhang and Shujiao Sun and Yushan Zheng and Junyu Yan and Ni Li and Yiyu Hong and Junsu Ko and Hyun Jung and Yanling Liu and Yu-cheng Chen and Ching-wei Wang and Vladimir Yurovskiy and Pavel Maevskikh and Vahid Khanagha and Yi Jiang and Li Yu and Zhihong Liu and Daiqiang Li and Peter J. Schuffler and Qifeng Yu and Hui Chen and Yuling Tang and Geert Litjens},
doi = {10.1109/jbhi.2020.3039741},
issn = {2168-2208},
year = {2021},
date = {2021-02-00},
urldate = {2021-02-00},
journal = {IEEE J. Biomed. Health Inform.},
volume = {25},
number = {2},
pages = {429--440},
publisher = {Institute of Electrical and Electronics Engineers (IEEE)},
abstract = {Accurate segmentation of lung cancer in pathology slides is a critical step in improving patient care. We proposed the ACDC@LungHP (Automatic Cancer Detection and Classification in Whole-slide Lung Histopathology) challenge for evaluating different computer-aided diagnosis (CADs) methods on the automatic diagnosis of lung cancer. The ACDC@LungHP 2019 focused on segmentation (pixel-wise detection) of cancer tissue in whole slide imaging (WSI), using an annotated dataset of 150 training images and 50 test images from 200 patients. This paper reviews this challenge and summarizes the top 10 submitted methods for lung cancer segmentation. All methods were evaluated using metrics using the precision, accuracy, sensitivity, specificity, and DICE coefficient (DC). The DC ranged from 0.7354 ± 0.1149 to 0.8372 ± 0.0858. The DC of the best method was close to the inter-observer agreement (0.8398 ± 0.0890). All methods were based on deep learning and categorized into two groups: multi-model method and single model method. In general, multi-model methods were significantly better ( p < 0.01) than single model methods, with mean DC of 0.7966 and 0.7544, respectively. Deep learning based methods could potentially help pathologists find suspicious regions for further analysis of lung cancer in WSI.},
keywords = {},
pubstate = {published},
tppubtype = {article}
}
2020
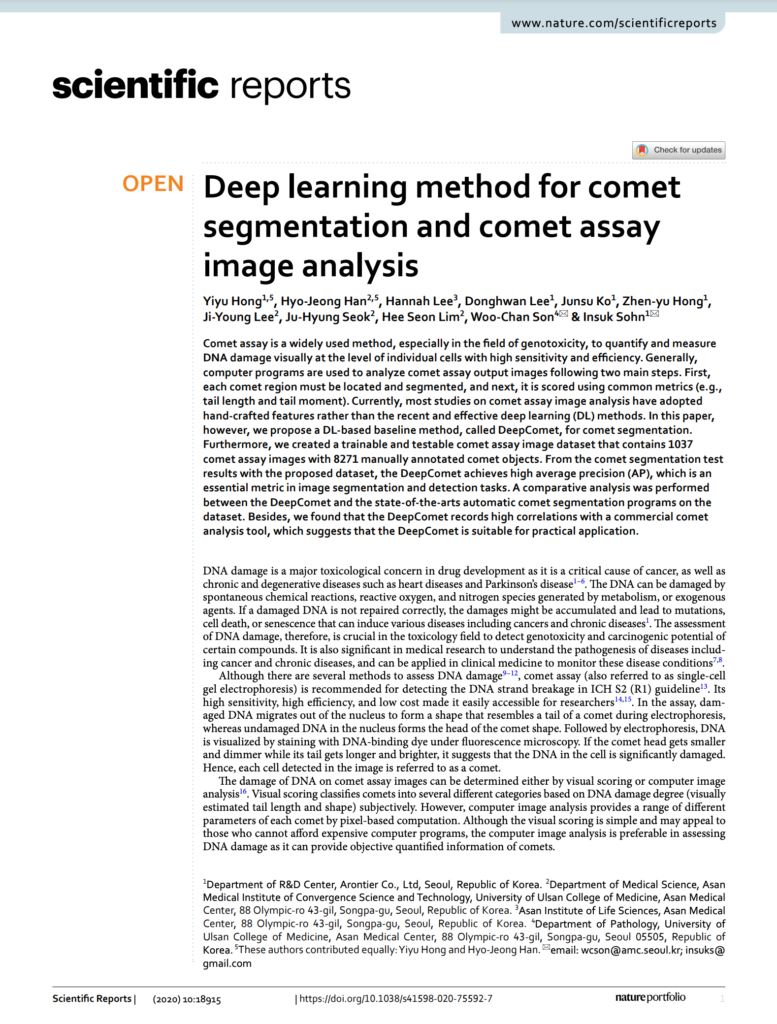
Hong Y; Han H; Lee H; Lee D; Ko J; Hong Z; Lee J; Seok J; Lim H S; Son W; Sohn I
Deep learning method for comet segmentation and comet assay image analysis Journal Article
In: Sci Rep, vol. 10, no. 1, 2020, ISSN: 2045-2322.
@article{Hong2020,
title = {Deep learning method for comet segmentation and comet assay image analysis},
author = {Yiyu Hong and Hyo-Jeong Han and Hannah Lee and Donghwan Lee and Junsu Ko and Zhen-yu Hong and Ji-Young Lee and Ju-Hyung Seok and Hee Seon Lim and Woo-Chan Son and Insuk Sohn},
doi = {10.1038/s41598-020-75592-7},
issn = {2045-2322},
year = {2020},
date = {2020-12-00},
urldate = {2020-12-00},
journal = {Sci Rep},
volume = {10},
number = {1},
publisher = {Springer Science and Business Media LLC},
abstract = {Comet assay is a widely used method, especially in the field of genotoxicity, to quantify and measure DNA damage visually at the level of individual cells with high sensitivity and efficiency. Generally, computer programs are used to analyze comet assay output images following two main steps. First, each comet region must be located and segmented, and next, it is scored using common metrics (e.g., tail length and tail moment). Currently, most studies on comet assay image analysis have adopted hand-crafted features rather than the recent and effective deep learning (DL) methods. In this paper, however, we propose a DL-based baseline method, called DeepComet, for comet segmentation. Furthermore, we created a trainable and testable comet assay image dataset that contains 1037 comet assay images with 8271 manually annotated comet objects. From the comet segmentation test results with the proposed dataset, the DeepComet achieves high average precision (AP), which is an essential metric in image segmentation and detection tasks. A comparative analysis was performed between the DeepComet and the state-of-the-arts automatic comet segmentation programs on the dataset. Besides, we found that the DeepComet records high correlations with a commercial comet analysis tool, which suggests that the DeepComet is suitable for practical application.},
keywords = {},
pubstate = {published},
tppubtype = {article}
}
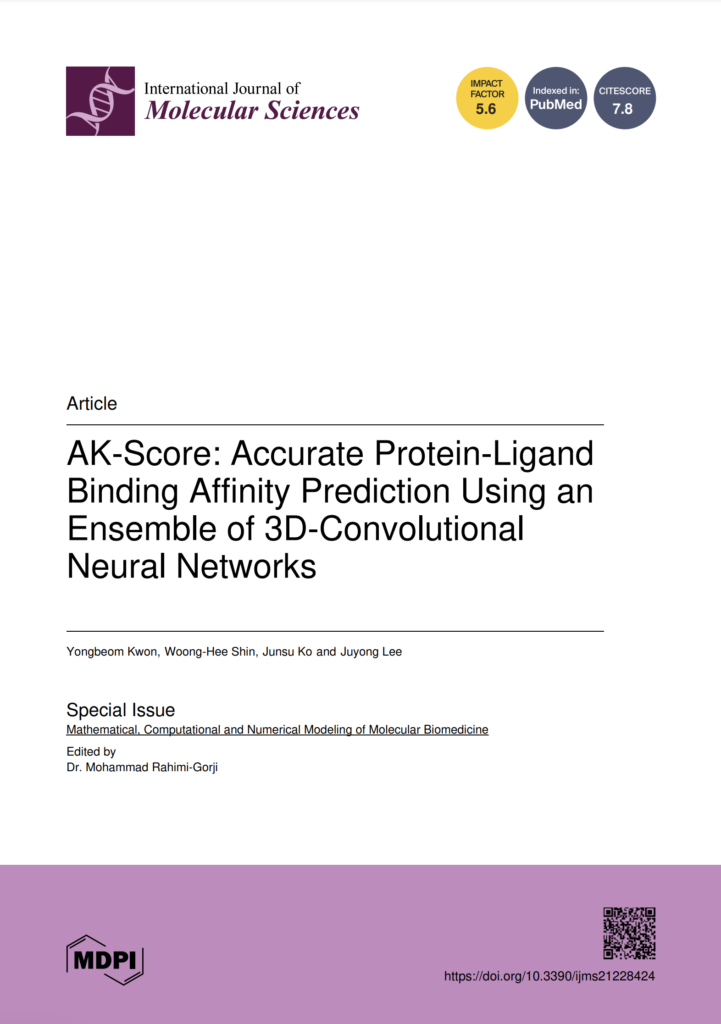
Kwon Y; Shin W; Ko J; Lee J
AK-Score: Accurate Protein-Ligand Binding Affinity Prediction Using an Ensemble of 3D-Convolutional Neural Networks Journal Article
In: IJMS, vol. 21, no. 22, 2020, ISSN: 1422-0067.
@article{Kwon2020,
title = {AK-Score: Accurate Protein-Ligand Binding Affinity Prediction Using an Ensemble of 3D-Convolutional Neural Networks},
author = {Yongbeom Kwon and Woong-Hee Shin and Junsu Ko and Juyong Lee},
doi = {10.3390/ijms21228424},
issn = {1422-0067},
year = {2020},
date = {2020-11-00},
urldate = {2020-11-00},
journal = {IJMS},
volume = {21},
number = {22},
publisher = {MDPI AG},
abstract = {Accurate prediction of the binding affinity of a protein-ligand complex is essential for efficient and successful rational drug design. Therefore, many binding affinity prediction methods have been developed. In recent years, since deep learning technology has become powerful, it is also implemented to predict affinity. In this work, a new neural network model that predicts the binding affinity of a protein-ligand complex structure is developed. Our model predicts the binding affinity of a complex using the ensemble of multiple independently trained networks that consist of multiple channels of 3-D convolutional neural network layers. Our model was trained using the 3772 protein-ligand complexes from the refined set of the PDBbind-2016 database and tested using the core set of 285 complexes. The benchmark results show that the Pearson correlation coefficient between the predicted binding affinities by our model and the experimental data is 0.827, which is higher than the state-of-the-art binding affinity prediction scoring functions. Additionally, our method ranks the relative binding affinities of possible multiple binders of a protein quite accurately, comparable to the other scoring functions. Last, we measured which structural information is critical for predicting binding affinity and found that the complementarity between the protein and ligand is most important.},
keywords = {},
pubstate = {published},
tppubtype = {article}
}

- | AI Research Scientst 채용 공고 | Arontier | 2024-04-17 |
---|---|---|---|
다음 | 2019 인공지능 신약개발 플랫폼 구축사업 신규과제 선정 | Arontier | 2021-06-30 |